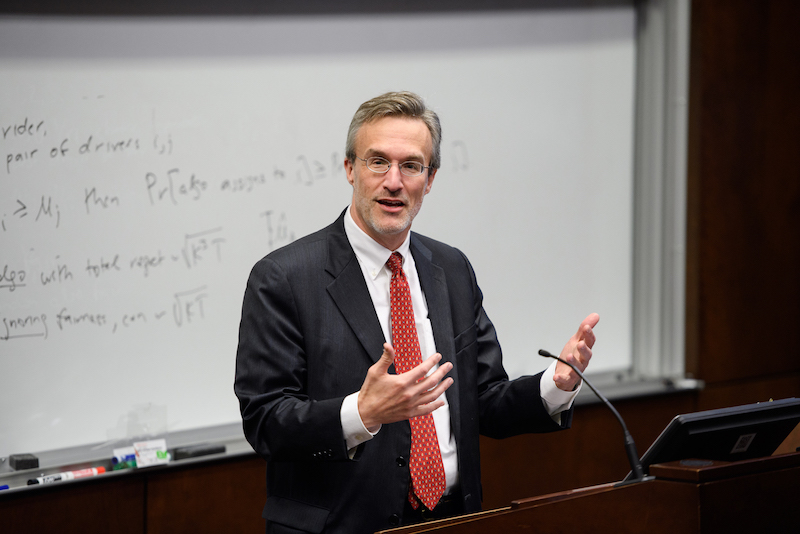
The Optimizing Government Project brings together scholars and researchers to discuss the use of machine learning by government.
In recent years, the private sector has succeeded in finding many ways to leverage machine learning—a type of artificial intelligence that enables computers to “learn and adapt through experience.” Well-known private sector applications of machine learning include Google’s self-driving car project, online recommendations personalized for customers on websites like Amazon and Netflix, and fraud detection by credit card companies.
But as the private sector embraces machine learning in new ways, the application of machine learning by government agencies has only started to take root. The use of artificial intelligence by government, though, raises important questions for a democratic society—about fairness, equality, transparency, and accountability.
Over the past year, the Optimizing Government Project at the University of Pennsylvania explored these questions through a series of interdisciplinary workshops. Workshop speakers included: Aaron Roth and Michael Kearns from Penn’s Department of Computer and Information Science; Tom Baker, Cary Coglianese, Seth Kreimer, and Sandra Mayson from Penn Law; Richard Berk from Penn’s Criminology Department; Nancy Hirschmann from Penn’s Political Science Department; Samuel Freeman from Penn’s Department of Philosophy; and Dennis P. Culhane from Penn’s School of Social Policy & Practice. The workshops also featured Benedict Dellaert of the Netherlands’ Erasmus University Rotterdam, Sorelle Friedler of Haverford College, Stephen Goldsmith of the Harvard Kennedy School, John Mikhail and David Robinson of Georgetown Law, Helen Nissenbaum of Cornell University, and Andrew Selbst of the Yale Information Society Project.
This series in The Regulatory Review distills many of the key insights from the workshops. Video recordings of each workshop and additional information can be found at the website of the Optimizing Government Project. The Project, sponsored by the Fels Policy Research Initiative, was established by Professors Coglianese and Berk and has been directed by Jeremy Sklaroff, a Penn Law and Wharton MBA student. The Project’s purpose is to bring together scholars and researchers from computer science, data analysis, social science, and law to “tackle both current and future challenges related to applying artificial intelligence techniques in governmental and public policy settings.”
The Regulatory Review is pleased to highlight the crucial issues confronting government as it moves into an era of machine-learning.
The Usefulness—and Possible Dangers—of Machine Learning
October 2, 2017 | Eric Schlabs, The Regulatory Review
The first Optimizing Government workshop held at the University of Pennsylvania Law School last year demystified machine learning, explaining its functionality, potential, and limitations, while also considering its potential for unfair outcomes.
Machine Learning’s Implications for Fairness and Justice
October 3, 2017 | Eric Schlabs, The Regulatory Review
Algorithms can help government perform its duties more effectively and accurately. But algorithms might also encode hidden biases that disproportionately and adversely impact minorities. What does fairness demand when government uses machine learning?
Experts Weigh in on Fairness and Performance Trade-Offs in Machine Learning
October 4, 2017 | Leah Wong, The Regulatory Review
Algorithmic fairness can come at a cost to other values. Even optimizing for one conception of fairness can detract from other notions of fairness. Government officials need to be aware of the technical consequences of committing to particular definitions of fairness—and to the trade-offs involved in the use of machine learning.
Regulating the Robots that Help Us Decide
October 5, 2017 | Paul Stephan, The Regulatory Review
Today, newly-developed computer algorithms are starting to recommend individualized health care and retirement plans to consumers. In the future, such “robo advisors” will do even more—recommending mortgages, credit cards, and other financial services to more individuals, all with increasing technical sophistication. How should the regulatory world adapt?
Should Robots Make Law?
October 9, 2017 | Sarah Kramer, The Regulatory Review
Machine learning is often described as a “black box” tool because of its autonomous and inscrutable qualities, in comparison to other types of computational techniques. How, then, does its use by government square with conventional democratic principles of transparency and accountability?
How Can We Reveal Bias in Computer Algorithms?
October 10, 2017 | Katie Cramer, The Regulatory Review
Are there technical ways to make machine learning algorithms more transparent? Is it possible to regulate governmental use of machine-learning systems so that they generate less inscrutable decisions? Strategies exist to make algorithms and their impacts at least more intuitively understandable.
How Machine Learning Can Improve Public Sector Services
October 11, 2017 | Katie Cramer, The Regulatory Review
What practical challenges lie ahead for government officials who seek to incorporate algorithms into their decision-making? The concluding workshop of the Optimizing Government Project offered insights into these challenges as well as ideas for how to overcome them.