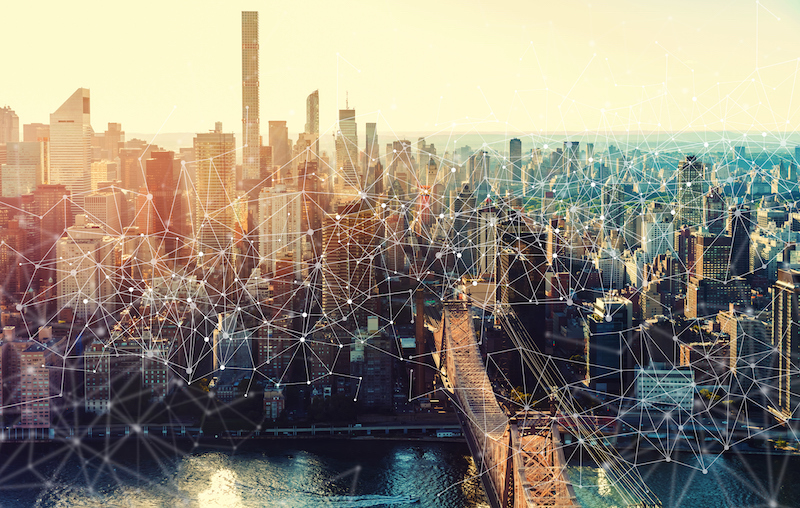
Facebook does not have a viable system to predict suicide in users.
In 2017, Facebook announced it was using artificial intelligence to predict suicide. Today, its artificial intelligence scans all types of user-generated content and assigns a suicide risk score to each piece of content. If the score is particularly high, Facebook may contact police and help them locate the responsible user.
Last year, I started analyzing the risks and benefits of this system in a series of articles. In October, I wrote that Facebook has revealed little about its suicide predictions, and because they are made outside the healthcare system, they are not subject to health privacy laws, principles of medical ethics, or state and federal rules governing health research. In December, I explained how contacting law enforcement based on Facebook’s predictions puts users at risk for warrantless searches of their homes, violent confrontations with police, and exacerbation of their mental health conditions. In January, I argued that Facebook should share its suicide predictions with medical researchers and computer scientists to ensure the predictions are fair and accurate.
After analyzing Facebook’s system, I have concluded that its public health risks are high, the benefits are unclear, and Facebook should conduct less rather than more mental health surveillance. Other commentators have reached the opposite conclusion.
In an article published in February, Ian Barnett and John Torous summarize previous articles on Facebook’s system including my own. However, their novel contribution is a proposal for increased mental health surveillance. Specifically, they suggest installing a second, and even more invasive, suicide screening program on top of Facebook’s existing system. Their proposed screening tool would continuously track each Facebook user’s fluctuating suicide risk over time.
Such a system would represent individualized mental health surveillance on an unprecedented scale. It is unlikely to be effective and would expose users to increased privacy and safety risks.
Facebook’s current system takes only a snapshot of each user’s activity and compares it to data derived from the rest of Facebook’s user base. This “population-level” reference data consists of keywords and phrases that Facebook believes are associated with increased suicide risk. Hypothetical examples include “goodbye” and “so much sadness.” If a user posts a status update or a comment containing Facebook’s keywords, then its artificial intelligence (AI) may flag that content as high-risk for suicide and give it a high suicide risk score. Facebook says it calculates risk scores only for pieces of content and not for the users who created that content.
Presumably, a piece of content is not linked to the responsible user until the content has been rated “high-risk” for suicide and a determination is made to notify police. At that point Facebook must link the score to the user in order to help police locate that person. The company claims it stores risk scores that are “too low to merit review or escalation” for no longer than 30 days, after which they are deleted.
Under the system proposed by Barnett and Torous, Facebook would be required to keep an ongoing mental health profile on every individual user, and it would store all suicide predictions indefinitely. According to Barnett and Torous, individualized models “are only effective when a person has been using Facebook for long enough to establish a history of comments and posts that are informative of suicide risk so as to train that person’s own model.” Essentially, Facebook would keep a personalized suicide prediction model for each user that would be updated over time, which would be a significant departure from Facebook’s current practices.
Not only is Barnett and Torous’s proposal overly invasive, it would likely be ineffective.
They claim the inaccuracies—that is, false positives and false negatives—of Facebook’s current system are due largely to its reliance on population-level data. They argue that an individualized system could compensate for those inaccuracies.
The inaccuracies in Facebook’s system, however, are not due to its reliance on population-level data. Instead, they stem from the fact that Facebook trains its algorithms on very low-quality input data. Specifically, the system is inaccurate because Facebook lacks access to real-world suicide data, a fact that Barnett and Torous fail to mention. Unlike medical researchers, who have access to real medical records, Facebook can only use rough proxies for suicidal behavior such as user reports of concerning posts.
For example, if a friend or relative of a Facebook user notifies the company about a suspicious post such as “goodbye world, I’ve had enough,” Facebook uses that information, and the subsequent response of its content moderation team, as a proxy for suicide risk. If the content moderation team ultimately decided to contact police in response to the post, then Facebook might use it as an example of a high-risk post for training Facebook’s suicide prediction AI. But there is one serious limitation to this approach: Facebook does not know whether the user who made the post went on to attempt or complete suicide. As a result, its approach is inherently inaccurate.
If medical researchers found the same statement—“goodbye world, I’ve had enough”—memorialized in a medical record instead of a social media post, they could look further into the medical record to see if that person went on to attempt or complete suicide. In other words, medical researchers can identify a real link between a statement and subsequent suicidal behavior. Unlike Facebook, they don’t have to guess, and they can use the information as high-quality training data for their prediction models.
By comparison, Facebook is operating with blinders on because it cannot see the ultimate outcome of the situation. Thus, Facebook’s algorithms suffer from the “garbage-in, garbage-out” problem that is common in computer science. Its poor-quality input data likely results in inaccurate predictions. Increasing surveillance of Facebook users by adding an individualized system on top of the existing system will not help because even an individualized system would rely on low-quality data unless Facebook somehow gained access to user medical records.
There is another problem. People who attempt suicide often do so impulsively, and they frequently have little or no history of mental illness. That is why an individualized approach will often be ineffective. Suppose a person suddenly attempts suicide without warning. If that person has no prior suicide attempts, and made no suspicious posts on Facebook previously, then his individual suicide prediction model would be unable to anticipate his suicide attempt. The model would have little or no data on which to base an accurate prediction. Thus a high-quality population-level approach using real suicide data is the right idea compared to an individualized approach. A person with no history of mental illness or suicidal behavior could be picked up by a screening program trained on high-quality training data because the system would have learned the behaviors that may precede a suicide attempt. But that is neither the system that Facebook currently has nor the system that Barnett and Torous propose.
The inherent inaccuracies of Facebooks system are dangerous because they have real-world consequences for people. Based on Facebook’s predictions, users may be detained, searched, hospitalized, and treated against their will. Their personal information may be transferred or sold to third parties, stolen, or used to discriminate against them. These outcomes can be traumatic and have devastating consequences for Facebook users. Yet Barnett and Torous fail to address these risks as they recommend even more surveillance of Facebook’s users.
There are very limited circumstances in which social media platforms should monitor user behavior for suicide risk. Live streaming of suicide attempts is one example. Outside of those limited circumstances, Facebook should adopt a “less is more” approach.
This essay is part of a 12-part series, entitled What Tomorrow Holds for U.S. Health Care.