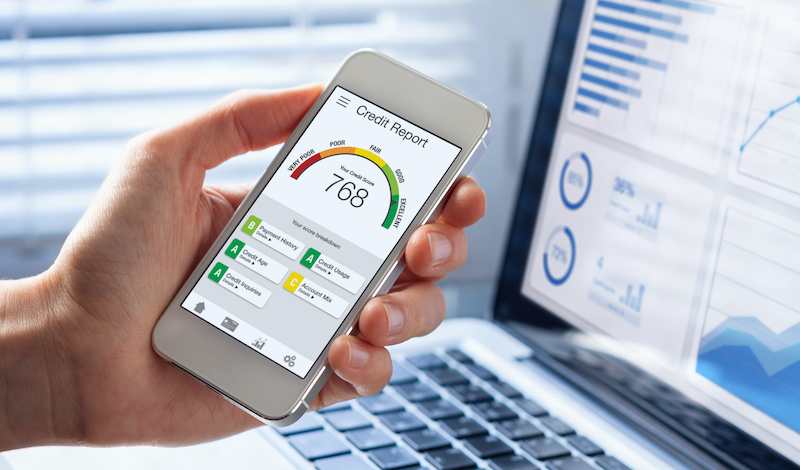
Scholar presents regulatory solutions to problems posed by using AI to determine credit scores.
Credit scores can control housing decisions, the cost of taking out a loan, and even employment. The advent of artificial intelligence (AI) in financial services poses a unique opportunity to improve fairness in the important arena of credit scoring—but it can also deepen the impact of bias.
In a recent working paper, legal scholar Nydia Remolina argues that financial regulators should step in to fill the gaps in areas where algorithms and data used in credit metrics fail to protect consumers.
These gaps arise out of the technologies used to advance AI in credit reporting. Companies that employ credit scoring models use proprietary algorithms protected by trade secret laws, obscuring the methods used to obtain these scores.
Remolina also describes how algorithms and data sourcing have become more complicated as technology has advanced, increasing—and obfuscating—the factors that enter into creditworthiness calculations. And machine learning is also often employed in these models, resulting in a “black box” that even algorithm owners cannot fully interpret.
Failure to address these gaps, Remolina explains, has led to demonstrated unfairness in assessing creditworthiness. Although discrimination in lending is not a new phenomenon, Remolina cautions that algorithms prevent potential discrimination claimants from knowing that they have been discriminated against in the first place.
Remolina further argues that the current approach taken by regulators—one that promotes, but does not mandate, transparency—fails to give consumers the tools to combat unfairness caused by algorithmic assessments. Although consumers in many jurisdictions are entitled to receive a report of their credit metrics, Remolina notes that the underlying process is often not disclosed, leaving consumers unable to learn from the data how to improve their scores.
Some consumers are further disadvantaged not just by algorithms but by data inequality. “Financially excluded groups,” or those who are classified as minorities or low-income, often have less credit data available for AI-based analysis, posing another problem that Remolina argues has not been addressed by regulators. And the boundaries determining what data are defined as financial data, and the use of what Remolina terms as “alternative data,” such as information derived from social media, has stymied regulators.
Other concerns generated by the use of AI in credit scoring are rooted in the financial service providers themselves. New competitors in these markets often fall outside of the purview of traditional regulatory authorities, which Remolina explains can negate the benefits consumers traditionally receive when competition increases, as these competitors can use their extensive data networks to identify and target at-risk consumers.
Remolina warns that current regulatory approaches are unable to address these challenges and she urges that financial regulators intervene in various ways to address problems with algorithmic credit scores.
One such solution she advocates would be to mandate that lenders test their algorithms periodically for discriminatory results. She notes that regulators currently focus on the inputs, rather than the results, of these algorithms, leading to unchecked biases. In addition to testing, Remolina urges providing consumers with data protections rights and the right to know the results of algorithms in the credit reporting space.
Remolina also argues that it is crucial to close the regulatory gaps created by new, nontraditional competitors in lending markets, such as digital lenders. She posits that all lenders should be required to provide information to borrowers about the credit reporting information shared with credit bureaus, providing consumers with greater transparency.
Remolina advocates the use of supervisory sandboxes—or experiments—in which lenders “approve loans to people with relatively low credit scores” to improve data and increase learning. These sandboxes would enable both regulators and companies engaged in assessing creditworthiness the tools to design better solutions.
Finally, Remolina contends that algorithmic accuracy and fairness could be improved by encouraging open data sharing between banking platforms and credit scoring agencies. She urges that this “open banking” approach allows for greater flexibility and consumer insight. As opposed to areas with new competitors and technologies, Remolina notes that data sharing in the banking arena receives traditional financial regulatory oversight, allowing for better control.
Although Remolina is unsatisfied with current regulatory approaches, she notes that steps have been taken in this area. In proposed legislation, the European Commission has classified AI credit scoring as high-risk, leading to the implementation of penalties and compliance guidelines.
These steps are “common globally,” says Remolina, but they fall short of providing a comprehensive response. Remolina concludes by urging regulators to implement her recommended proposals and “change their role in the governance of algorithmic credit scoring.”