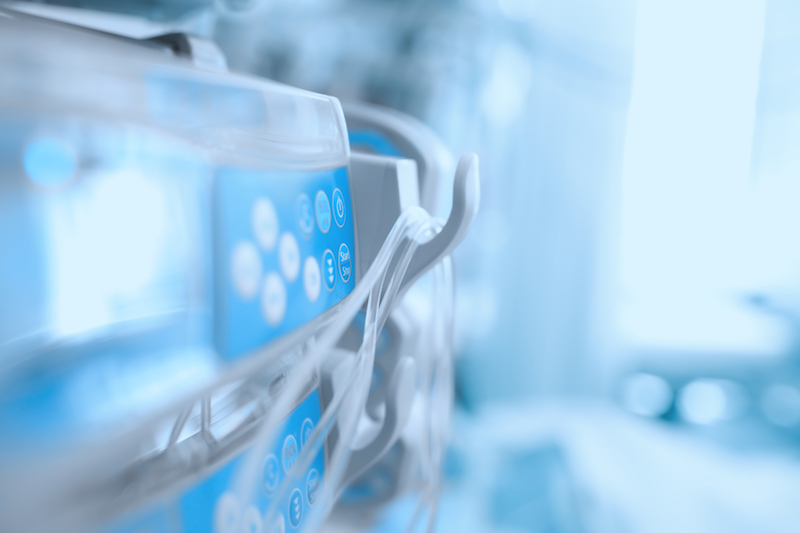
Scholar argues that FDA should reform its regulation of algorithm-based medicine.
In emergency situations, doctors have little time to save the lives of trauma patients. Gunshot wounds, car crashes, and other life-threatening harms often cause severe blood loss, which is the leading cause of preventable death when trauma puts patients’ lives on the line.
To manage the demands of these emergency cases, physicians today complement their medical skill-set with a new tool: algorithms.
But in a recent paper, a legal scholar argues that federal regulatory reforms must occur to unleash the full lifesaving potential of algorithms in health care. Nicholson Price, a professor at University of Michigan Law School, claims that the U.S. Food and Drug Administration (FDA) lacks the necessary expertise in computer science to apply its current regulations to medical algorithms and, as a result, could discourage much-needed innovation.
Price asserts that the FDA will likely categorize medical algorithms as high-risk regulated medical devices because doctors will use them like pre-existing diagnostic tools that already fall into this risk category. Because of the high-risk nature of these medical devices, FDA requires premarket approval—the most stringent regulatory requirement for medical device licensing.
In addition to the expectation that medical devices go through clinical trials, premarket approval requires medical device developers to present thorough evidence about their products’ safety and efficacy to FDA. Regulations require approximately six months for premarket approval, but the process usually lasts longer than this baseline requirement.
For the development and marketing of medical algorithms, FDA’s stringent regulatory requirements pose important challenges, argues Price.
More than anything, FDA needs greater expertise in computer science. Medical algorithms depend on machine learning—a developing field of artificial intelligence that seeks to enable computers to learn information without human action. Developers design medical algorithms in ways that enable them to adapt to new data input. If a medical algorithm computes the ideal dose of antibiotics for a patient, for example, it can adjust its recommendation as soon as medical professionals add new data into their databases.
Because medical algorithms change over time autonomously and in ways their developers cannot predict, their codes become moving targets, argues Price. Premarket approval of medical algorithms thus requires deep expertise in machine learning, which FDA currently lacks.
Moreover, premarket approval may stifle innovation, argues Price. Premarket approval is the “most involved, most expensive, and most time-consuming form of regulation in FDA’s arsenal of regulatory oversight,” according to Price. Because of FDA’s rigorous regulatory standards, premarket approval deters companies from developing and marketing appropriate medical algorithms.
Although FDA encourages developers to design medical algorithms through potentially profitable intellectual property mechanisms, like patents and copyrights, Price’s own research indicates the weakness of these incentives. Instead of risking unnecessary investments in the tedious premarket approval process, developers will choose to commercialize less regulated medical devices, claims Price.
Worse still, FDA may well decide to require premarket approval every time medical algorithms change, states Price. Because of their machine learning capacities, medical algorithms adapt “in real time or near-real time.” FDA’s current regulatory structure subjects all iterations of medical algorithms to close scrutiny—a standard that may prove unsustainable over time, observes Price.
To solve these problems, Price proposes three complementary approaches.
First, FDA should develop a more flexible regulatory regime. Instead of imposing stringent requirements on every medical algorithm available in the health care system, FDA should tailor its oversight strategies to the risks inherent in specific medical algorithms. If a medical algorithm seeks to inform decisions about whether to perform surgery or prescribe chemotherapy, for example, then FDA would require premarket approval. Yet, if a medical algorithm has little bearing on the welfare of patients, FDA should not impose severe regulatory requirements.
Second, FDA should implement a postmarket monitoring system. FDA currently focuses its enforcement resources on medical algorithms before they hit the market. But FDA cannot predict with enough certainty the quality of these algorithms before doctors use them on the job. FDA should thus police medical algorithms only when these computer codes have generated enough “real world evidence” to assess their utility, says Price.
Finally, FDA should use forms of indirect regulation like collaborative governance. Health care actors, like insurers, doctors, and hospitals, could regulate medical algorithms in ways that fit their needs. By enabling health care actors to assist in the regulation of medical algorithms, FDA can better ensure that it does not overregulate life-saving technologies, while empowering private actors to monitor their resources without invasive governmental oversight.
Price’s paper appears in the Michigan Law Review.