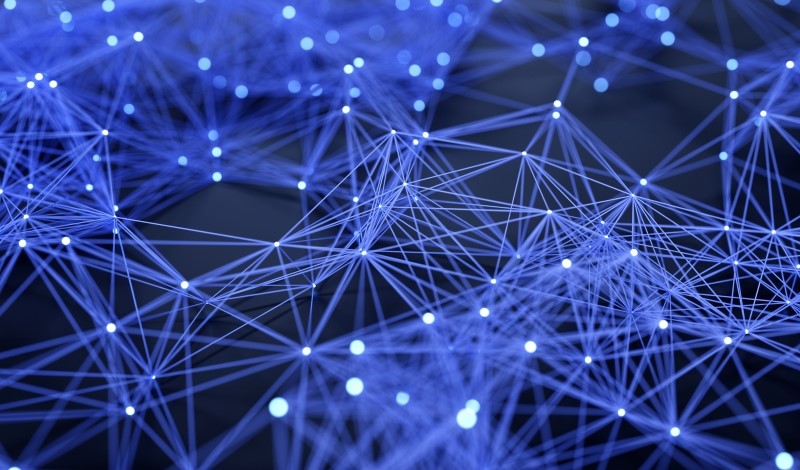
Management-based regulation is needed due to artificial intelligence’s extreme heterogeneity.
Artificial intelligence increasingly delivers valuable improvements for society and the economy. But the machine learning algorithms that drive artificial intelligence also raise important concerns.
The way machine-learning algorithms work autonomously to find patterns in large datasets has given rise to fears of a world that will ultimately cede critical aspects of human control to the dictates of artificial intelligence. These fears seem only exacerbated by the intrinsic opacity surrounding how machine-learning algorithms achieve their results. To a greater degree than with other statistical tools, the outcomes generated by machine learning cannot be easily interpreted and explained, which can make it hard for the public to trust the fairness of products or processes powered by these algorithms.
For these reasons, the autonomous and opaque qualities of machine-learning algorithms make these digital tools both distinctive and a matter of public concern. But when it comes to regulating machine learning, a different quality of these algorithms matters most of all: their heterogeneity. The Merriam-Webster Dictionary defines “heterogeneity” as “the quality or state of consisting of dissimilar or diverse elements.” Machine learning algorithms’ heterogeneity will make all the difference in deciding how to design regulations imposed on their development and use.
One of the most important sources of machine learning’s heterogeneity derives from the highly diverse uses to which it is put. These uses could hardly vary more widely. Consider just a small sample of ways that different entities use machine-learning algorithms:
- Social media platforms use them to select and highlight content for users;
- Hospital radiology departments use them to detect cancer in patients;
- Credit card companies use them to identify potential fraudulent charges;
- Commercial airlines use them to operate aircraft with auto-piloting systems;
- Online retailers use them to make product recommendations to visitors to their websites; and
- Political campaigns use them in deciding where and how advertise.
Even within the same organizations, different machine learning algorithms can perform different functions. An automobile manufacturer, for example, might use one type of machine-learning algorithm to automate certain on-road operations of their vehicles, while using other machine learning algorithms as part of its manufacturing processes or for managing its supply chain and inventory.
In addition to their varied uses, machine-learning algorithms can themselves take many different forms and possess diverse qualities. These algorithms are often grouped into several main categories: supervised learning, unsupervised learning, semi-supervised learning, and reinforcement learning. Within each category, the range of algorithms and their forms can be highly diverse. Naïve Bayesian models, decision trees, random forests, and neural networks are just a few types of supervised learning models. Even within any single type, finer points about how each model generated by an algorithm is structured, not to mention differences in the data used to train it, can lead each application of machine learning almost to fall within a category of its own.
Despite the wide variation in algorithms, it also remains that the same machine-learning model can be put to different uses within a single organization. For example, Meta—the corporation that owns Facebook and Instagram—has noted that, even though its “image classification models are all designed to predict what’s in a given image, they may be used differently in an integrity system that flags harmful content versus a recommender system used to show people posts they might be interested in.”
Added to the extreme variation in uses and designs of algorithms is the fact that, for many uses, multiple different algorithms are used in combination with each other to support automated systems. What may at times be referred to as “an” algorithm is often actually a suite or family of algorithms, integrated into an automated system or process in a manner designed to perform a specified function. Furthermore, these algorithms and their combinations are updated and changed over time, as new or refined algorithms are shown to do better. Today’s ChatGPT, for example, runs on models that are markedly different than earlier language models, and it and other large language models will only be updated, enhanced, and modified repeatedly in the years to come.
These changes in machine-learning models come on top of the fact that when the data processed by a learning algorithm changes, then so too can its performance. This means that, for some algorithms, their performance can be constantly evolving as they encounter and process new data.
That performance can also come along with a variety of problems. Just as machine learning’s form and uses can vary widely, so too can the nature of these problems. Some are safety concerns. Others are spillover effects or negative externalities. Others are privacy concerns. And then there exist important concerns about bias or discrimination and a host of other public policy concerns surrounding machine-learning algorithms.
What does the availability of ChatGPT, for example, mean for education? Do social media platforms that use machine-learning algorithms push content to users in ways that accentuate conflict, keep users distracted, or make them crave more time on their smart phones? Digital tools driven by machine-learning algorithms can also generate new artwork from existing works, raising questions about ownership rights and rules about appropriation. These tools can be used perniciously too, such as by facilitating the spread of misinformation or providing new opportunities for fraud through deep fakes. Pernicious actors can also use artificial intelligence to propagate cyberattacks that threaten both digital and physical assets.
As should be evident, the heterogeneous uses for machine-learning algorithms leads to a variety of regulatory concerns. It is surely axiomatic to observe that when the types of regulatory problems vary, regulation itself must vary as well to fit the nature of the problem. At the very least, regulation must be designed in a way that accommodates variation in uses and either targets diverse problems or provides appropriate incentives for regulated entities to find and address those problems.
As a result, regulators will need to pursue measures that take into account the varied and dynamic nature of these algorithms and their associated problems. It is impossible to specify a tidy, one-Machine learning’s heterogeneity will make flexible rules strong candidates for adoption. No one-size-fits-all “prescriptive” or “specification” standard will make sense, as that would necessitate the regulator telling firms exactly how to design, train, and use their algorithms. Regulators will almost surely never have sufficient capacity to regulate with such specificity.
An obvious alternative would be for the regulator to adopt performance standards that specify outcomes to be achieved (or avoided) but then give regulated firms the flexibility to decide how to proceed as long as they meet (or avoid) the outcome in the regulatory standard. As appealing as performance standards may be, they necessitate that the regulator will be able to specify the desired outcome in a clear, monitorable fashion—and then have the capacity to do the actual monitoring. Sometimes that might be the case, such as when machine learning is embedded in a larger system that can be observed independently and subjected to sufficient testing and monitoring. But in many cases it will be unlikely that regulators can develop sufficiently clear, monitorable performance tests for algorithms themselves.
When standard-setting organizations around the world have adopted voluntary performance guidelines for algorithms, they have tended to do so by articulating general performance principles calling for algorithms to yield outcomes that are “fair,” “safe,” “explainable,” and so forth. Although these principles-based approaches may be helpful in offering general guidance to industry, they are far from operational. It remains to be seen whether and how regulators could articulate with greater precision outcome values such as fairness and explainability. Even with safety, one must surely ask: Exactly how safe is safe enough? Absent an ability to specify outcome values in measurable and monitorable terms, it is hard to see how regulators could rely on a performance-based approach to the regulation of machine learning.
In situations where neither a one-size-fits-all prescriptive rule nor a performance-based rule seem likely to work, regulators have turned to an alternative regulatory strategy called management-based regulation. Under a management-based approach, the regulator requires the firm to engage in systemic managerial activities that seek to identify problems and then create internal responses to correct them. This approach has been widely applied to address other regulatory problems where heterogeneity dominates, such as food safety and chemical facility security. In these situations, the sources of the underlying regulatory problem are highly diverse and dynamic. The management-based approach typically calls for a regulated entity to develop a management plan, monitor for potential risks, produce internal procedures and trainings to address those risks, and maintain documentation on the operation of the firm’s management system. Sometimes these regulations also require firms to subject their management systems to third-party auditing and certification.
Management-based regulation will be an obvious option to consider for machine learning. This regulatory option does not demand that the regulator have the same level of knowledge the regulator be able to specify and measure all the relevant outcomes. It also gives firms considerable flexibility and thereby accommodates heterogeneity across firms and over time.
Unsurprisingly, many emerging soft law standards for machine learning are taking a management-based approach. The voluntary framework that NIST has issued to improve the trustworthiness of machine-learning applications, for example, bears all the hallmarks of a management-based approach. Specifically, it calls for firms to develop “structures, systems, processes, and teams” for “anticipating, assessing, and otherwise addressing potential sources of negative risks” and to put in place “rigorous software testing and performance assessment methodologies,” “systematic documentation practices,” and “plans for prioritizing risk and regular monitoring and improvement.”
Although the NIST framework is not mandatory, similar approaches are starting to emerge in regulations or proposed regulations in various parts of the world. Canada, for example, has imposed a requirement that its own federal government agencies conduct algorithmic impact assessments, quality assurance auditing, and various documentation measures before launching algorithmic systems that substitute for human decision-makers. A soon-to-be-adopted European Union regulation is expected to impose similar impact assessment and auditing requirements on both public and private sector machine-learning systems. These auditing and impact assessment requirements are management-based. They do not impose any specific prescriptions for the design and use of algorithms nor what outcomes they achieve — but they do direct firms to undertake a series of risk management steps.
In other contexts, management-based regulations have sometimes required firms to disclose publicly their plans and audit results. Mandatory disclosure is another likely option for the future regulation of machine-learning algorithms. Already, big-tech firms are starting to develop their own semi-standardized means of disclosing information about their uses of machine learning as well as the basic properties of the algorithms and the data on which they are trained and deployed. These voluntary disclosure efforts—what are known as “model cards” or “system cards”—could provide a template in the future for mandatory disclosure of information about machine-learning algorithms.
Yet for the same reasons that performance-based standards are unlikely to prove viable as a regulatory strategy, it is unlikely that any disclosure regulation could demand a unified outcome metric to be applied to all algorithms and all use cases. But any firm that has an internal management process supportive of the responsible use of artificial intelligence will necessarily generate some common types of information that could be disclosed. The disclosure of information from firms’ management of their algorithms would go some distance toward addressing concerns about machine learning’s opacity as well as providing consumers and the public better assurance that firms are testing, validating, and deploying machine learning in a responsible manner.
Research in other regulatory domains shows that management-based regulation can lead firms to reduce risks. But as much as management-based regulation has been demonstrated to work in other contexts and is conceptually well-suited for regulating machine learning, it is hardly a panacea. The evidence for the long-term efficacy of this strategy remains less clear and worries exist that managerial rigor and steadfastness by firms can atrophy over time. The possibility exists that, even if firms subjected to AI impact assessment and auditing requirements take their required risk management responsibilities seriously at first, these management-based requirements can become rote paperwork exercises over time. It is crucial that regulators build the capacity to assess the quality of firms’ management efforts and that regulators sustain rigor in their oversight of their management-based regulatory regime.
Vigilance is also needed simply because of the rapid pace of change. Machine learning’s future is a dynamic one and regulators need to equip themselves to make smart decisions in a changing environment. This means regulators must remain engaged with the industry they are overseeing and continue learning constantly. Regulators will make mistakes—they always have. But the key will be to try to minimize the consequences of those mistakes and, most of all, to learn from failures. Responsible regulation, like the responsible use of artificial intelligence, requires vision, attentiveness, and the capacity to learn and adapt. If regulation of machine learning is to succeed, it must be viewed as an ongoing pursuit of continuous improvement.
This essay is excerpted and adapted from the author’s article, “Regulating Machine Learning: The Challenge of Heterogeneity,” which appeared in the February 2023 issue of the TechReg Chronicle.