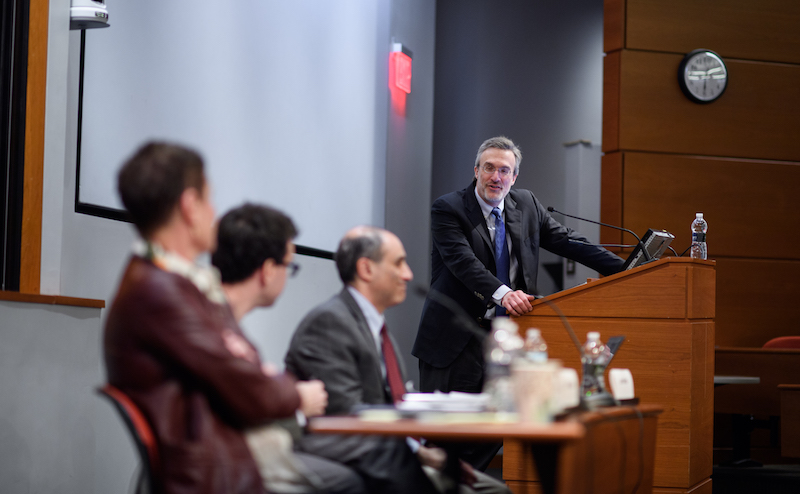
Workshop evaluated benefits and challenges of delegating government decision-making to computers.
A law-making machine that runs the government may seem like an idea straight out of a Star Trek episode, but computers already play key roles in all facets of society—including in governmental decision-making. Increasingly, modern machine learning algorithms—sometimes called digital robots—deliver improved accuracy and efficiency in private-sector settings. Does this type of computer technology fit well with democratic principles when used by governments? Is society ready for robots that make law?
A recent workshop held at the University of Pennsylvania Law School examined these questions posed by the interaction of democratic governance and technology. The workshop was part of the Optimizing Government Project, which seeks to foster interdisciplinary collaboration on research related to machine learning and government. Cary Coglianese—a professor at the University of Pennsylvania Law School, director of the Penn Program on Regulation, and a leader of the Optimizing Government Project—moderated the discussion.
One concern centered on the knowledge of government officials who would rely on or oversee machine learning algorithms. These algorithms recognize patterns in data and can support automated decision-making based on the patterns they “learn.” Government officials, however, may not know what factors the computer algorithm has incorporated into its pattern recognition and decision-making process, noted Helen Nissenbaum at the workshop. Nissenbaum—a Professor of Information Science at Cornell Tech—argued that algorithms’ “inscrutability” could compromise government transparency.
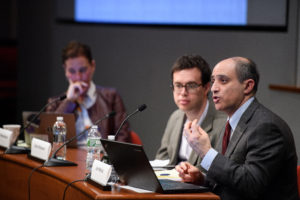
Professor John Mikhail fields questions from attendees at the workshop.
But, as John Mikhail observed at the workshop, human decision-making can be just as complex and inscrutable. Mikhail, a professor at Georgetown University Law Center, emphasized that “information-processing devices,” which include human brains and computers, all function similarly when making decisions, at least at a certain level of abstraction.
Both brains and computers rely on a “computational theory,” including “rules” for mapping inputs to outputs. Human computational theories can often be difficult to fathom, Mikhail argued, just like complex computer algorithms.
Mikhail used the famous “trolley problem” to illustrate this point. In the classic version of this ethics problem, a runaway train barrels towards five people tied to the track. Pull a lever, and the train continues on a different track, but hits one bystander. Another variation involves pushing someone into the train’s path to stop it from killing the five instead of diverting the train towards a bystander. People often say they would pull the lever in the first scenario, but not push the person in the second one, even though the result would be the same.
Why? Mikhail suggested this seemingly inconsistent result arises from unconscious moral algorithms, which are inscrutable and complex, much like machine learning algorithms. Thus, although some differences remain, he advised against drawing too sharp a distinction between humans and machines when evaluating the potential for government to rely on artificial intelligence.
Furthermore, a lack of full transparency does not necessarily prevent citizens from determining whether a decision-making process has been fair. David Robinson, principal and co-founder of the technology law and policy consulting firm Upturn, contended that whether automated decision-making processes worked correctly could be evaluated even without knowing the precise formula or having access to all the data used. He noted that, even in bureaucratic processes where the government cannot be fully transparent, such as in the national security or tax enforcement contexts, policymakers still can prove to the public that the same rule was applied consistently and thus fairly.
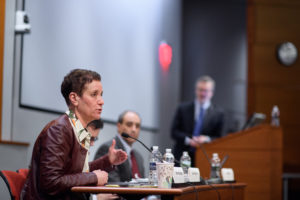
Professor Helen Nissenbaum discusses concerns about the use of algorithms in decision-making by government.
The use of machine learning algorithms, however, may still raise other concerns. Robinson pointed out that historical data patterns discriminate against minorities that were not being fairly treated at the time the data was collected. As a result, programs relying on that data could duplicate this discrimination. Automated decision-making also implicates privacy interests, Nissenbaum observed, since machine learning algorithms require large amounts of data to make accurate predictions.
Technical limitations create additional problems. According to Nissenbaum, employing new technologies may cause the loss of functional integrity, defined as distortions of established decision-making practice caused by incorporating new technology into decision-making processes. For example, users may alter how they think about information to conform to categories in data entry frameworks provided by software programs.
Further, using machine learning algorithms creates novel legal issues. Mikhail mentioned that Congress already delegates power to agencies to make rules, and speculated that courts could view further delegations of decision-making power to machines as beyond what Congress intended.
Artificial intelligence may also fail to mimic the flexibility inherent in existing legal processes. Robinson noted that programming applies very specific rules across all situations, while the law often applies more flexible or ambiguous rules on a case-by-case basis. Thus, computer decision-making leads to more rigid, and possibly less just, results.
So what happens when a computer decides unjustly? When humans make bad decisions, other people can hold them accountable through the democratic process. When a computer makes a decision, can the public hold the computer accountable in the same way? As Nissenbaum asked, who is responsible when “it’s the computer’s fault”?
The “cultural prestige of numbers” could make answering this question more difficult. Non-engineers—including lawyers and policymakers—often feel incapable of questioning the performance or design of high-tech systems, making it more likely for errors or injustices to persist, noted Robinson.
Even the courts might not be able to remedy unjust decisions. A person bringing a discrimination lawsuit under the Equal Protection Clause of the Fourteenth Amendment to the U.S. Constitution, for example, must prove that there was a discriminatory intent or unlawful purpose behind the rule being challenged. But how can those plaintiffs prove such intent when a computer program applying unknown variables made the decision?
Nissenbaum suggested that these issues related to transparency and accountability may erode responsibility for government decision-making. Machine learning algorithms may be efficient tools, but they should be used carefully and thoughtfully.
Mikhail hoped that, over time, a better understanding of both human and machine decision-making processes could eliminate or minimize transparency issues. He also suggested that increased use of automated decision-making could lead to legal changes. In particular, he speculated that courts might begin to accept claims based solely on the discriminatory impact of a rule, rather than continuing to require, for federal officials, a showing of discriminatory intent.
The workshop, which was part of a series supported by the Fels Policy Research Initiative, can be viewed in its entirety via the video-recording available on the Optimizing Government Project’s website.
This essay is part of a seven-part series, entitled Optimizing Government.