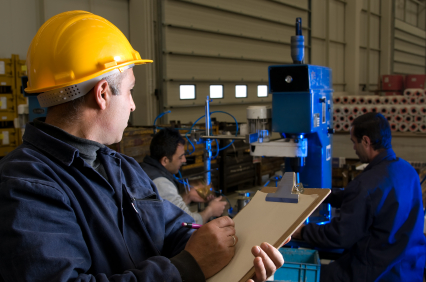
Using predictive targeting could better identify firms with the biggest risks to workers.
For many of the federal agencies that promulgate and enforce regulations to protect public health, safety, and the environment, the era of “big government” never even began. The U.S. Occupational Safety and Health Administration (OSHA) is a prime example: the agency employs about 2,000 inspectors, who are collectively able to visit roughly 100,000 establishments each year to look for unsafe and unhealthy conditions in the workplace.
This may sound like an ample effort, until one considers the scope of the problem: OSHA’s rules apply to more than 8 million establishments, and every year more than 4,500 workers die in traumatic occupational accidents, while epidemiologists estimate that about 40,000 additional workers die prematurely from chronic over-exposures to toxic substances.
According to data from the Labor Department, analyzed by the AFL-CIO, the ratio of OSHA inspectors to number of covered establishments is such that it would take OSHA between 26 and 243 years to inspect all of the jobsites in each state once only.
So OSHA—and the nation’s workers—can ill afford for its inspectors to spend time checking out
establishments that are fully compliant with all regulatory and other norms, while at the same time miss opportunities to find and fix instances of grave danger elsewhere, before workers are killed or stricken with disease.
This is where modern methods of statistical analysis, in particular the concept of “predictive policing,” come in.
When it is not responding to written complaints or investigating catastrophic accidents after the fact, OSHA schedules companies for proactive inspections using only one possible “red flag”—the self-reported injury rate in each establishment during the previous year. With the generous support of the Robert Wood Johnson Foundation’s Public Health Law Research program, my colleagues and I at the University of Pennsylvania are exploring ways to do better. OSHA’s enforcement data clearly show that targeting by injury rate alone leads the agency to many “sheep in wolves’ clothing” (firms that are fully compliant) and fails to find many true “wolves” (firms with low injury rates due to inaccurate reporting or that expose workers to conditions that have not yet caused grave harm).
We are creating a much richer database that combines what OSHA found in all its inspections during 2008 and 2009 with information on several dozen other characteristics of each establishment visited. We hypothesize that different “red flags”—in particular, indicators of financial turmoil and evidence that firms are flouting other regulatory requirements—are more strongly related to the severity of workplace hazards than the injury rates are. We have merged into the OSHA dataset two other large enforcement databases: one that tracks penalties and the number of calendar quarters in non-compliance for three major Environmental Protection Agency (EPA) programs (air pollution, water pollution, and hazardous waste disposal), and one that tracks similar information for several other Department of Labor programs dealing with fair wages, overtime pay, employment discrimination, and the like. We are currently adding to the merged datafile a large time-series of measures of credit scores, indebtedness, sales growth, ownership changes and other financial and managerial indicators at the establishment level.
Already we can see that knowing which firms were most in violation of EPA regulations could lead OSHA to more of the worst workplace settings. The graph below shows the cumulative distribution of OSHA penalties for four categories of establishments: those EPA had never previously inspected, those EPA found to be in full compliance with its rules, those occasionally out of compliance during the previous three years, and those found in violation of one or more EPA programs most or all of the time. Notice that the penalty distributions never overlap—for every common percentile point, the OSHA penalty grows higher the more non-compliant the firms are with respect to EPA. On average, OSHA found workplace conditions more than five times more severe at the EPA “problem sites” than at sites EPA did not see fit to inspect (the OSHA penalties follow a complicated formula that weighs the likelihood and gravity of the harm each violation is expected to cause).
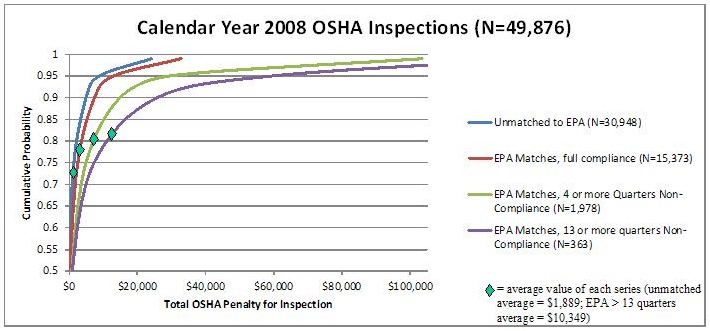
The algorithms we develop to gauge each establishment’s possible tendency to be an OSHA “hot spot” may also be able to reduce the “false negatives” in OSHA’s current targeting system. By looking at fatal accidents that OSHA investigated (reactively) in 2010-2012, we will see to what extent these occurred at firms that could have been inspected in 2008-09, but were not because OSHA arguably was not listening to the most powerful signals of harm.
Workplace inspections are public health interventions that should best leverage limited government resources to reach the places most in need of scrutiny. Predictive targeting is a non-intrusive way to listen better for the signals that firms are sending by their day-to-day behavior.
This essay originally appeared in Harvard Law School’s Bill of Health.