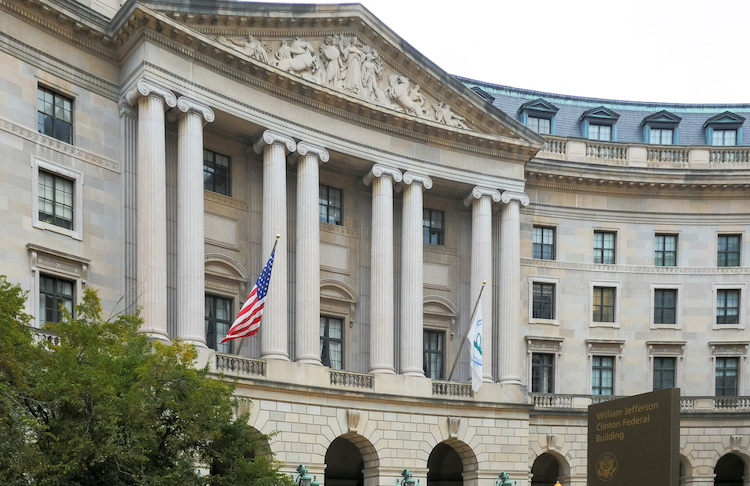
An agency’s regulatory experience informs the development of effective future programs.
The Administrative Conference of the United States’ recent recommendation, Learning from Regulatory Experience, has brought about renewed interest in encouraging agencies to learn from variation in existing regulations, conduct pilot programs, and “test” regulations in other ways.
Nobody doubts that policy pilots are one way that agencies can collect and analyze data while developing new regulations and examining those that are already on the books. There are, however, barriers unique to the regulatory context that can make successfully developing, implementing, and defending a pilot program challenging for government agencies. Fortunately, regulatory learning is built into the federal rulemaking process itself and is the norm for agencies like the U.S. Environmental Protection Agency (EPA).
In EPA’s case, rigorous analysis and data-driven decision-making are the underpinnings of its rulemaking process. Statutory provisions governing each regulatory area specify the many aspects of each problem that EPA must consider in developing its rules, and the Administrative Procedure Act directs that the agency’s rulemaking decisions be reasoned and supported by the record. EPA has internalized all of these requirements in its practices. Furthermore, along with conducting its own analyses, the agency hears from a broad range of stakeholders during the comment period, and often regulated entities will provide examples of how the current regulatory approach affects them.
An informal way that agencies pilot new approaches is to address one piece of the problem at a time. Courts have recognized that “agencies have great discretion to treat a problem partially” and that a particular regulation may be “a first step toward a complete solution.”
In this way, many rules function as informal pilots in that the agency will consider the results in the course of adapting requirements either over time or to other areas. For example, in advancing the agency-wide effort to bring permitting and enforcement into the digital age, EPA first issued a rule requiring electronic reporting for the National Pollutant Discharge Elimination Program. This has allowed the agency to get real-time feedback on both the benefits and complexities of harnessing modern technology before deciding whether and, if so, how, to extend electronic reporting to other areas.
Similarly, some regulations, such as the rule setting forth public notification requirements for combined sewer overflows to the Great Lakes basin, are defined in scope by both industrial sector and geography. Furthermore, even though EPA does not typically set time limitations when regulations are published, the agency is required under many statutory provisions to revisit certain regulatory standards on a periodic schedule, which can serve an analogous function to formal sunset provisions in certain respects.
As a result, rulemaking is inherently iterative, and the agency continually evaluates how regulatory programs are performing. Data about how the existing regulatory framework is working in the real world inform subsequent decisions to regulate, resulting in an informal retrospective review.
In addition, rulemaking is just one step in the agency’s implementation of its statutory mandate. Many of EPA’s regulatory standards are implemented through permits. Based on site-specific conditions or interest from the community, some facilities have agreed to permit conditions that call upon them to adopt new technologies, such as sensors that continuously detect water quality or fence-line monitors. Accordingly, permits can provide great opportunities for piloting new approaches to assess feasibility, cost, and value before deciding whether to replicate more broadly the innovations they contain.
A final non-regulatory vehicle through which agencies can experiment and gather information is technical assistance. EPA has, for example, conducted a pilot project to measure the effectiveness of compliance assistance. Its regional office randomly assigned auto body facilities with elevated levels of air toxics to either a treatment group that received workshops, webinars, and site visits, or a control group that did not. In the short term, the effects were statistically significant, although minimal because the overall compliance rate for both groups was high. But through the provision of technical assistance the agency can learn more about an industry and the impact of regulatory programs.
Still, information is expensive, and for the government there are unique hurdles to gathering the data necessary for formal retrospective reviews or comparisons. One consideration is the Paperwork Reduction Act, which requires approval from the Office of Management and Budget, a 30-day comment period, and a publication in the Federal Register whenever a federal agency solicits facts or opinions through identical questions posed to ten or more people. These requirements obviously discourage the use of after-the-fact surveys. For this reason, in many cases the most streamlined way to collect the information needed to assess program effectiveness is to build that into a rule at the outset. However, the agency does face a disincentive to include additional data-gathering requirements beyond what is necessary to run the program because doing so would increase the cost estimate for the rule and create additional burdens for regulated entities.
In addition, randomization, another important tool for gathering data because it isolates the effect of an intervention or program, is fraught in the regulated space because the differential application of requirements would often be in tension with the typical goal of providing a level playing field. If the regulation imposes costs or burdens, regulated entities that feel that they are at a competitive disadvantage will likely argue that the agency’s action is arbitrary and capricious. True, threshold cutoffs are commonly built into statutes or regulations, subjecting larger facilities to different or additional requirements. That said, using facilities that fall on different sides of the cutoff to compare the effectiveness of the two regulatory regimes can be complicated because sophisticated entities may have an incentive to modify their behavior to choose which program applies. For example, using a discontinuity regression methodology, one study found that concentrated animal feeding operations which fell just under the regulatory threshold were likely choosing not to expand in order to avoid triggering additional requirements.
Finally, because rulemaking is a resource-intensive process and many rulemakings take several years to complete, agency officials have an incentive to address as much of a particular problem as possible in order to be efficient, make an impact, and effectuate policies while in power.
Although agencies face unique legal and practical obstacles to conducting formal regulatory pilots, they are focused on data-driven decision-making in developing and evaluating the performance of regulatory programs. Agencies welcome data and studies from the public; experts outside the agency often provide analyses that inform future rulemakings. Indeed, the need to consider information gathered by the agency and submitted by the public is embedded in the Administrative Procedure Act’s requirements that the agency respond to comments and have a reasoned basis for its actions.
The views expressed here are those of the author only and do not necessarily represent those of the United States or EPA.
This essay is part of a 13-part series, entitled Using Rigorous Policy Pilots to Improve Governance.