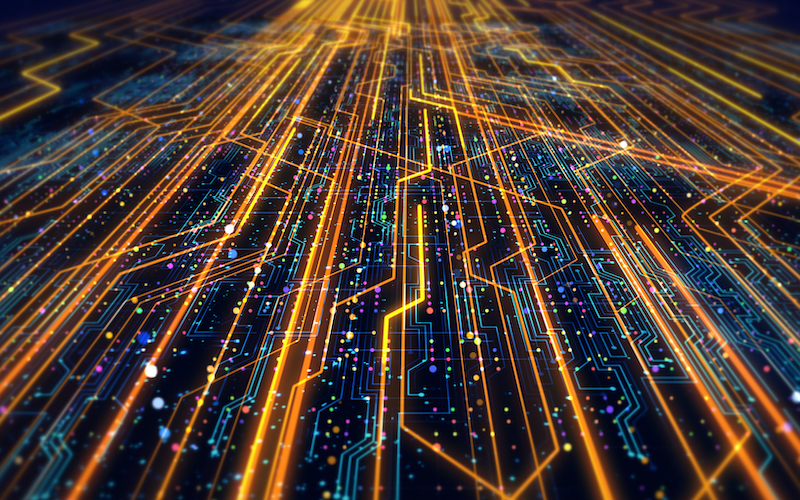
Scholars assert that government agencies need a policymaking mindset when purchasing machine learning technology.
Once merely the realm of science fiction, autonomous governmental decision-making machines are fast becoming reality. Agencies are increasingly relying on algorithmic systems to automate a range of processes, such as facial recognition software to identify criminal suspects and technology that scans veterans’ medical records for suicide risk.
But do the government agencies deploying such systems know how they operate?
In a recent article, Deirdre Mulligan and Kenneth Bamberger, professors at the University of California at Berkeley, argue that government officials are increasingly purchasing machine learning systems with little knowledge about their design or how well that design aligns with public values.
Mulligan and Bamberger argue that the administrative staff acquiring these systems often lack the requisite technical expertise to understand how the technology operates. Mulligan and Bamberger contend that such opacity can cause problems when technical solutions do not achieve their purpose, result in unintended consequences, or make biased decisions.
Mulligan and Bamberger state that agencies’ lack of knowledge about technology systems can be largely attributed to agencies’ procurement mindset when acquiring new products. Many agencies do not consider technology procurement decisions to constitute policy, despite systems often embedding important policy choices within the design. Instead, many agencies focus more on considerations such as price, fairness in the bidding process, innovation, and competition, as opposed to decisions about goals, values, risks, uncertainty, and constraints on future agency discretion.
In their article, Mulligan and Bamberger provide the example of Wisconsin’s use of computer software to predict the likelihood of an individual engaging in future criminal activity at criminal sentencing. In Loomis v. Wisconsin, the state of Wisconsin conceded that it was unclear how the system accounted for gender. Mulligan and Bamberger argue that deciding how to account for gender during sentencing was an important policy question. Yet it was not evident whether the state government deliberated about how gender was to be used during the procurement of the system or its application during sentencing. Rather, the evidence suggested that the ultimate decision was left to the software vendor’s discretion.
When systems embed policy, Mulligan and Bamberger argue that a procurement approach fails to meet key administrative law requirements, namely that substantive decisions not be arbitrary and capricious, and that agencies follow a transparent reasoning process. Instead of considering the important policy choices inherent in machine learning, agencies are instead treating purchases as normal government contracting decisions.
Mulligan and Bamberger argue that, when policy decisions are made through system design, agencies need to shift from a procurement mindset to a policymaking mindset. This policy mindset is not needed for all technology purchases; Mulligan and Bamberger distinguish between inward systems and those that create public-facing policy about which agencies should deliberate.
In considering where to draw the line between different systems, Mulligan and Bamberger turn to two established administrative law questions that the courts ask to assess whether an agency’s decision is legislative: Does the agency action in question prospectively limit the agency’s discretion? If so, do any legal consequences flow from the action?
Considered against these questions, Mulligan and Bamberger argue that many embedded policy decisions in machine learning are legislative, such that agencies need to justify design and policy choices.
As examples, agencies should justify decisions about system goals, how to operationalize the goal into a target variable, and what modelling framework to use. In addition to these technical matters, Mulligan and Bamberger assert that agencies need to be more transparent with the public about a system’s code, underlying models, limits, assumptions, and training data, along with the fact that the agency is engaged in policy decision-making.
To address agencies’ justification problem, Mulligan and Bamberger envisage how agencies could adopt a policy mindset to satisfy administrative law standards when acquiring machine learning technology.
First, to enable reasoned expert deliberation, Mulligan and Bamberger argue that governmental agencies need to overcome their lack of technical expertise. Mulligan and Bamberger state that the Obama Administration’s centers-of-expertise provide a compelling model to address this issue.
This approach would involve establishing pools of technical experts who can provide consultation services to agencies on technology design matters. Mulligan and Bamberger also recommend developing guidance documents and methods to standardize the questions and processes that federal agencies use to assess and design algorithmic systems.
Second, agencies could introduce “political visibility” into their deliberation by using algorithmic impact assessments that consider technical aspects of system design and surface potential political implications of choices.
Mulligan and Bamberger argue that these statements, when publicly disclosed, provide a common reference point for the public and experts to consider collectively the “points of policy within a machine learning system.” Although such assessments would not necessitate a substantive outcome, this approach would force administrative agencies to identify, recognize, and explain their policy choices.
Finally, Mulligan and Bamberger argue that it is important that technology systems use “contestable design.” That is, systems need to reveal their inputs and provide for iterative human involvement as the system evolves, so that agency staff can remain aware and participate as the policies embedded in the systems develop.
Mulligan and Bamberger conclude by clarifying that they do not consider that machine learning systems should be “procured at peril” due to fear of abdicating important policy decisions. Rather, government agencies should engage with such systems by questioning their biases and blind spots, as well as learning from and teaching them.
It is through reasoned policy and design choices that agencies can better align algorithmic systems with administrative law requirements.