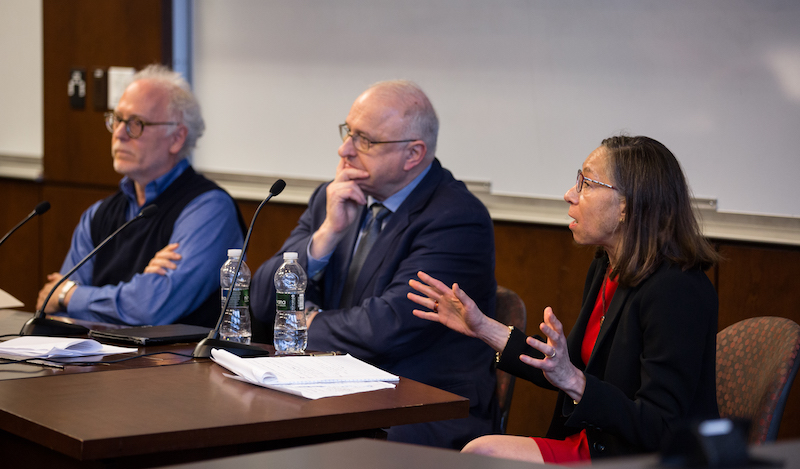
Penn professors grapple with balancing efficiency and equality of government algorithms.
Nowadays, government is armed with algorithms that can forecast domestic violence and employee effectiveness, allowing it to perform its duties more effectively and to achieve correct results more often. But these algorithms can encode hidden biases that disproportionately and adversely impact minorities. What, then, should government consider when implementing predictive algorithms? Where should it draw the line between effectiveness and equality?
Panelists speaking at the University of Pennsylvania Law School grappled with these questions during the second of four workshops that are part of a larger Optimizing Government Project that seeks to inform the use of machine learning in government. The panel, moderated by Penn Law Professor Cary Coglianese, sought to conceptualize fairness and equality and to distill their philosophical and legal implications for machine learning.
Nancy Hirschmann, a political science professor at Penn and director of the Program on Gender, Sexuality, and Women’s Studies, began the panel by observing that the Ancient Greek idea that likes should be treated alike and differences should be treated differently is too simple for today’s society of multiple and complex identities and abilities. Instead, she said, equality must apply to everyone in accordance with their specific differences.
But attention to difference can create difficulties for equality: although women ought to be considered equals of men, a complete failure to consider differences might mean, for example, that women cannot receive pregnancy leave—because men cannot become pregnant. Or if they do receive it, and their difference is acknowledged, then they are penalized. Treating people equally while respecting their differences has been a difficulty struggle. So, Hirschmann argued, when machine-learning algorithms take differences into account, they should be designed to avoid over-generalizations of minority groups and instead identify nuanced patterns through empirical research.
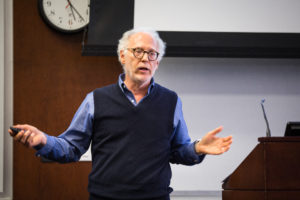
Penn Law’s Professor Seth Kreimer discusses machine learning at the workshop.
Hirschmann then went on to discuss the role of implicit bias in machine learning. Although algorithms can avoid explicit bias against women and minorities, she noted that implicit bias is a persistent problem in both algorithms themselves and in data interpretation, as algorithm creators cannot avoid biases that they do not know they have. Hirschmann urged computer scientists to continually account for biases and to engage with ethnographers and anthropologists when creating frameworks.
Next, Samuel Freeman, the Avalon Professor of the Humanities at Penn, parsed the differences between justice, which involves applying and assessing the law and fundamental rights, and fairness, which plays a role in assessing laws but is mostly about equality.
Freeman listed three relevant forms of justice: formal justice, which requires like cases be treated alike; substantive justice, which requires respect for people as moral and social equals; and procedural justice, which requires that decisions be made in a way that protects individuals’ liberty and maximizes correct outcomes by, for example, excluding inadmissible evidence at trial.
Machine learning, he said, is focused on improving procedural justice by ensuring that outcomes in government procedures are more often correct.
But fairness and substantive justice make it difficult to answer abstract questions about machine learning’s procedural justice, argued Freeman, because machine learning can create unfairness in the form of implicit discrimination against minorities. Freeman left open the question of whether there can be a permissible tradeoff between fairness and equal treatment, on one hand, and efficiency and truth, on the other, or whether fairness and equal treatment must always take priority.
Penn Law Professor Seth Kreimer concluded the event by discussing the concept of equal protection, which the Supreme Court has characterized as “essentially a direction that all persons similarly situated should be treated alike.” But when are people “similarly situated”?
Kreimer stated that distinctions between groups of people are legitimate when they are “rationally related” to a “legitimate government interest.” But despite the ease with which this low standard allows government to treat groups differently, there are three elements of equal treatment that might render government use of machine learning problematic, or even unconstitutional.
First, equal treatment serves as a rejection of arbitrary exercises of government power. This element, Kreimer said, is satisfied by machine learning, which uses non-arbitrary algorithms. Next, equal protection requires justifications of distinctions to the general public. Because “the algorithm said so” is a vague justification that does not enable citizens to effectively respond, machine learning is constitutionally problematic. Finally, Kreimer pointed to the late Justice Scalia’s admonition that equal protection “requires the democratic majority to accept for themselves and their loved ones what they impose on you and me.” Because machine learning might make distinctions that uniquely harm minorities, the “democratic majority” would no longer be forced to live with the results it imposes on others, thereby violating Justice Scalia’s equal protection principle.
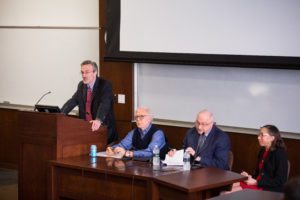
Penn Law’s Professor Cary Coglianese moderates discussion among the panelists.
Kreimer then shifted the discussion to machine learning’s impacts on racial minorities. Because of our society’s moral conceptions of race and concerns about history, equal protection doctrine requires courts to inspect racial distinctions more carefully.
Machine learning can easily be programmed to exclude race, but it might inadvertently substitute other characteristics that correlate with race. When such variables were created by historical racial hostility—like involvement in the criminal justice system—or when they reinforce cycles of exclusion, they might be unconstitutional, Kreimer argued.
Such variables might also violate statutes, including Title VII of the Civil Rights Act of 1964, which prevents “not only overt discrimination but also practices that are fair in form, but discriminatory in operation” in hiring and firing. Although an employer may defend against differential treatment if it is “consistent with business necessity,” the differently treated employee may show that the employer could have adopted an alternative distinction with less disparate impact that served the employer’s legitimate needs. Therefore, even if machine learning’s predictive capacities are necessary for business, machine learning’s transparency puts its alternatives on full display—the fired employee would have access to the algorithm’s code, its decision-making path. This opens the door to lawsuits, said Kreimer.
As to what level of decreased productivity serves an employer’s legitimate needs, like so many other questions about government use of machine learning, there is no clear answer.
This event and the rest of the Optimizing Government workshops can be viewed here. The Optimizing Government project was supported by the Fels Policy Research Initiative at the University of Pennsylvania.
This essay is part of a seven-part series, entitled Optimizing Government.