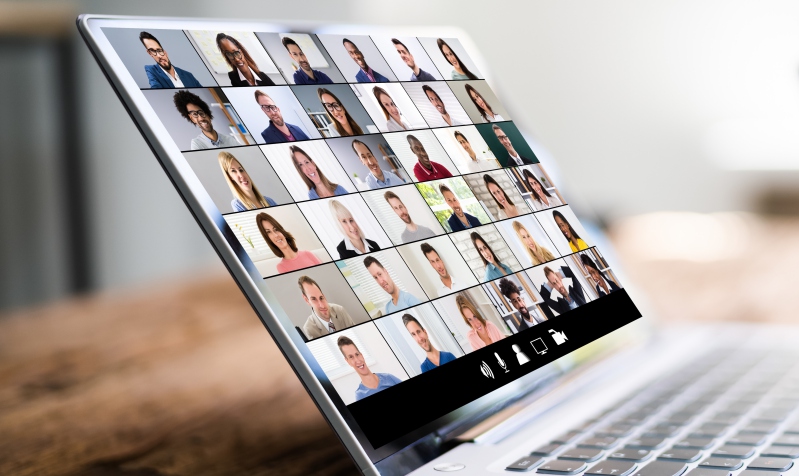
Regulators struggle to counter bias in hiring because algorithms reproduce existing inequalities.
What if hiring algorithms rejected job applicants explicitly for being women? Amazon’s experimental hiring algorithm did just that in 2015. Amazon sought to create a program to screen resumes for top talent, but it trained its algorithm using a decade of resumes from mostly male applicants. The algorithm replicated historical hiring patterns, discriminating against women applicants. Although Amazon abandoned the tool in 2017 before deployment, it illustrates how algorithms can reproduce existing patterns of inequality.
According to a LinkedIn survey, 67 percent of recruiters and hiring managers believe that artificial intelligence helps save time. The cost to fairness and equity, however, is unclear.
As recruiters use algorithms at more steps of the hiring process, bias can enter hiring decisions in several ways. Algorithms can influence hiring decisions by targeting job postings based on factors, such as age or gender, which limits who can apply. Programs that review resumes can lead to discrimination if resumes include a Black-sounding name, list a women’s college, or mention a disability. Algorithms that analyze video interviews may struggle with facial recognition for darker-skinned applicants, as well as penalize non-native speakers or people with disabilities.
Federal civil rights law prohibits employment discrimination based on race, color, religion, sex, and national origin. Other federal laws prohibit employment discrimination based on pregnancy, disability, age, and genetic information.
The U.S. Equal Employment Opportunity Commission (EEOC) issued guidelines in 1978 for how employers can and should choose their employees. Ten senators wrote to the EEOC in December 2020, requesting information about the Commission’s authority to investigate companies that offer hiring technologies. They raised concerns that more companies would use algorithms for hiring due to pandemic restrictions, which could reproduce and deepen systemic patterns of discrimination in the workforce. The EEOC has not yet responded.
In this week’s Saturday Seminar, scholars propose methods to counter discrimination in algorithmic hiring.
- Regulating algorithmic hiring practices will help move society closer to the national ideal of equal opportunity in spaces of employment, explains Ifeoma Ajunwa of University of North Carolina School of Law in an article for the Harvard Journal of Law & Technology. Ajunwa advocates requiring employers to conduct regular audits of automated hiring systems to meet their duty of care to use equal opportunity mechanisms. Ajunwa suggests that a regulatory auditing process of employment algorithms would encourage employers to pay attention to data retention and record-keeping practices.
- Technology companies that produce predictive algorithms for hiring should be accountable if their technology reinforces or worsens existing patterns of inequalities in hiring, argues Pauline T. Kim of the Washington University in St. Louis School of Law in an article in the Virginia Law Review. Kim explains, however, that individuals will find it difficult to succeed in court because they lack information about how companies customized their hiring experiences in potentially discriminatory ways. Instead, Kim recommends using regulation to address concerns about fairness and bias in algorithmic systems at the design stage to anticipate and prevent problems.
- Algorithms for workforce analytics can both mitigate biases and reproduce structural inequality, explains Stephanie Bornstein of University of Florida Levin College of Law in an article published in the Alabama Law Review. Bornstein recommends that regulators require employers to document their algorithmic choices before they use them in hiring decisions. She argues that existing discrimination law should strengthen employer liability to mitigate disparate impacts produced by algorithms.
- The EEOC’s guidelines may create a legal imperative for tech companies to use de-biasing techniques on their algorithms, argue Manish Raghavan of Cornell University and several coauthors in a 2019 working paper. Raghavan and his coauthors explain that employers can defend themselves in discrimination cases by justifying the disparate impact as a business necessity, but they will still be liable if plaintiffs produce an alternative business practice with less adverse impact. Raghavan and his coauthors suggest that vendors can easily reduce disparate impact in modern assessments, so de-biasing might be considered an alternative business practice that would render an employer liable if it is not undertaken.
- Federal civil rights law fails to address discrimination created by algorithms adequately, argue Allan Costa, Chris Cheung, and Max Langenkamp of the Massachusetts Institute of Technology. They warn that companies may evoke the Defend Trade Secrets Act to avoid sharing data that result in liability for discriminatory use of data by claiming the information is a trade secret. Costa, Cheung, and Langenkamp also argue that companies must realize that intellectual property law will probably not protect them from sharing algorithmic methods with civil rights groups or other auditors because intellectual property law only protects the “precise expression” of an algorithm.
- In an article published in the South Carolina Law Review, Matthew U. Scherer, Allan G. King, and Marko J. Mrkonich of Littler Mendelson P.C. explore how employers, agencies, and courts can ensure antidiscrimination compliance in algorithmic-based hiring tools. Scherer, King, and Mrkonich point to the “mismatch between the state of technology and existing legal standards” as an area of improvement for agency action. They propose a new framework to assess hiring algorithms based on standards of reasonableness, fairness, and the essence of the job itself. Scherer, King, and Mrkonich recommend that the EEOC clarify the appropriate legal standards for evaluating algorithmic hiring procedures as soon as possible to counter disparate impacts.
The Saturday Seminar is a weekly feature that aims to put into written form the kind of content that would be conveyed in a live seminar involving regulatory experts. Each week, The Regulatory Review publishes a brief overview of a selected regulatory topic and then distills recent research and scholarly writing on that topic.