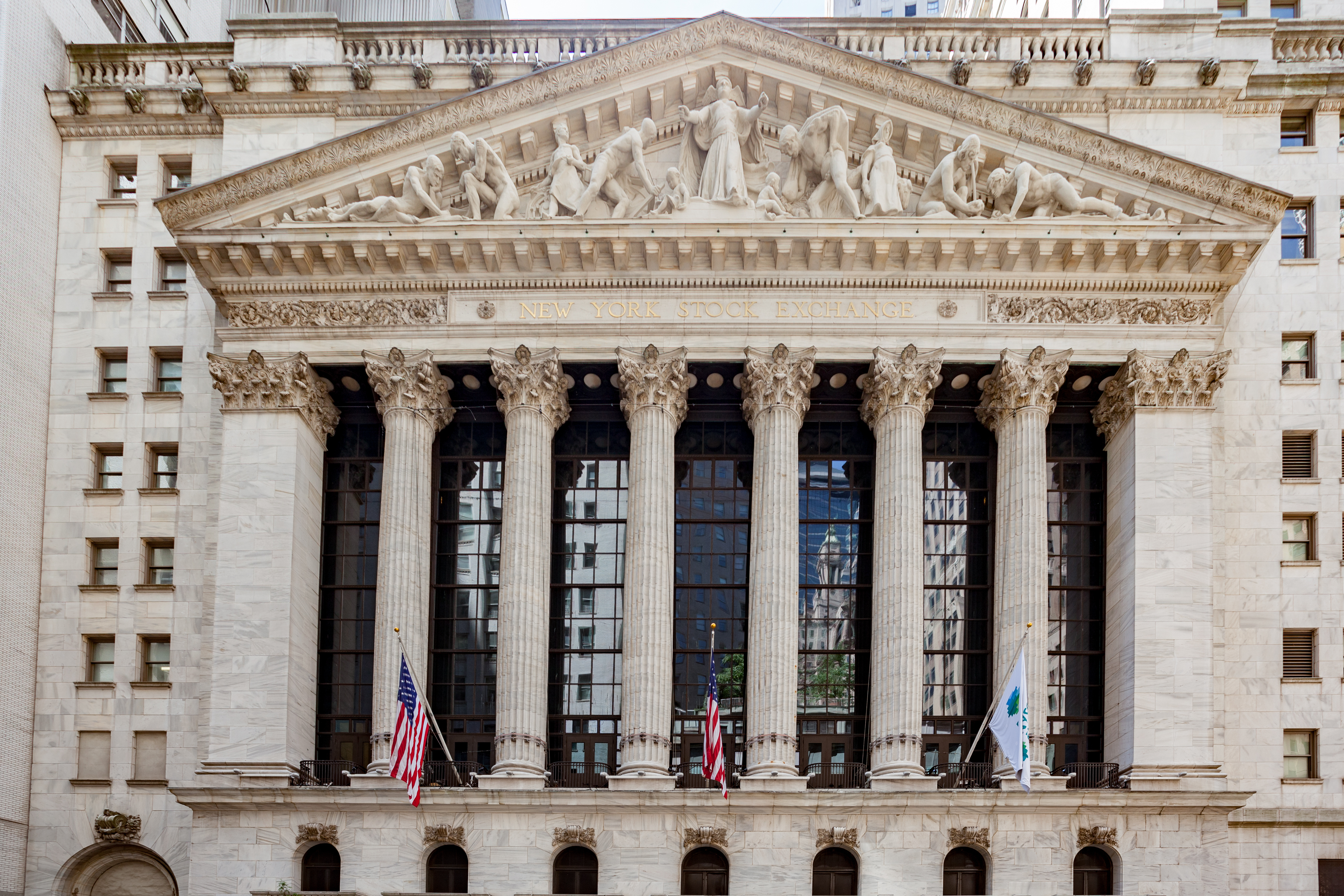
Scholars present framework for the regulation of automated advisors in the financial services industry.
Imagine you have just been given a modest amount of extra money that you would like to invest. If hiring a financial advisor is out of your price range, you could consider turning to a “robo-advisor” for help. Robo-advisors—or automated financial advice websites—are on the rise. Two leading investment management firms, BlackRock and Vanguard, have already instituted robo-advisor websites that work in conjunction with human advisors.
Although robo-advisors present the possibility of lower-cost, and potentially higher-quality, financial advice, they can also create a new set of challenges for the state and federal agencies that regulate the financial services industry. In a recent paper, two scholars consider the ways in which robo-advisors differ from human advisors and discuss the obstacles these differences present to regulators as they design regulatory schemes for robo-advisors.
Some of the problems that plague the financial services industry in its current, human-based form are likely to persist in dealings with robo-advisors. For example, financial advisors’ advice may be biased toward a certain client action that earns them a larger commission. Tom Baker of the University of Pennsylvania Law School and Benedict G. C. Dellaert of Erasmus University Rotterdam argue that the public should not assume robo-advisors would not be subject to the same misalignment of incentives, particularly when the robo-advisors are designed or purchased by financial intermediaries with the same incentives as human advisors.
Still, the authors contend that robo-advisors are different from human advisors in significant ways. New features differentiate robo-advisors from human advisors and present new regulatory challenges, such as the algorithms and processes that are the foundation of robo-advisors, the data required for robo-advisors to be effective, and the choice architecture that robo-advisors use to form their advice.
By Baker and Dellaert’s estimate, most or all robo-advisors run on algorithms that aim to match certain product attributes with certain client attributes. For example, a robo-advisor handling a retirement savings portfolio might recommend investments in different kinds of funds as the consumer ages. Baker and Dellaert argue that regulators must fully assess the competence of these algorithms.
Regulators must first gather information on how robo-advisors’ algorithms function. The authors suggest that regulators should require firms to provide “explanations of the models and the data upon which the models are based,” “explanations of the outcomes that the algorithms are seeking,” and “explanations of what other alternatives the robo advisor creators considered and rejected.” With this information in hand, regulators will be able to assess algorithms’ honesty by comparing the information provided to regulators with the information provided to consumers and by ensuring that the algorithms do not weigh factors in a manner that could harm consumers. Baker and Dellaert caution, however, that any actions taken based on this information should be “informed by domain-specific expertise.”
In other words, before these algorithms can effectively guide consumers, they must have high-quality data on both the products under consideration and the preferences and financial profile of the consumer. Baker and Dellaert argue that the extent to which this data can be obtained presents a potential roadblock to high-quality financial advice from robo-advisors.
Baker and Dellaert suggest that the barriers to complete data on the products under consideration are likely to result from business practices. For example, a business may not trust a robo-advisor with what it considers to be proprietary information. Other firms may simply not keep the kinds of records from which the requested data comes. Although collecting consumer data may seem easier on the surface—robo-advisors can collect the data from consumers as part of providing their services—consumer data could actually be just as difficult to collect because the requested data may not be easily accessible. For instance, consumers may not have easy access to the detailed asset and investment records an investment robo-advisor might need or the income and expense records required by a mortgage robo advisor.
As regulators begin to assess how to deal with access to data, the authors recommend that regulators consider several factors: whether a robo-advisor has accessed “reasonable sources of data,” whether gaps in the data will create biases that harm consumers, what strategies the robo-advisor used to try to fill data gaps and why it chose those strategies, and whether the regulator has the authority to increase access to the relevant data.
Once a well-designed algorithm has a sufficient data set, it can begin to rank options and present its conclusions to consumers. In presenting its conclusions, a robo-advisor will use a certain choice architecture—the design of how choices are presented to consumers. Baker and Dellaert argue that the choice architecture a robo-advisor uses will play an important role in how a consumer interprets the information provided by the robo-advisor. To bolster their argument, Baker and Dellaert point to behavioral science research showing that how options are framed and the number of options presented can have a significant impact on consumer decision-making.
The authors suggest that an important best practice for firms to employ—and for regulators to obtain the results of—is experimental testing. Experimental testing, they argue, will allow regulators to assess whether a robo-advisor has made a “meaningful and empirically informed” decision about choice architecture.
Baker and Dellaert conclude by warning regulators not to delay. They argue that the process of developing a regulatory regime for robo-advisors will be complicated, requiring substantial learning on the part of regulators. Because a relatively small scale adoption of poorly-designed robo-advisors threatens less public harm than a large scale adoption would, they argue that now “is [the] time for [regulators] to develop the necessary expertise.”