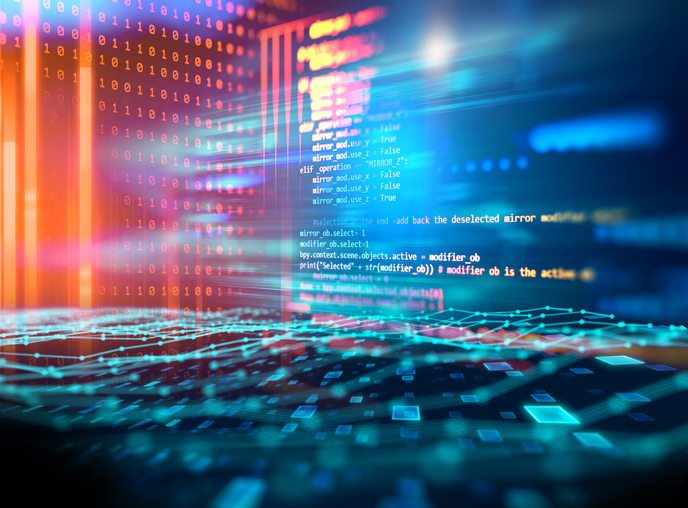
Federal guidance on artificial intelligence needs additions to ensure the U.S. has a seat at the international table.
The rapid proliferation of applications of artificial intelligence and machine learning—or AI, for short—coupled with the potential for significant societal impact has spurred calls around the world for new regulation.
The European Union and China are developing their own rules, and the Organization for Economic Cooperation and Development has developed principles that enjoy the support of its members plus a handful of other countries. In January, the U.S. Office of Management and Budget (OMB) also issued its own draft guidance, ensuring the United States a seat at the table during this ongoing, multi-year, international conversation.
The U.S. guidance—covering “weak” or narrow AI applications of the kind we experience today—reflects a light-touch approach to regulation, consistent with a desire to reward U.S. ingenuity. Critics say the White House is embracing “permissionless innovation,” which involves the development and circulation of products or services without prior approval from regulators. Supporters have argued that the dynamic, boundary-pushing innovation principle is better than the restrictive precautionary principle.
As someone who has studied the application of AI in government and industry, I believe the draft guidance should be informed by current experience, of which there is no shortage.
In a recent report on smart manufacturing, my colleagues and I conclude that AI is suited to applications based on large amounts of high-quality data and will be resilient to potential errors because of one or more of three factors: (1) the consequences of failure are minimal; (2) the context is constrained; and (3) a human overseer is present. A manufacturing environment is a good candidate for AI applications—data are plentiful, the environment is restricted within clear operating parameters, and trained humans oversee automated systems.
Indeed, within the last five years, technological progress has led manufacturers to employ AI for a wide variety of purposes: workforce training; product design; production process improvement; quality control; predictive maintenance; supply-chain optimization; distribution of goods; and creation of AI-embedded products.
And because manufacturers are already highly regulated, three types of regulatory challenges to AI-enabled innovation are emerging.
First, outdated regulations can preclude innovation. To illustrate, some federal motor vehicle safety standards were written under the presumption of a human driver. Unless changed, these standards preclude autonomous vehicles that would rely on narrow AI applications currently under development. Federal regulators recently acknowledged this problem.
Second, uncertain regulatory requirements impede investment. A few regulatory programs create uncertainty for manufacturers considering investment in AI. For example, the U.S. Food and Drug Administration (FDA) requires drug manufacturers to follow Current Good Manufacturing Practices (CGMP), which are FDA regulations that help to ensure proper design, monitoring, and control of manufacturing processes and facilities. Are AI-enabled factories allowed under CGMP? Manufacturers wishing to invest in such applications need to know the answer.
Third, new regulations can serve as barriers to market entry. Some products require a green light from regulators before entering commerce. When such products are AI-enabled, such as medical devices based on machine learning or aerospace parts created by generative design, regulators face novel issues that may significantly delay approval.
Given these emerging challenges, how should AI be regulated? Let me suggest three principles—each of which is embodied in the draft OMB guidance.
First, government should not regulate an activity simply because AI is being applied; regulation should be based on the nature and magnitude of the risk posed by the application.
This first principle is similar to a long-standing principle found in the federal coordinated framework for the regulation of biotechnology, first issued in 1986. In that framework, a transgenic organism is regulated based on the risk posed by the new organism or product. Likewise, AI should be regulated based on the nature and magnitude of the risk posed by the application.
Second, existing regulatory programs can and should be used to ensure societal concerns are addressed. It is only when these existing regulatory programs are inadequate that new guidance, new regulation, or changes to existing regulation should be considered.
In some cases, AI applications are already covered by local, state, and federal regulation. An example of such an existing regulatory program is export controls, where certain algorithms are considered dual-use technology subject to licensing by the U.S. Department of Commerce. This principle is also similar to a tenet of the 1986 coordinated framework for the regulation of biotechnology—namely, to rely on existing statutory authorities whenever possible.
Lastly, any needed regulation should be proportional to the risk posed by the application and should do more good than harm.
By focusing on the risk posed by AI applications, OMB’s draft guidance recognizes that the consequences of AI are what matter. And the draft guidance recommends forgoing new regulations if existing regulations are sufficient or if a national standard is not essential.
Despite the inclusion of these principles, OMB’s draft guidance is far from complete. Four important complementary policies or actions will be needed if the guidance is to ensure public trust—a goal of the Trump Administration.
First, the coverage of the guidance should be extended to governmental applications. Limiting coverage to only the private sector is insufficient to ensure public accountability and foster public trust. A recent workshop illustrated many ongoing governmental applications of AI technology, including those that have emerged from collaborations between government and industry, complicating any clean regulatory distinction.
Second, maintain federal research and development leadership in explainable AI and trustworthy AI. Efforts such as those within the Defense Advanced Research Projects Agency will go a long way toward addressing the opacity issue—that is, the “black box” nature of AI.
Third, develop the capacity to investigate incidents where AI applications have gone awry. For example, because the National Transportation Safety Board investigates transportation accidents, it will have to investigate accidents where AI is at issue. Federal regulators must develop expertise in AI and interpreting AI-enabled decisions.
Finally, report annually to Congress on the regulatory oversight of AI. This requirement can be mirrored after the OMB annual report on the costs and benefits of federal regulation, or perhaps it could even be folded into this statutorily required report.
With these improvements to the OMB draft guidance, the United States will have a stronger voice in international discussions.