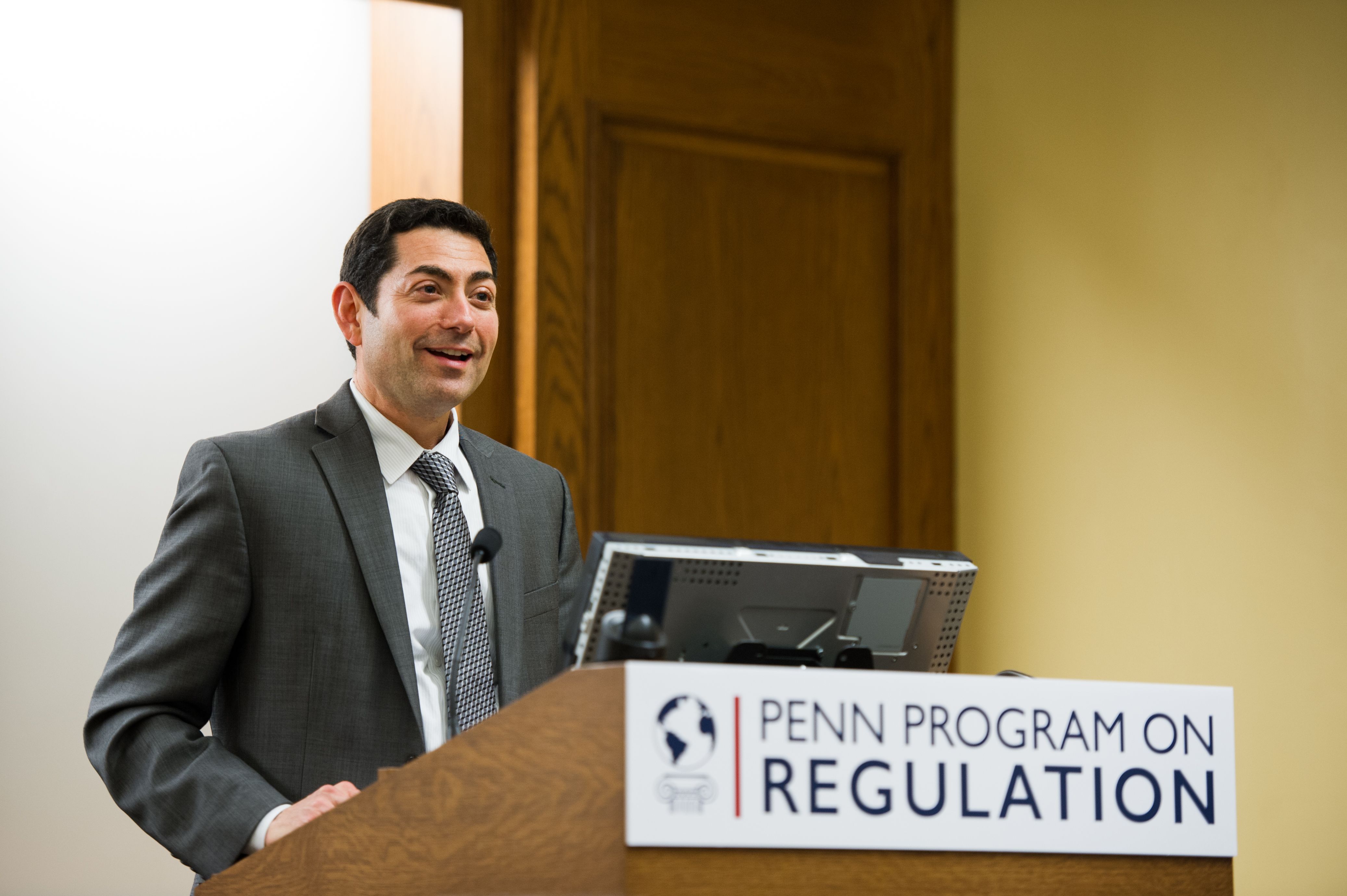
Agencies’ uses of sophisticated information technologies highlight the possibilities of administrative automation.
Let us start by acknowledging that humans make mistakes. Social psychologists, economists, political scientists, and even policymakers routinely acknowledge the limitations of how humans tend to consider probabilities, or otherwise weigh the consequences of particular decisions. Decision-makers may exhibit racial or gender biases, may over- or under-weigh the importance of a particular piece of information, may naively assume their own wisdom, or may insist on the naiveté of rivals. Even thoughtful experts who are familiar with the subtleties of environmental, national security, or public health data may fail to recognize patterns that can give agencies useful ideas about how to achieve their responsibilities.

Justice Mariano-Florentino Cuéllar delivers remarks at the Penn Program on Regulation’s annual regulation dinner.
It is certainly understandable, then, why societies could become interested in making greater use of computer systems that hold the promise of improving the quality and integrity of administrative decisions. Government agencies are beginning to rely more on computer programs to make decisions, and this trend will likely accelerate. An example involving federal regulation of pesticides highlights the subtle ways in which computer-based analysis and legal standards could interact—as well as the reasons why agencies may embrace new analytical techniques that heavily rely on automation.
The U.S. Environmental Protection Agency (EPA) administers the Federal Insecticide, Fungicide, and Rodenticide Act, which requires the registration of pesticides before marketing in interstate or foreign commerce. The current toxicity testing for pesticides depends heavily on assessing animals’ reactions to chemicals—a technique that can be easily criticized as costly, slow, and inhumane. At the most basic level, current toxicity testing methods limit the number of chemicals the EPA can test, even though it faces strong pressures to test more than 80,000 chemicals. But further, it limits the number of toxicity pathways one can test, the levels of biological organizations one can examine, the range of exposure conditions one can consider, and the life stages, genders, and species one can cover.
Given the inadequacy of current methods of toxicity research, the National Academy of Sciences published a report in 2007 calling for a transformative shift in toxicity and risk assessment and increased use of computational toxicology. In response, the EPA is introducing many different forms of computational methods in regulating pesticides.
Computation is also helping the EPA better calculate and predict environmental exposure to chemicals. Modern computational methods can build complex models that consider many variables that determine the level of exposure to toxic chemicals, such as the difference in exposure to animals versus humans, variability in exposure to humans, and the overall uncertainty of these predictions.
To support these efforts, the EPA is involved in massive data collection. It created the Aggregated Computational Toxicology Resource, a relational data warehouse for chemical and toxicity data from various public sources to support data mining and modeling. The EPA is also poised to start using virtual tissues; the agency is currently developing a “virtual liver” at the EPA’s National Center for Computational Toxicology.
The EPA’s reliance on computational toxicology underscores how agency decisions may increasingly implicate not only human choices about research methods, but architectural choices in the development of algorithms and neural networks to analyze data in new ways.
Changes in disability claims, too, may emerge as agencies seek to resolve logistical problems while compensating for inconsistencies of human judgment. In 2013, in an effort to reduce its reliance on paper records, to increase consistency across cases, and to automate some of its workflow, the U.S. Department of Veterans Affairs launched a computerized case management system for incoming disability claims. The software reportedly automates how the Department determines the level of different veterans’ disabilities for purposes of compensation. And importantly, it “calculates the level of disability—from zero to 100%—solely on the vet’s symptoms from the [self-reporting] questionnaire.” In essence, the software took over this responsibility for determining levels of disability from Department “raters”—human beings charged with determining a claimant’s entitlements.
Consider one additional example of automation, from a domain of responsibility shared by the public and private sectors: the testing of pharmaceutical products. As part of its review of new drug applications, the U.S. Food and Drug Administration (FDA) often considers “Population Pharmacokinetics” models, which test how drugs will interact with different bodies, depending on age, weight, and other factors. Traditionally, experts known as “pharmacometricians” would select several hundred statistical models (not real people) on which to test these drug interactions. As expected, choosing which models to include was time consuming and labor intensive.
As an alternative, the FDA recently approved a new drug application in which models were selected by an algorithm. According to the developer’s press release announcing the fact, such “automated model selection provides pharmaceutical and biotech companies results in less than half the time and at a lower cost compared to the traditional method.”
As these several examples suggest, greater reliance on artificial intelligence has much that will appeal to government officials. In the years ahead, government contractors will push new technologies to sell to administrative agencies. Outside lawyers will continue to criticize arbitrary agency decisions. Civil society groups will make the case for more predictable and analytically-sound administrative decisions. Taken together, these various pressures are likely to encourage agencies to find ways of relying on data and computer programs to make regulatory decisions.
And the promise of automation in the administrative state will not be entirely contingent on computer systems that mimic human interaction. Some travelers may prefer to be screened by even a fairly conventional computer system, rather than by an agent whose biases and limitations could color her judgment. After all, human decision-makers get things wrong.
The use of statistical and other predictive techniques by computers could improve not only individual decisions, but systemic bureaucratic performance as well. As computing technology improves, new possibilities will emerge to juxtapose two seemingly opposite qualities that could make automation more difficult to resist—the ability to analyze data and make predictions in subtle fashion that does not easily track human intuition, coupled with the capacity to make increasingly persuasive arguments to defend a decision.
But what, exactly, could more robust reliance on sophisticated information technologies accomplish? The simplest scenario is one where information technology duplicates what a human administrator could do, at a lower cost. Alternatively, the right expert systems could also screen out biases and certain heuristics that are considered, in the aggregate, to be undesirable, such as availability and vividness heuristics.
Even more intriguingly, computer programs could make it possible for government officials to analyze information for the purpose of predicting outcomes or responding to potential strategic behavior in a fashion that would be enormously difficult—if not impossible—for a human decision-maker to approximate. Massive concentrations of data analyzed by neural networks could generate intricate new predictions of how criminal enterprises, for example, adjust to new anti-money laundering measures, and what mix of counter-measures could help neutralize new forms of subterfuge to hide money corruptly stolen from foreign governments or obtained through fraud. Machine learning techniques could help food safety administrators further target scarce inspection resources to conduct the limited number of foreign inspections that are possible given existing resource constraints. These possibilities make it hard to ignore the opportunities for automating certain aspects of the administrative state—and all the more important to consider the normative questions that the uses of automation will raise.
This essay, part of a four-part series, draws on Justice Cuéllar’s forthcoming book chapter, Cyberdelegation and the Administrative State, which formed the basis of his remarks delivered at the annual regulation dinner at the University of Pennsylvania Law School.