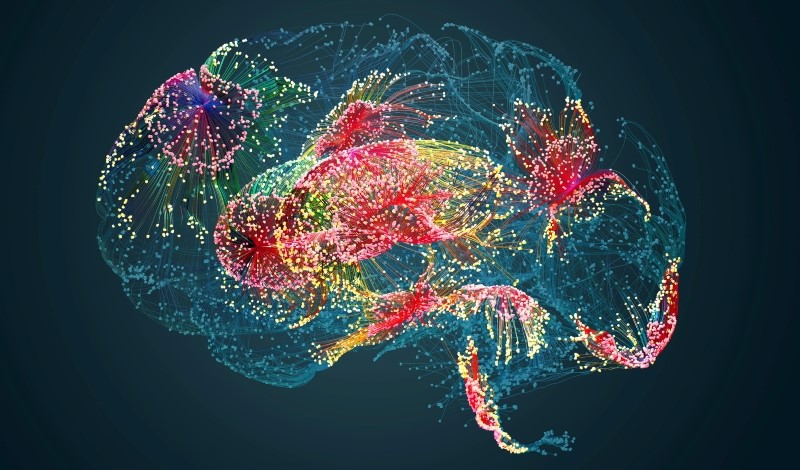
A scholar highlights problematic ways that artificial intelligence and health privacy interact.
As artificial intelligence (AI) continues to revolutionize health care, the need to protect personal health data has become increasingly crucial. But intervening attempts to protect personal health data may also slow health care AI development.
Society can establish a new balance between technological progress and data protection to protect personal health data without disrupting innovation. In a recent article, law professor W. Nicholson Price II explores the complex relationship between increased privacy protections and health care AI, offering a unique perspective on achieving a balance that may allow for both AI innovation and personal privacy.
AI-created privacy problems are rapidly evolving. Price discusses a new AI-created privacy issue: AI’s ability to find patterns in seemingly disconnected data may unintentionally reveal information never meant to be revealed. Price illustrates this problem through a real-world example of a large corporation whose AI analyzed shopping habits to infer pregnancy status—an observation unlikely to be made by human analysts.
Price also demonstrates how AI weakens mechanisms, such as deidentification, used to protect medical data to establish AI’s impact on medical privacy. Deidentification, Price points out, is a commonly used method of protecting medical privacy by removing identifiers from private information. The governing legal rule for health data privacy, the Health Insurance Portability and Accountability Act (HIPAA), only oversees identifiable health information and carves out a safe harbor for deidentified information.
By stripping the listed identifiers, users of health data can avoid HIPAA oversight. And AI is capable of doing just that. With enough computing power, AI can reidentify anonymous data. It can also make sophisticated guesses about a person’s non-health data.
AI has reduced the effectiveness of this deidentification, argues Price. He notes that researchers have used AI to reidentify “substantial majorities” of patients using deidentified information. Even as AI can reduce medical privacy, however, AI can use its ability to process large amounts of data to increase medical privacy by deidentifying highly specific health information that, before AI, was too costly to deidentify—allowing it to be used for research without revealing the patient’s identity.
The competition between increasing and decreasing privacy—and the resulting arms race between stronger privacy protections and AI that can defeat those protections—creates an “ongoing dysfunction” that informs Price’s recommendation for a new understanding of health privacy.
Even as AI may create problems for medical privacy, medical privacy can cause problems for AI. Price discusses how increased medical privacy can slow down AI development by making health datasets more expensive to use, less accurate, and even more difficult to create.
Many modern health and patient care products, such as telemedicine applications and patient and diagnostic tools, use AI, and the U.S. Food and Drug Administration has approved hundreds of AI-related products in the past few years. Although these products are creating new diagnostic, treatment, and organizational protocols, Price summarizes the privacy hurdles that stymy their more rapid development, such as privacy-protective regulatory burdens, dataset inaccuracies, and trust issues that harm data gathering efforts.
Developing accurate AI requires the use of large volumes of data. But removing identifiers from data to make them HIPAA-compliant decreases its usefulness for AI development because it becomes more difficult to develop accurate models. Sometimes, when long-term health records are required for AI training, privacy regulations make it challenging to connect data points to form a coherent long-term record. Price concludes that these hurdles increase AI development costs, slowing progress.
Biases in AI training datasets are another significant concern for health AI developers. Although some bias is inevitable, Price argues that privacy protections increase bias by raising data gathering costs, leading to less accurate datasets. Increased privacy protections can increase the cost of obtaining patient authorization and consent. As a result, only a small subset of hospitals share their data with health AI developers and other health care researchers.
These hospitals—ones with substantial resources, Price notes—tend to be academic institutions in urban areas and not rural hospitals or community health centers. But when health AI trained only on urban data is applied in a rural setting, the AI can perform worse, such as in the case of IBM’s Watson for Oncology.
Price demonstrates this worsened performance with the example of IBM’s Watson for Oncology, a health AI tool meant to improve cancer care. Watson for Oncology learned from data from the well-resourced Memorial Sloan Kettering Cancer Center in New York. But IBM chose to shelve Watson Health after data audits showed that the AI offered flawed treatment recommendations due to its geographically limited training datasets.
Price discusses a follow-up study that discovered how health AI datasets seemed to be “disproportionately trained on cohorts from California, Massachusetts, and New York, with little to no representation from the remaining 47 states.” AI trained on these datasets reflect the disproportionalities in the flawed data, causing AIs to encounter issues or perform poorly once applied to data from these underrepresented areas.
The data-gathering process itself can also cause bias issues, Price elaborates. Different populations have varying levels of willingness to allow their data to be used for research. Price argues that “the long history of systemic racism and prejudice that exists within the health system” has damaged trust in the health care system. This systemic mistrust increases the difficulty of gathering diverse data. As a result, health-related AI can be demographically unbalanced, leading to further bias.
AI’s ability to identify patterns in datasets can also introduce bias. Price illustrates this ability with an example of smartphones with differing privacy protections. Smartphones with more restrictive privacy protections tend to be more expensive, leading to demographic differences from users of cheaper phones. AI trained on data just from the more expensive smartphones may be less accurate when applied to data collected from users of the cheaper smartphones.
Price finishes his analysis with an argument for legal changes to the regulatory regimes covering health privacy. One way, Price argues, is for regulators to revise HIPAA to mitigate its potentially harmful effects on dataset creation—and the bias that may result from those harmful effects. The less inequality that HIPAA creates, the less it negatively affects health AI development.
Alternatively, Price suggests that individuals can lower their expectations of health privacy and allow their identifiable health data to be used for research—increasing the amount of information in datasets and improving the AI that learns from these datasets. In doing so, people can reap the benefits of better health AI. While balancing the need for health privacy and AI development can be challenging, Price concludes that the rewards of improving their complicated relationship may be great.