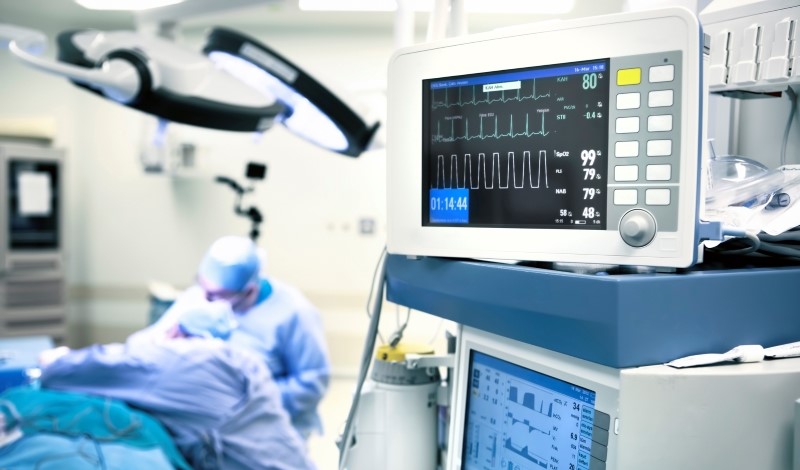
Scholars propose enabling patients to sue the makers and providers of discriminatory health care algorithms.
A few years ago, the health care software company Epic developed an artificial intelligence (AI) tool to help predict which patients are likely to miss medical appointments. One of the inputs in the system was prior no-shows. As a result, the tool apparently encouraged providers to double-book low-income patients, who are more likely to miss appointments due to difficulties arranging transportation, childcare, and time off of work. This alleged double-booking then risked creating a vicious cycle of more missed appointments and even more double-booking for these vulnerable patients.
In a recent article, Sharona Hoffman and Andy Podgurski, professors at Case Western Reserve University, argue that this type of unintentional discrimination from AI tools in health care settings may violate federal civil rights laws and a provision of the Affordable Care Act. They propose that the U.S. Congress amend these statutes to allow individuals to sue manufacturers or providers directly when they encounter discrimination that stems from algorithmic tools.
Electronic health records, as one major type of AI-driven health care tool, are especially prone to discriminating against vulnerable communities, Hoffman and Podgurski argue. They claim that the health records of minorities and economically disadvantaged patients are more likely to have missing data points in their electronic health records, due to lack of insurance, greater likelihood of missing appointments with providers, and other factors. As a result, the algorithms that scan these records to assess a patient’s potential for certain illnesses and conditions might erroneously find no such health risks.
Hoffman and Podgurski argue that the problem of missing health care data is only one contributor to the greater problem of algorithmic bias. Manufacturers feed certain datasets to AI-based health care tools to “train” their algorithms. But if a dataset does not accurately represent the patient population of interest, these algorithms can end up making predictions that do not match that population’s actual risks of disease and other health outcomes.
Not only does this bias lead to inaccurate predictions for patients, but it also creates negative feedback loops, Hoffman and Podgurski contend. Because vulnerable patients receive less precise care due to ill-fitted clinical prediction algorithms, training algorithms may incorporate this lack of precision into the AI-based health care systems.
Hoffman and Podgurski suggest that minorities and low-income individuals can easily find themselves trapped in worsening cycles of inadequate care if manufacturers do not step in to hard-code corrections into these medical AI tools.
For example, a small company in Toronto trained an algorithm to use patients’ speech patterns to diagnose Alzheimer’s disease. But the tool only correctly diagnosed “native English speakers of a specific Canadian dialect.” Its predictions for others were inaccurate.
Researchers later discovered that the company had only trained the tool with data from white patients from majority-white countries. The unrepresentative nature of underlying data likely contributed to the tool’s discrimination against non-native-English speakers, Hoffman and Podgurski note.
Medical AI tools can be especially discriminatory toward Black patients, Hoffman and Podgurski write. They describe several algorithmic determinations made by such tools that had the effect of reducing access to health care resources among Black patients. For example, they describe a heart failure prediction algorithm that systematically coded Black patients as being at a lower risk of death.
Despite the pervasive measurement errors and selection biases in health care AI tools, individuals seeking to sue manufacturers to hold them accountable for this discrimination lack adequate avenues, Hoffman and Podgurski argue.
Major federal civil rights laws that might seem to allow patients to bring discrimination claims—Title VI of the Civil Rights Act and Section 1557 of the Affordable Care Act—currently do not allow for lawsuits based on unintentional discrimination, note Hoffman and Podgurski.
They recommend that Congress amend these laws so that they do allow for patient lawsuits based on disparate impact without providers’ or developers’ discriminatory intent. Hoffman and Podgurski suggest that Congress can accomplish this effect by specifying that aggrieved patients have an individual right to sue companies that make and employ discriminatory medical AI.
Hoffman and Podgurski caution that amending Title VI of the Civil Rights Act to allow for greater anti-discrimination litigation could permit such lawsuits far beyond the health care context. They advise that a slightly more modest approach would be for Congress to add an individual right to sue for discrimination under Section 1557 of the Affordable Care Act, which would limit these lawsuits to the health care industry.
As an alternative to an easier pathway to patient lawsuits, Hoffman and Podgurski propose that Congress create a body that oversees and promotes “AI integrity.” Specifically, they recommend that Congress pass the Algorithmic Accountability Act, a bill that would require organizations and businesses to track and report the harmful and discriminatory impacts of their AI tools.
Hoffman and Podgurski conclude that, although the promise of AI is exciting for the health care arena, congressional action is ultimately needed to protect patients from harmful discrimination.