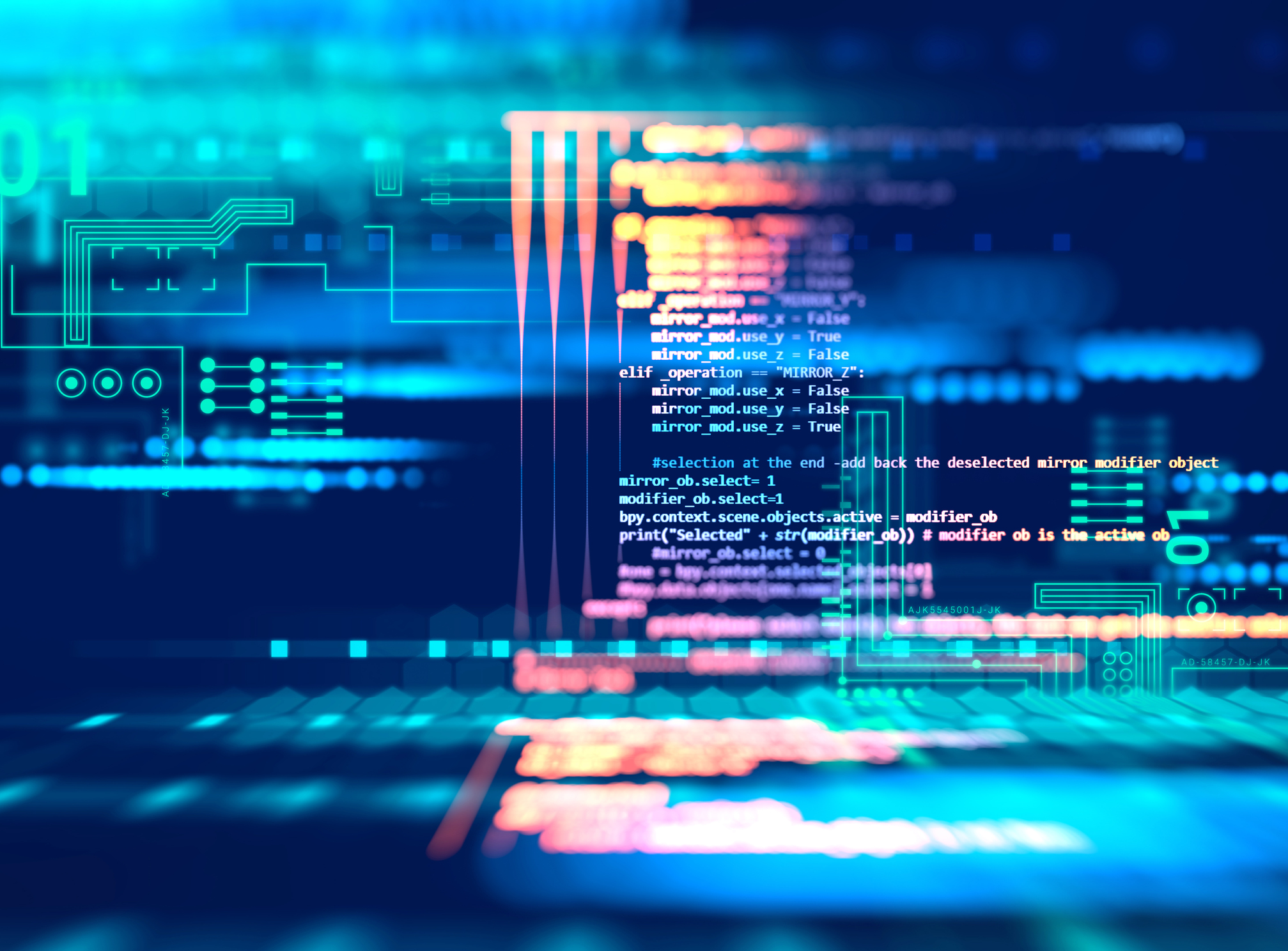
Rather than raising alarm bells, government uses of artificial intelligence fit well within existing legal frameworks.
Sophisticated computational techniques, known as machine-learning algorithms, increasingly underpin advances in business practices, from investment banking to product marketing and self-driving cars. Machine learning—the foundation of artificial intelligence—portends vast changes to the private sector, as many job functions performed by humans will increasingly be performed by these digital robots. But job displacement by machine learning will not be limited to the private sector. Governments may soon undergo their own data revolution and find ways to use machine learning to support smarter public sector decision-making by administrative agencies—potentially even replacing certain human decisions.
This governmental revolution will likely take the form of what we call adjudicating by algorithm and regulating by robot. In the former, algorithms could become the arbiters of determinations about individuals, such as in matters involving claims for government benefits or the granting of licenses. In the latter, algorithms could, in certain cases, choose from among many possible rules the one that an agency embeds in law. When applied both to adjudication and rulemaking, algorithms promise powerful increases in speed and accuracy in decision-making, perhaps also eliminating the biases that can permeate human judgment. Furthermore, using machine-learning algorithms to automate rulemaking and enforcement might prove especially useful, even essential, for overseeing automated private-sector activity, such as high-speed securities trading.
Despite these advantages, the specter of rule by robots has already begun to raise alarm. Algorithmic adjudication and robotic rulemaking imply a loss of autonomy and control over self-government. Would such practices even be legal? After all, the U.S. legal system assumes a government “of the people, by the people, and for the people”—not government by the robots.
At first glance, an automated government would seem antithetical to principles embodied in U.S. constitutional and administrative law, including those involving limits on the delegation of governmental authority, requirements for due process, proscriptions on discrimination, and demands for transparency. Yet, on closer inspection, and with a proper understanding of how machine learning operates, governmental reliance on algorithms need not be feared, and existing legal doctrines need not create insuperable barriers to governmental use of machine learning.
Critical to algorithms’ compatibility with current law are their mathematical properties. Consider, for example, the nondelegation doctrine. Under this doctrine, government cannot delegate lawmaking authority to private entities, a prohibition that presumably would also limit delegations to machines. Although this doctrine firmly curtails the delegating of lawmaking power outside of government—with the U.S. Supreme Court even characterizing such delegations as “obnoxious”—the way that machine learning functions should make algorithmic delegations unproblematic. For one, algorithms do not suffer the key dangers of self-interested and self-generated biases that make delegations to private individuals so obnoxious. For another, the math underlying machine learning necessitates that government officials program algorithms with clear goals, or objective functions, which will certainly satisfy the intelligible principle test that courts use when applying the nondelegation doctrine.
Turning to due process, the longstanding test found in the Supreme Court’s decision in Mathews v. Eldridge requires a balancing of a decision method’s error rates, the private interests at stake, and demands on government resources. The private interests at stake are exogenous to—that is, unaffected by—the decision method used. But since machine learning can both reduce error rates and economize government resources, algorithms should fare well under traditional due process standards.
In terms of anti-discrimination, federal agencies could maximize the accuracy of their decisions and even reduce the disparate impacts of their adjudicatory algorithms by including variables representing individuals’ memberships in protected classes. Yet, even such explicit consideration of class variables will still likely be permissible under existing Fifth Amendment equal protection doctrine that applies to federal agencies —due to the unique ways in which machine learning considers those variables. Additionally, the “black box” nature of machine learning will often preclude any inference of discriminatory intent, absent some separate showing of manifest animus on the part of the government officials.
Finally, it might seem that machine learning’s black-box properties come into tension with the principles of transparency that undergird much of U.S. administrative law. Agencies must give adequate reasons for rulemakings and provide transparent information about their decisions. Yet, machine learning does not afford the ability to offer causal claims in support of decisions; it is not possible to use it to justify a government decision to regulate X in a certain way because doing so causes some reduction in a harm, Y. That said, causation is not the keystone of transparency. It will suffice under prevailing doctrine for officials to justify so regulating X because it optimizes a well-constructed, policy-relevant objective function, one that results in a reduction in Y even if the algorithm being used cannot also support a strict, statistical inference that regulating X causes a reduction in Y. Furthermore, although some algorithmic specifications may be exempt from disclosure under the Freedom of Information Act, the basic terms of an algorithm’s objective function will always remain disclosable, satisfying conventional standards for reason-giving and disclosure.
In sum, we need not be terrified by the prospect of adjudicating by algorithm or rulemaking by robot. Although the introduction of artificial intelligence in government may conjure images of computerized overlords, machine learning is just like any other machine: useful when deployed appropriately by responsible human officials. For the same reasons as government has long relied on physical machines to support decision-making, such as with weights and measurements, modern digital machines can be readily incorporated into governmental practice under prevailing law.
This is not to say, of course, that machine learning cannot be misused or abused. Any tool can be. Indeed, although machine learning passes legal muster, government officials should consider broader policy concerns that animate existing legal doctrines when deciding whether and how to use algorithms. They should also strive to maintain avenues for government officials to engage empathically with the public. But when used sensibly, machine learning promises important benefits in terms of improving accuracy, reducing untoward human biases, and enhancing governmental efficiency. Algorithmic adjudication and robotic rulemaking offer the public sector many of the same decision-making advantages that machine learning increasingly delivers in the private sector.
This essay originally appeared in the Oxford Business Law Blog. It summarizes the authors’ more detailed analysis in Cary Coglianese and David Lehr, Regulating by Robot: Administrative Decision-Making in the Machine-Learning Era, 105 Geo. L.J. 1147 (2017).