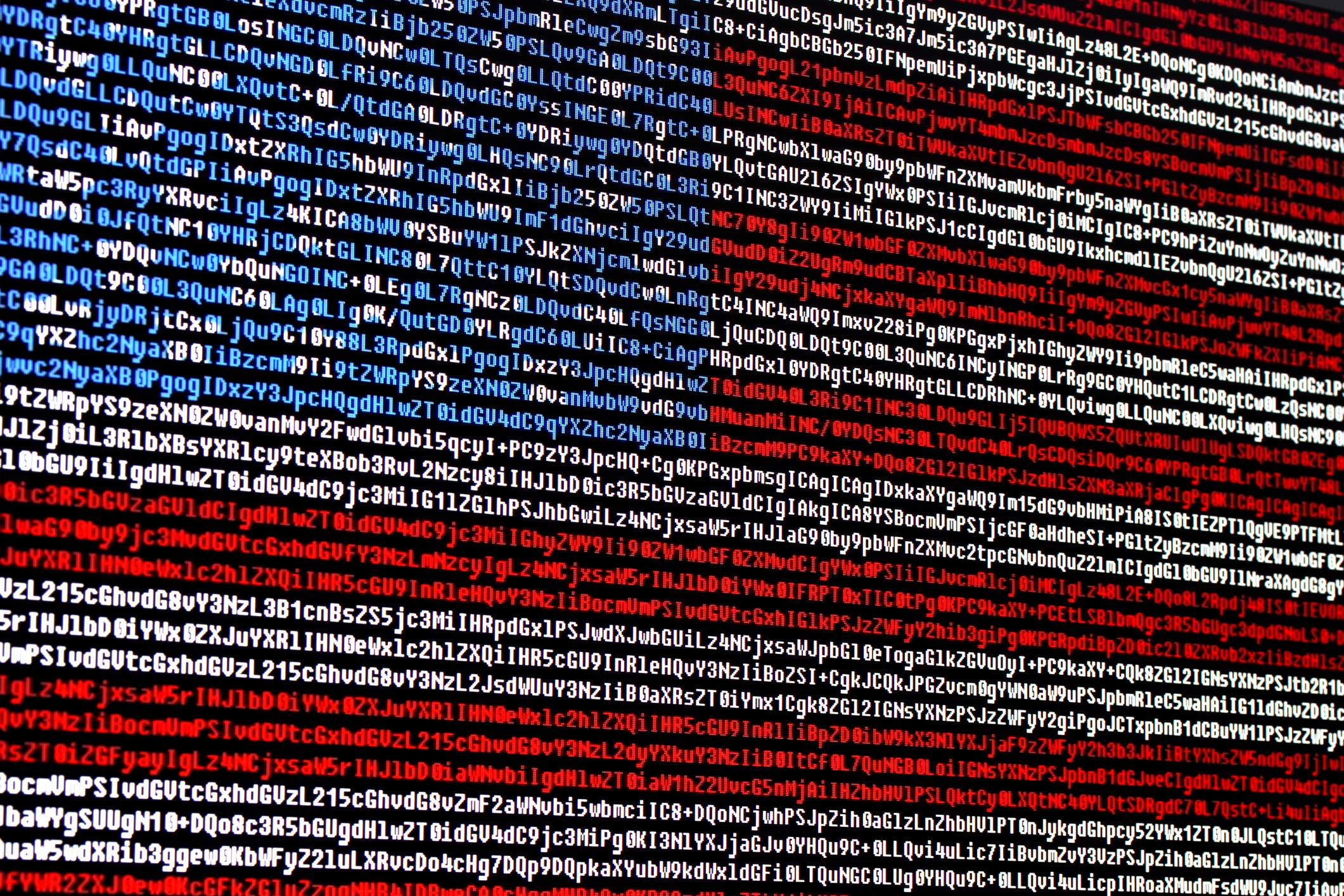
Governmental use of artificial intelligence can fit well within existing administrative law constraints.
The world of artificial intelligence has arrived. At the highest level, literally, commercial airplanes rely on machine-learning algorithms for auto-piloting systems. At ground level, again literally, self-driving cars now appear on public streets—and small robots automatically vacuum floors in some of our homes. More profoundly, algorithmic software reads medical scans to find cancerous tumors. These and many other advances in the private sector are delivering the benefits of forecasting accuracy made possible by the use of machine-learning algorithms.
What about the use of these algorithms in the public sector? Machine-learning algorithms—sometimes referred to as predictive analytics or artificial intelligence—can also help governmental organizations make more accurate decisions. Just as these algorithms have facilitated dramatic innovations in the private sector, they can also enable governments to achieve better, fairer, and more efficient performance of key functions.
But would the extensive reliance by federal agencies on machine-learning algorithms pose special administrative law concerns?
Overall, my answer is that machine learning does raises some important questions for administrative lawyers to consider, but that responsible agency officials should be able to design algorithmic tools that fully comply with prevailing legal standards.
These are real issues—not science fiction. Machine-learning technologies are already being put into use by federal agencies in the service of domestic policy implementation. Admittedly, the vast majority of these uses have so far raised few interesting legal questions. No one seriously thinks there are legal problems with the Postal Service using learning algorithms to read handwriting on envelopes in sorting mail, or with the National Weather Service using them to help forecast the weather.
And, with Heckler v. Chaney in mind, the case in which the Supreme Court held that agencies’ choices about who to subject to enforcement are ordinarily unreviewable, relatively few legal questions should arise when agencies use algorithms to help with enforcement, such as to identify tax filings for further auditing.
But we are rapidly moving to a world where more consequential decision-making, in areas not committed to agency discretion, could be aided by, and perhaps even replaced by, automated tools that run on machine-learning algorithms. For example, in the not-so-distant future, certain government benefits or licensing determinations could be made using artificial intelligence.
Such uses will raise nontrivial legal questions because of the combination of two key properties of artificial intelligence systems: automation and opacity.
The first property—automation—should be pretty obvious. Machine-learning algorithms make it possible to cut humans out of decision-making in qualitatively important ways. When this happens, what will become of a government that, in Lincoln’s words, is supposed to be a “of the people, for the people, and by the people”—not by the robots?
By itself, though, automation should not create any legal bar to the use of machine-learning algorithms. After all, government officials can already legally and appropriately rely on physical machines—thermometers, emissions monitoring devices, and so forth.
It is the second key property of machine-learning algorithms—their opacity—that, when combined with the first, will appear to raise distinctive legal concerns. Machine-learning algorithms are sometimes called “black-box” algorithms because they “learn” on their own.
Unlike traditional statistical forecasting tools, machine learning does not rely on human analysts to identify variables to put into a model. Machine-learning algorithms effectively do the choosing as they work their way through vast quantities of data and find patterns on their own. The results of a learning algorithm’s forecasts are not causal statements. It becomes harder to say exactly why an algorithm made specific determination or prediction.
This is why some observers will see automated, opaque governmental systems as raising basic constitutional and administrative law questions, specifically those involving the nondelegation doctrine, due process, equal protection, and reason-giving. Yet, for reasons I develop at considerable length in two recent articles, these questions can readily be answered in favor of governmental use of artificial intelligence. In other words, with proper planning and implementation, the federal government’s use of algorithms, even for highly consequential purposes, should not face insuperable or even significant legal barriers under any prevailing administrative law doctrines.
First, let us look at the nondelegation doctrine. If Congress cannot delegate lawmaking authority to private entities, then it might be thought that government cannot legally delegate decision-making authority to machines. Yet, algorithms do not suffer the same dangers of self-interestedness that make delegations to private human individuals so “obnoxious,” as the Supreme Court put it in Carter v. Carter Coal. Moreover, the math underlying machine learning necessitates that officials program their algorithms with clear objectives, which will surely satisfy anyone’s understanding of the intelligible principle test.
Second, with respect to due process, the test in Mathews v. Eldridge requires balancing a decision method’s accuracy with the private interests at stake and the demands on government resources. The private interests at stake will always be exogenous to machine learning. But machine learning’s main advantage lies in accuracy, and artificial intelligence systems can economize government resources. In most circumstances, then, algorithms should thus fare well under the due process balancing test.
Third, consider equal protection. Artificial intelligence raises important considerations about algorithmic bias, especially when learning algorithms work with data that have biases built into them. But machine-learning analysis can be constructed to reduce these biases—something which is sometimes harder to achieve with human decision-making. Moreover, due to the unique ways in which machine learning operates, federal agencies would likely find that courts will uphold even explicit inclusion of variables related to protected classes under the Fifth Amendment. The “black box” nature of machine learning will typically preclude inferences of discriminatory intent.
Finally, what about reason-giving? Despite machine learning’s black-box character, it should still be possible to satisfy administrative reason-giving requirements. It will always be possible, for example, to provide reasons in terms of what algorithms are designed to forecast, how they are constructed, and how they have been tested validated. Just as agencies now show that physical devices have been tested and validated to perform accurately, they should be able to make the same kind of showing with respect to digital machines.
In the end, although the prospect of government agencies engaging in adjudication by algorithm or rulemaking by robot may sound novel and futuristic, the use of machine learning—even to automate key governmental decisions—can be accommodated into administrative practice under existing legal doctrines.
When used responsibly, machine-learning algorithms have the potential to yield improvements in governmental decision-making by increasing accuracy, decreasing human bias, and enhancing overall administrative efficiency. The public sector can lawfully find ways to benefit from the same kinds of advantages that machine-learning algorithms are delivering in the private sector.
This essay is adapted from an address Professor Coglianese delivered at the June 2019 Plenary Meeting of the Administrative Conference of the United States.