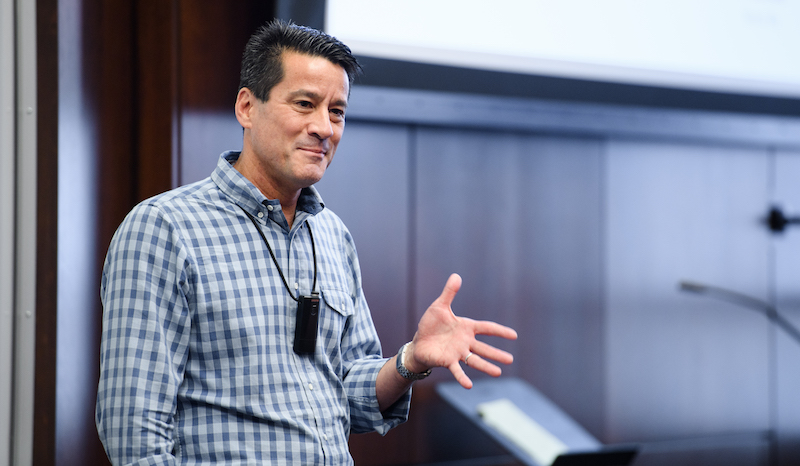
Experts from multiple disciplines discuss notions of fairness within the age of machine learning.
Artificial intelligence plays an increasingly important role in informing public policy—for everything from the likelihood of an individual becoming homeless or dropping out of school can be predicted through algorithms today. But how do these algorithms generate their predictions? Are the results fair? How do we define “fair” when we balance technology and government decisions?
Top researchers recently explored these questions at a workshop hosted the University of Pennsylvania Law School, “Fairness and Performance Trade-Offs in Machine Learning.” The event was part of a larger project dedicated to exploring policy and technical challenges as machine learning models continue to be adopted by governments in a range of contexts to “optimize” government. The researchers explored whether these kinds of algorithmic decisions are consistent with multi-disciplinary conceptions of fairness.
Michael Kearns, a computer science professor at Penn and founding director of both the Warren Center for Network and Data Sciences and the Penn Program in Networked and Social Systems Engineering, presented a case study examining the technical consequences of committing to a particular definition of fairness in the context machine learning. Relying on a computer scientist’s notion of individual fairness—that it is unfair to preferentially choose one individual for a loan, a job, or admission to college over another if the individual is not as qualified as the other individual—Kearns showed that the issue of fairness is not always as simple as it might seem.
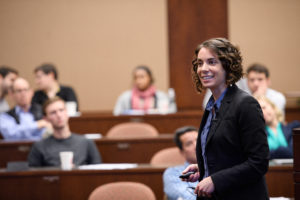
University of Georgia School of Law Professor Sandra Mayson discusses machine bias during her lecture.
Machine learning, according to Kearns, may ultimately end up leading to unfair “exploitation” and “exploration” trade-offs. Kearns used the Uber model as an example. For instance, even if one continued to select the best driver based on the objective rating average of each driver, one still might not have any idea who is actually the “best” driver; any indication of the “best” driver may change as the number of total Uber rides increases.
The temptation to maximize profits and give rides to the better driver based solely on the numbered ratings—what is called “exploitation”—impedes learning about the different factors that could have had an effect on the ratings—“exploration.” These different factors may include the unconscious biases of passengers towards drivers of different races, preferences for certain vehicles, and the time of the day drivers typically work. Therefore, although a machine-learning algorithm could be objectively fair by the numbers, it could be “unfair” by societal standards.
Kearns argued that it is still possible to design the algorithm to include notions of fairness, but it might be harder to accomplish this outcome. The algorithm could be designed to add context in order to correct for biases when searching for the expected reward—the most optimal outcome.
Kearns concluded that, even though the achievement of fairness is consistent with implementing the optimal policy, it is not necessarily consistent with actually learning the optimal policy. Because the feasibility of learning an optimal policy depends on unknown factors, these algorithms may take more time and may ultimately have technical efficiency trade-offs.
Providing an interdisciplinary outlook on the effects of machine learning, Sandra Mayson, an assistant professor of law at the University of Georgia School of Law and formerly a research fellow at Penn Law’s Quattrone Center for the Fair Administration of Justice, situated the fairness concerns surrounding machine learning in a broader moral and legal framework. She started off with the classic case of machine bias, where two different companies, ProPublica and Northpointe, conducted their own analyses on the fairness of COMPAS, an algorithm created by Northpointe and used nationwide to guide sentencing, parole, and bail determinations in the criminal justice system. ProPublica is an investigative journalism newsroom.
Both companies came back with different interpretations of the same empirical results, emphasizing different measures of fairness in their interpretation. ProPublica claimed that the COMPAS risk scores were plagued by racial bias, systematically giving black persons a higher likelihood of criminal reoffense than white persons. Northpointe countered that the algorithm mechanically assigned any group with existing higher recidivism rates with higher risk scores. Mayson said these results highlighted that “several possible metrics of fairness exist in any kind of predictive situation.”
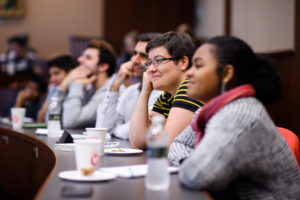
Attendees listen to the panelists discuss the equity of machine learning in government.
Mayson also drew on anti-discrimination law to illustrate two dominant legal conceptions of fairness: disparate treatment and disparate impact. She explained that disparate treatment is formal or intentionally differential treatment of “similarly situated” people. She provided examples of laws that regulated and prohibited disparate treatment on the basis of different characteristics, such as the U.S. Constitution’s Equal Protection Clause and Title VII of the Civil Rights Act.
In contrast, the disparate-impact theory of discrimination concerns practices that, while facially neutral, cause a disproportionate, adverse impact on specific members of a protected class under Title VII, such as women or racial minorities. In other words, these practices are, as Mayson explained, “fair in form, but discriminatory in operation.” Accordingly, efforts to combat disparate-impact discrimination are predicated on the belief that government should not engage in practices that reinforce exclusion of certain groups, Mayson noted.
The distinction between disparate treatment and disparate impact has given rise to what Mayson described as a tension in the context of machine learning. She explained that algorithms that aim to eliminate disparate treatment might end up having a disparate impact on people based on their group membership.
Kearn’s technological definition of fairness may, to a certain extent, be responsive to disparate treatment. Kearns’s metric would eliminate disparate treatment on the basis of a non-merit-relevant factor, but not necessarily eliminate disparate treatment on the basis of other sensitive traits: race, sex, national origin, and religion. Mayson noted that whether the algorithm did treat people differently on the basis of such traits would ultimately depend on whether these traits have any independent predictive outcome on the desired result.
Concerning disparate impact, Mayson believed Kearns’s fairness metric could avoid some forms of disparate impact if algorithms were programed to give equally qualified people in separate sub-groups an equal chance to be the predicted optimal choice.
Ultimately Mayson and Kearns expressed hope that this was only the beginning of a cross-disciplinary conversation on fairness and performance trade-offs in machine learning.
The rest of the Optimizing Government Project fall workshops can be viewed at the project’s website. The project was supported by the Fels Policy Research Initiative at the University of Pennsylvania.
This essay is part of a seven-part series, entitled Optimizing Government.