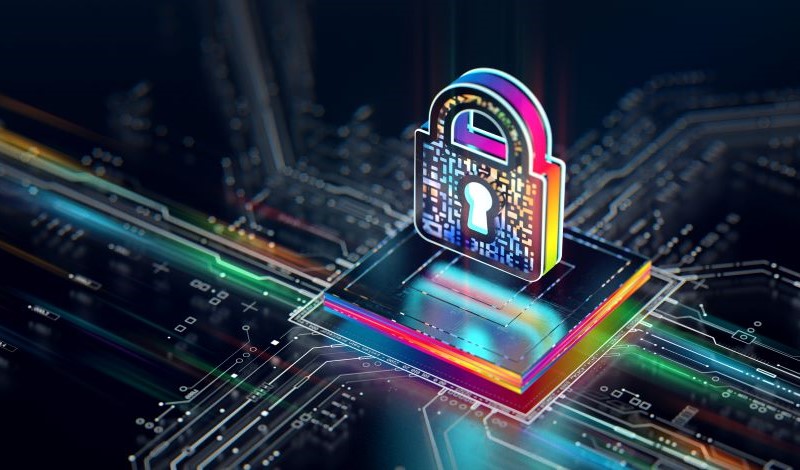
AI governance should center on novel threats rather than familiar risks.
A new chapter of artificial intelligence (AI) governance is underway, catalyzed by two significant developments. First, DeepSeek’s success in developing an advanced, open-weight model at comparatively less expense than leading labs in the United States demonstrates that reaching the technological frontier is more accessible to more actors than previously anticipated. The economics of capability development have fundamentally shifted, enabling a broader range of entities—including potential adversaries—to create and deploy advanced AI systems with relatively few resources. Second, Vice President JD Vance’s speech at the Paris AI Action Summit and remarks at the American Dynamism Summit effectively undercut whatever momentum had been building toward a unified international approach to AI safety, signaling a pivot away from global governance frameworks toward more nationalistic approaches to AI development and regulation.
If, as Vice President Vance said, the Trump Administration is done talking about AI safety, then AI security now serves as the dominant regulatory narrative. That clear pivot, however, introduces a larger question: What does AI security entail?
AI security is fundamentally concerned with AI’s “capability leaps”—attributes that create new “threat vectors,” or methods for security attacks. A focus on AI security involves turning the vast preponderance of regulatory attention and resources to defending against threats enabled by AI’s novel capabilities that represent a marked jump beyond existing dangers. These “threshold-crossing capabilities” allow malicious actors to accomplish harmful objectives that were previously impossible or impractical. Unlike mere intensifications of familiar problems, these capabilities fundamentally alter the threat landscape.
Current legislative efforts across the globe overwhelmingly focus on content-based and cultural concerns such as regulating AI-generated media, addressing bias, and managing misinformation. Although these issues deserve attention, they primarily represent extensions of existing problems rather than novel threats. AI-generated deepfakes, for instance, may be more convincing and easier to produce, but they exist on the same continuum as previously existing misinformation tactics. Our current legal and social frameworks, with modest adjustments, can largely address these concerns.
Privacy, however, presents an instructive case that helps demonstrate both the utility and limitations of our capability threshold framework. Unlike content moderation concerns, AI’s impact on privacy is not merely an intensification of existing problems—it represents something qualitatively different.
Traditional privacy regulation operates on a simple premise: Organizations must obtain consent before collecting certain data, and individuals can control what personal information they share. This model worked reasonably well in a world where data collection was explicit and direct.
Advanced AI fundamentally changes this equation. Consider three concrete examples:
First, AI systems can now infer highly sensitive information that was never explicitly shared. Research has shown that machine learning models can predict personal attributes and medical conditions from seemingly unrelated digital footprints, identifying markers of depression from language patterns, pregnancy from purchase history, or chronic illness from search queries before users themselves have shared this information publicly. These assessments are not just the results of more efficient data analysis; they are the creation of new personal data. These new data were not collected with consent.
Second, AI enables pattern recognition across disparate data sources that reveals private information no single dataset contains. When a retailer’s purchase records are analyzed alongside public transportation data and social media activity, AI can construct detailed profiles revealing information individuals specifically chose to withhold from any single company. This privacy violation occurs not in the data collection, which may be entirely legitimate, but in the inference.
Finally, AI’s predictive capabilities can anticipate future behaviors or life changes before individuals themselves are aware of them. Systems can predict with alarming accuracy when someone is likely to get sick or experience relationship difficulties—all without any direct disclosure of these matters. These predictions fundamentally undermine the concept of informed consent, as individuals cannot proactively agree to sharing information they do not yet know about themselves.
These capabilities represent a genuine threshold breach—not merely increasing breaches of existing privacy laws but fundamentally altering what can be known about individuals without their knowledge or consent. Traditional privacy frameworks built around notice and consent simply cannot address these new capabilities.
Yet, reasonable people might disagree about whether these developments constitute a true capability threshold or merely an intensification of existing privacy challenges. This ambiguity illustrates why our framework should serve as a useful guide rather than a rigid classification system. It helps direct regulatory attention to where existing frameworks are most clearly inadequate and allows for thoughtful debate in borderline cases.
Where AI demands an entirely new security paradigm is in areas where it creates capabilities that were previously impossible or limited to a small set of highly resourced actors.
Among the most urgent AI security threats is the democratization of bioweapon development capabilities. What was once the exclusive domain of advanced state programs is now potentially accessible to non-state actors through AI-guided design of pathogens. Recent advances in genetics may allow AI systems to, for example, identify novel pathogen variants with enhanced transmissibility or lethality, design synthetic organisms that evade existing detection systems, enable the optimization of production methods that require minimal specialized equipment, and, perhaps most concerning, help circumvent known countermeasures like vaccines or treatments.
These advances represent a true jump in capabilities: AI enables malicious actors without significant scientific training to potentially develop bioweapons of greater fatality rates and enhanced transmissibility while simultaneously lowering barriers to creation and diffusion. This capability transfer from state to non-state actors upends our security calculus. Whereas monitoring a state’s access to certain chemicals or technology is somewhat feasible, such oversight over all possible actors is impossible.
Even though cyber threats have existed for decades, AI enables a noticeable shift in capability. AI enables self-propagating systems that autonomously identify and exploit novel vulnerabilities. It creates adaptive attack methods that evolve in real-time to bypass defensive measures. These technologies facilitate coordinated multi-vector attacks beyond human operational capacity. Most alarmingly, they enable systems capable of identifying and targeting critical infrastructure vulnerabilities without human guidance. The difference is profound: Traditional cyber-attacks require more human direction, but AI-enabled systems could operate continuously at machine speed, potentially discovering novel attack vectors beyond human conception.
AI capabilities are shifting military and security paradigms. Advanced AI enables systems capable of independent target selection and engagement without human oversight. These systems allow for decentralized coordination of multiple autonomous systems operating as swarms. The technology creates new potential vulnerabilities in critical systems and across multiple vectors.
Perhaps most destabilizing, advanced AI grants relatively small actors the ability to deploy sophisticated autonomous systems against larger powers. The Houthis, for instance, have relied on drone swarms to attack ships sailing through key ports and straits. The key security concern is not merely more effective weapons, but the shift in who can deploy them and how they operate independent of human control.
The current legislative landscape is misaligned with these novel threats. Around the world, a primary focus has been on content regulation, such as requiring watermarking or labeling of AI-generated media; discrimination concerns, such as prohibiting uses of AI that might disadvantage protected groups; privacy protections, such as limiting data collection and processing by AI systems; and transparency requirements, such as mandating disclosures about AI use and capabilities.
Although these concerns are legitimate, they represent familiar regulatory territory. Existing frameworks for addressing deceptive media, discrimination, and privacy can be modestly adapted to address AI-enhanced versions of these challenges.
Meanwhile, the truly novel threats—those that represent genuine capability jumps—remain largely unaddressed by targeted regulatory frameworks. This mismatch creates dangerous security gaps while simultaneously risking overregulation in areas where existing frameworks could suffice.
An effective AI security framework must begin with the “threshold breach” principle of identifying and prioritizing threats where AI creates qualitatively new capabilities rather than merely intensifying existing challenges. There are multiple examples of potential interventions that would fit within that framework.
First, rather than regulate AI systems broadly, Congress should institute controls specific to the capabilities that present novel threats. Supervised access to biological design capabilities would require rigorous security protocols and monitoring for systems capable of protein folding prediction or genetic sequence optimization. Mandatory security testing for autonomous systems would implement red-team testing requirements specific to systems capable of autonomous operation. Compute restrictions for high-risk applications would apply hardware-level controls on systems demonstrating capabilities in sensitive domains.
Second, to address the unprecedented biosecurity challenges presented by AI capabilities, Congress should establish a comprehensive national biodefense modernization initiative that integrates advanced technological solutions with traditional biosecurity frameworks. This initiative would develop rapid pathogen identification systems capable of detecting engineered organisms. It would establish a classified database of potential AI-designed biological threats and countermeasures. The program would create surge capacity for rapid vaccine and treatment development against novel pathogens. In addition, it would implement continuous monitoring of open-source AI capabilities related to biological design.
Finally, Congress should authorize and fund a comprehensive critical infrastructure hardening program through dedicated legislation that provides both the resources and authorities necessary to defend essential systems against AI-enhanced threats. This program would implement air-gapped backup systems for critical infrastructure control systems. It would develop “AI-resilient” security protocols that are tailored to novel AI threats and, more generally, do not rely on predictable defense patterns. The initiative would create rapid-response protocols specifically for addressing autonomous cyber-attacks. It would also establish a classified threat intelligence sharing program between government and infrastructure operators.
A pivot to AI security offers another significant benefit: It removes unwarranted regulatory attention from what we might call “boring AI”—applications that may cause harms, but that are well addressed by other existing legal regimes. These include productivity tools, business analytics systems, educational tutors, health care scheduling applications, and other AI systems that present no novel threat vectors.
The current regulatory approach risks imposing a stifling regulatory fog over these technologies. This fog creates uncertainty that disproportionately harms small and mid-sized firms. Although large tech companies can afford compliance departments, regulatory consultants, and legal teams to navigate complex AI regulations, smaller innovators typically cannot. The compliance costs of broad-based AI regulation are thus anti-competitive, favoring large incumbents and creating barriers to entry for potential disruptors.
Anti-competitive results are particularly problematic because many of the most socially beneficial AI applications, such as personalized educational tools for underserved communities, specialized healthcare applications for rare conditions, or public sector efficiency improvements, often emerge from smaller, mission-driven organizations rather than large tech firms focused on mass-market applications. Regulatory uncertainty and compliance burdens may prevent these crucial innovations from reaching those who need them most.
By focusing regulatory attention specifically on novel capabilities that create new threats, we allow boring AI to develop under existing legal frameworks—consumer protection laws, anti-discrimination statutes, and, to a lesser extent, privacy regulations—without imposing additional AI-specific burdens. This approach ensures that we address genuine novel risks while allowing beneficial innovation to flourish, particularly among smaller entities developing specialized applications to solve public problems.
This security-focused approach is also far more politically viable in the current environment than content-based regulation for several reasons. First, there is a bipartisan consensus on national security, as protection against novel threats to American citizens and infrastructure enjoys broad support across political divides. Second, this approach emphasizes targeted rather than broad regulation, focusing on specific, high-risk capabilities rather than sweeping AI regulation, which aligns with a preference for limited government intervention. Third, this framework prioritizes strategic advantage preservation by maintaining America’s edge in AI capabilities while preventing adversaries from exploiting dangerous applications, which appeals to national security hawks. Finally, this framework ensures minimal impact on commercial innovation by targeting only the most dangerous capabilities, allowing continued development in low-risk domains.
The current Administration’s emphasis on American leadership and security makes this approach particularly appealing, as it addresses concrete threats rather than abstract cultural concerns that tend to divide along ideological lines.
By focusing regulatory attention on these specific novel threats rather than familiar cultural concerns, we can develop an AI security framework that is both effective at addressing genuine risks and politically viable in the current environment.
The window for establishing these targeted security measures is now, before malicious actors can exploit these novel capabilities. By prioritizing threats that cross critical security thresholds rather than mere intensifications of familiar problems, we can create a security framework that protects against the most dangerous aspects of AI while allowing continued innovation in beneficial applications. As privacy regulation demonstrates, however, this framework should be applied thoughtfully and with an awareness of its limitations.