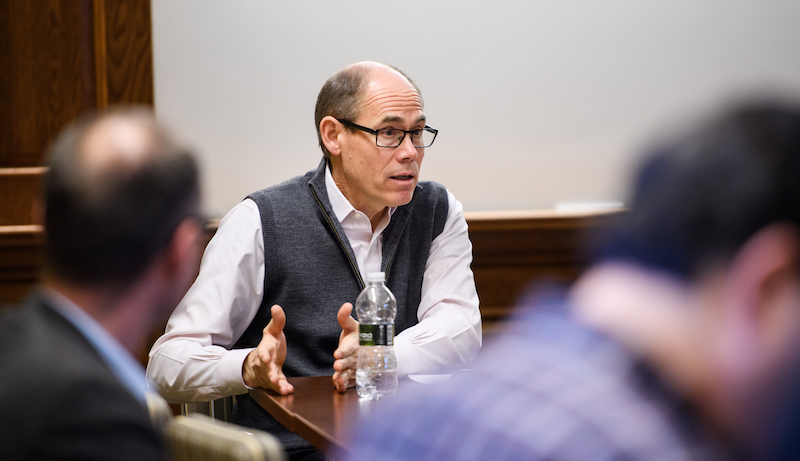
Professors tackle the challenges of regulating financial robo advisors.
Today, newly-developed computer algorithms recommend individualized health care and retirement plans to consumers. In the future, these “robo advisors” will do even more— recommending mortgages, credit cards, and other financial services to more individuals, all with increasing technical sophistication. Professors Tom Baker from the University of Pennsylvania Law School and Benedict Dellaert from Erasmus University Rotterdam have started thinking about how the regulatory world can adapt.
Some of the challenges of regulating robo advisors are not new. One problem—ensuring that these advisors make recommendations in the customer’s, not the company’s, interest—is the same one that a recent U.S. Department of Labor rule tackled for human advisors. Other concerns, like competence and social fairness, are as old as the earliest commercial regulations.
But the dawn of computerized advising will make these issues harder to address. At an event at the University of Pennsylvania Law School as part of the Optimizing Government Project, Baker and Dellaert explained that evaluating data and algorithms, as well as building regulatory capacity for this work, makes the problem of robo advisors especially challenging. In a forthcoming paper, they propose asking starter questions, gradually scaling up regulatory capacity, and creating contests to find the best components of these advising algorithms.
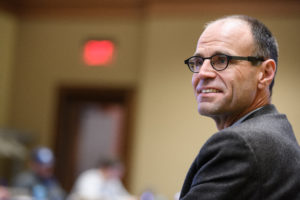
Professor Benedict Dellaert discusses concerns about reliance on robots for giving sensitive advice.
Regulating robo advisors will be challenging, and much remains unknown, but Baker and Dellaert have created a starter list of questions that regulators can ask industries to get more information. As Baker noted, “We don’t ask our regulators to design better algorithms. All that I think we realistically can ask a regulator is to ask good questions about the algorithms and to learn about them.”
Perhaps regulators might ask which consumer attributes and which product attributes are considered when making personalized recommendations. Because the data received by robo advisors help determine the ultimate recommendations they make to consumers, regulators should also ask about which data sources were—and were not—available to robo advisors.
After data are collected, the engineers and programmers writing the algorithms have many choices to make, and Baker and Delleart say those choices should also be monitored by regulators. In the short term, regulators should examine how the algorithms assign weights to different considerations. For example, if a robo advisor is recommending retirement plans, how much does it weigh the consumer’s age, risk tolerance, and other factors?
In the long term, Baker and Dellaert say regulators could examine which algorithms lead to the best recommendations: which algorithms, for example, recommended retirement plans that gave consumers the most gains over a long period of time?
But using acceptable data and algorithms is not enough. Baker and Dellaert say regulators should also examine how robo advisors present their recommendations to consumers. Known as “choice architecture,” social science research shows that people’s decisions change depending on how the options are presented to them. Outside the world of computers, for example, people recycle more when garbage bins around them are smaller. Programmers of robo advisers will make myriad choice architecture decisions, including how many product options to display to consumers, in what order, and with what information. Best practices in choice architecture exist, and the paper recommends that regulators ensure those best practices are followed.
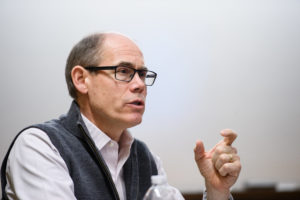
Professor Baker responds to a question during the workshop.
These are not simple tasks for regulators to monitor. Finding all available information—including all the credit card, mortgage, or investment product options that a consumer might purchase—is not easy. And even if all the data are present, those data might not be accurate, or even useful. As Baker explained, “The more you work with data, the more you realize how messy it is.”
Baker and Dellaert also underline a practical problem: Regulators lack the technological capacity to analyze algorithms in a complex way. As a solution to this, they recommend a scaling approach, starting with less ambitious regulation now and expanding it along with the emerging robo advisor industry.
They also recommend alternatives to traditional regulatory schemes, which Baker describes as saying, “Thou shalt do X.” Instead, they propose that regulators (or others) hold contests where algorithms compete to reach the best outcomes for consumers. Taking the idea a step further, Baker and Dellaert describe “contests of contests,” where regulators offer large cash prizes to those who create the best contests for measuring robo advisors’ success. The succession of new contests would provide an alternative to command-and-control regulation in an area where right answers are hard to find.
Baker and Dellaert describe their paper as “an exploratory essay.” Regulating robo advisors is a new field, and its problems are just now being illuminated. This paper is likely the first of many to grapple with the question of how to regulate the robots that help us decide.
This essay is part of a seven-part series, entitled Optimizing Government.