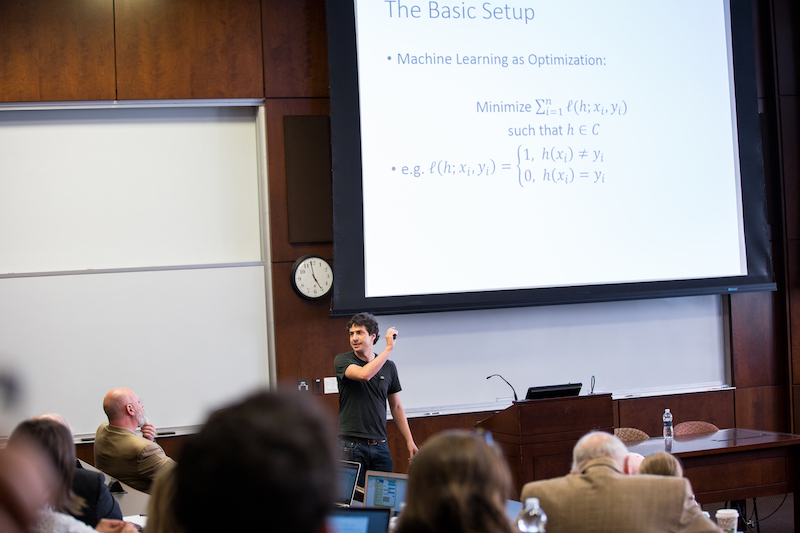
University of Pennsylvania workshop addresses potential biases in the predictive technique.
Stephen Hawking once warned that advances in artificial intelligence might eventually “spell the end of the human race.” And yet decision-makers from financial corporations to government agencies have begun to embrace machine learning’s enhanced power to predict—a power that commentators say “will transform how we live, work, and think.”
During the first of a series of seven Optimizing Government workshops held at the University of Pennsylvania Law School last year, Aaron Roth, Associate Professor of Computer and Information Science at the University of Pennsylvania, demystified machine learning, breaking down its functionality, its possibilities and limitations, and its potential for unfair outcomes.
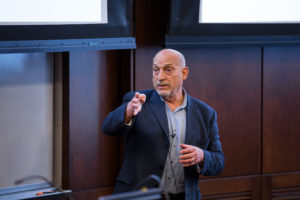
Chairman of the Penn Department of Criminology Richard Berk offers commentary.
Machine learning, in short, enables users to predict outcomes using past data sets, Roth said. These data-driven algorithms are beginning to take on formerly human-performed tasks, like deciding whom to hire, determining whether an applicant should receive a loan, and identifying potential criminal activity.
In large part, machine learning does not differ from statistics, said Roth. But unlike statistics, which aims to create models for past data, machine learning requires accurate predictions on new examples.
This eye toward the future requires simplicity. Given a set of past, or “training,” data, a decision-maker can always create a complex rule that predicts a label—say, likelihood of paying back a loan—given a set of features, like education and employment. But a lender does not seek to predict whether a past loan applicant included in a dataset actually paid back a loan given her education and employment, but instead whether a new applicant will likely pay back a loan, explained Roth.
A simple rule might not be perfect, but it will provide more accuracy in the long run, said Roth, because it will more effectively generalize a narrow set of data to the population at large. Roth noted that for more complex rules, algorithms must use bigger data sets to combat generalization errors.
Because machine-learning algorithms work to optimize decision-making, using code and data sets that can be held up to public scrutiny, decision-makers might think machine learning is unbiased. But discrimination can arise in several non-obvious ways, argued Roth.
First, data can encode existing biases. For example, an algorithm that uses training data to predict whether someone will commit a crime should know whether the people represented in the data set actually committed crimes. But that information is not available—rather, an observer can know only whether the people were arrested, and police propensity to arrest certain groups of people might well create bias.
Second, an algorithm created using insufficient amounts of training data can cause a so-called feedback loop that creates unfair results, even if the creator did not mean to encode bias. Roth explained that a lender can observe whether a loan was paid back only if it was granted in the first place. If training data incorrectly show that a group with a certain feature is less likely to pay back a loan, because the lender did not collect enough data, then the lender might continue to deny those people loans to maximize earnings. The lender would never know that the group is actually credit-worthy, because the lender would never be able to observe the rejected group’s loan repayment behavior.
Third, different populations might have different characteristics that require separate models. To demonstrate his point, Roth laid out a scenario where SAT scores reliably indicate whether a person will repay a loan, but a wealthy population employs SAT tutors, while a poor population does not. If the wealthy population then has uniformly higher SAT scores, without being on the whole more loan-worthy than the poor population, then the two populations would need separate rules. A broad rule would preclude otherwise worthy members of the poor population from receiving loans. The result of separate rules is both greater fairness and increased accuracy—but if the law precludes algorithms from considering race, for example, and the disparity is racial, then the rule would disadvantage the non-tutored minority.
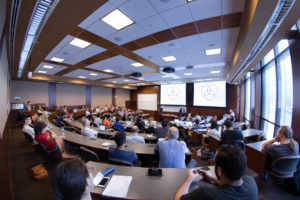
Students, faculty, and other attendees listen as panelists present background on machine learning.
Finally, by definition, fewer data exist about groups that are underrepresented in the data set. Thus, even though separate rules can benefit underrepresented populations, such rules create new problems, argued Roth. Because the training data used by machine learning will include fewer points, generalization error can be higher than it is for more common groups, and the algorithm can misclassify underrepresented populations with greater frequency—or in the loan context, deny qualified applicants and approve unqualified applicants at a higher rate.
Roth’s presentation was followed by commentary offered by Richard Berk, the Chair of the Department of Criminology. Berk explained that algorithms are unconstrained by design, which optimizes accuracy, but argued that the lack of constraint might be what gives some critics of artificial intelligence some pause. When decision-makers cede control of algorithms, they lose the ability to control the assembly of information, and algorithms might invent variables from components that alone have, for example, no racial content, but when put together, do.
Berk stated that mitigating fairness concerns often comes at the expense of accuracy, leaving policymakers with a dilemma. Before an algorithm can even be designed, a human must make a decision as to how much accuracy should be sacrificed in the name of fairness.
Roth stated that this tradeoff causes squeamishness among policymakers—not because such tradeoffs are new, but because machine learning is often more quantitative, and therefore makes tradeoffs more visible than with human decision-making. A judge, for example, might make an opaque tradeoff by handing down more guilty verdicts, thereby convicting more guilty people at the expense of punishing the innocent. But that tradeoff is not currently measurable. Both Roth and Berk expressed hope that machine learning’s effect of forcing more open conversations about these tradeoffs will lead to better, more consistent decisions.
Penn Law Professor Cary Coglianese, director of the Penn Program on Regulation, introduced and moderated the workshop. Support for the series came from the Fels Policy Research Initiative at the University of Pennsylvania.
This essay is part of a seven-part series, entitled Optimizing Government.