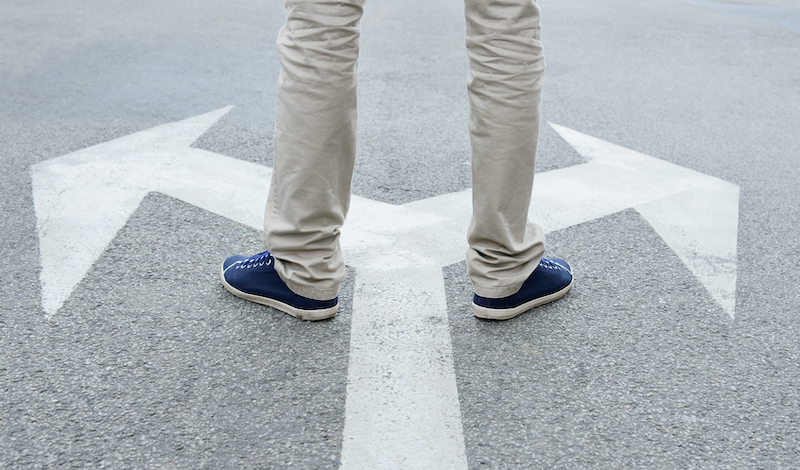
Scholars discuss how policymakers can help businesses predict future government action.
Accurately predicting government actions helps businesses minimize costs and operate efficiently. But as Yogi Berra said, it is hard to make predictions, especially about the future. Businesses often lack the necessary information to predict regulatory and legislative changes.
In response, the government should help produce and share this information, conclude Jonathan S. Masur of the University of Chicago Law School and Jonathan Remy Nash of Emory University School of Law. In a recent academic article, Masur and Nash argue that policymakers should consider forcing regulated entities to predict the likelihood of future government action and to disclose publicly whatever information they generate from that prediction.
These “prediction-forcing” regulations could help companies make more efficient, cost-effective decisions by aggregating other companies’ predictive information.
But presently, this predictive information remains widely dispersed among regulated entities and only available in bits and pieces. Masur and Nash call this dispersion the “assembly problem.” When the government contemplates new regulation, each regulated entity develops its own sliver of predictive information. But these entities cannot easily combine their information into a comprehensive whole.
Properly enforced, prediction-forcing regulations could solve this problem, according to Masur and Nash. They cite, for example, guidance from the U.S. Securities and Exchange Commission that directs public companies to disclose “management’s discussion and analysis” of financial risks related to future climate regulation. Consequently, a regulated company—known as a registrant—must predict whether pending legislation or regulation is “reasonably likely” to be enacted and, if so, disclose that action’s likely impacts on the company’s financial condition. In theory, then, as registrants disclose their predictions, other registrants could use that information to improve the accuracy of their own predictions.
Although the guidance document did not increase publicly available predictive information, Masur and Nash attribute this result to underenforcement, manipulation by registrants, and the relatively small effects of climate change on registrants’ operations.
Nonetheless, Masur and Nash maintain that policymakers should prefer prediction-forcing regulations to other methods of promoting regulatory prediction, such as greater governmental transparency or creating property rights for predictive information.
Although Masur and Nash suggest that greater transparency would be the most effective way for the government to improve private regulatory predictions, they conclude that regulators could not implement effective transparency measures. They worry that policymakers would characterize their actions in misleading ways to avoid disclosing information.
A statute that requires an agency to disclose transcripts from all official meetings, for example, would likely cause the agency to communicate more often in informal settings. Even if rules avoid this “leakage,” Masur and Nash argue that greater transparency could reduce the effectiveness of legislation and regulation by preventing government actors from efficiently cooperating on policy initiatives.
Masur and Nash also dismiss the idea that creating property rights for predictive information would enhance regulatory predictions. Rather, Masur and Nash claim that these rights are essentially unenforceable. Since firms use regulatory information to influence other corporate decisions, it is hard to prove whether one company based a decision on information owned by another company, they argue.
Market-based reforms like prediction markets also fail to promote the distribution of regulatory information, conclude Masur and Nash. Some scholars disagree, arguing that prediction markets—which pay participants based on whether certain outcomes occur—can successfully aggregate information. But Masur and Nash fear that the likelihood of government action is too abstract for investors to price accurately, which leaves prediction markets susceptible to “thinness”—a lack of liquidity due to a lack of participation—and manipulation by insiders.
Similarly, Masur and Nash reject regular capital markets—where investors buy and sell registrants’ securities, or tradeable assets—as a viable way to aggregate predictive information. Unlike the value of securities in predictive markets, the value of stock in a regular market depends on numerous factors that may not include a firm’s exposure to a regulatory risk, note Masur and Nash. As a result, companies cannot infer from stock prices any information about the likelihood of future regulation.
With no better options, Masur and Nash settle for prediction-forcing regulations.
Prediction-forcing regulations could, however, worsen the quality of government action, concede Masur and Nash. Because they would force a company to disclose its predictions only when the likelihood of a government action is reasonably high, prediction-forcing regulations might encourage companies to lobby to reduce the probability of unwanted regulation. If lobbying costs less than producing and disclosing predictions, companies will use their resources to defeat regulation rather than create and share predictive information, Masur and Nash fear.
These incentives could reward companies that thwart socially beneficial regulation for their own benefit. Policymakers must determine, then, whether the risk of losing socially desirable regulations outweighs the potential cost-saving benefits of prediction-forcing regulations, conclude Masur and Nash.