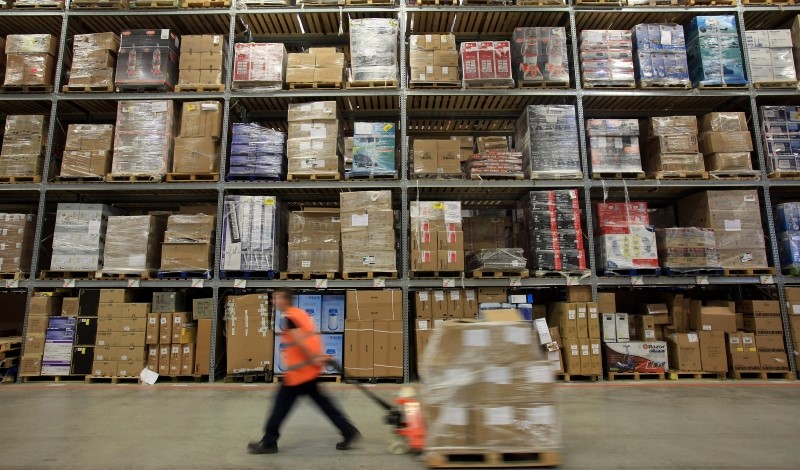
Scholars offer a new analytic approach to testing platforms for self-preferencing bias.
“Hey, Alexa. Will the FTC win its case against Amazon Marketplace?”
Even Alexa cannot answer this question—one that the Federal Trade Commission (FTC) will grapple with in its long-awaited case against Amazon Marketplace. In a recent complaint, the FTC alleges that Amazon Marketplace unfairly highlights its own products on its website to encourage consumers to select the Amazon brand over competitors’ products.
In a recent article, two researchers question this claim. Lukas Jürgensmeier and Bernd Skiera of Goethe University explain how evaluating the fairness of product placement on a retail platform is more complex than it might seem. Using an algorithm they designed, Jürgensmeier and Skiera argue that Amazon is likely not preferencing its products over other brands, contrary to the FTC’s claim. In one analysis, for example, Jürgensmeier and Skiera find that Amazon’s products are 50 percent less favored in search results than are comparable products by other brands.
To test whether a platform is providing a comprehensive recommendation to consumers, stakeholders have developed standards to assess “fairness”—that is, whether the recommendations a platform offers are unbiased and do not discriminate against certain groups.
Jürgensmeier and Skiera argue that there is no universal fairness standard due to differences across platforms. For instance, platforms can offer recommendations in various formats, such as product labeling, profile ordering, or curated lists. Platforms also use different product attributes to generate their recommendations. Jürgensmeier and Skiera assert that a single fairness standard would not be able to account for these diverse criteria.
Jürgensmeier and Skiera also argue that the context within which the stakeholder tests the platform influences the fairness standard. If a stakeholder is evaluating a platform under different regulatory frameworks, for example, they argue that a single fairness definition cannot account for the varying parameters used to determine the algorithm’s legality.
But Jürgensmeier and Skiera contend that it is challenging to accurately test a recommendation’s fairness without a workable fairness definition. And they highlight that existing evaluations of platform fairness do not allow researchers to use different fairness standards, making them inapplicable for many of the algorithms that people want to test.
To address the limitations of existing evaluations, Jürgensmeier and Skiera developed their own “context-specific” algorithm to test for biased recommendations on online retail platforms.
According to Jürgensmeier and Skiera, their algorithm addresses existing challenges because they can apply it to fit a variety of platforms and contexts. Despite the algorithm’s diverse use, the algorithm also provides a “clear criterion” for when to consider a recommendation fair and includes steps that account for differing product attributes and fairness definitions.
When they applied this algorithm to Amazon data, it found that Amazon’s own products receive a much lower degree of placement privilege over other brand’s products.
In one application of their algorithm, Jürgensmeier and Skiera analyzed whether Amazon’s own products showed up more often at the top of Amazon’s search results than did similar third-party substitutes. Jürgensmeier and Skiera found that Amazon’s products were 50 percent less favored in search results than comparable products by other brands, which suggests that Amazon’s search results likely do not favor Amazon’s own products over other brand’s products.
In another example, Jürgensmeier and Skiera focused on Amazon’s use of the “buy box,” which is similar to an “Add to Cart” feature that highlights one seller. Amazon uses an algorithm to determine the buy box seller. The algorithm awards the buy box to a product based on its competitive pricing, fast and free shipping, high quality customer service, and a high in-stock percentage. Jürgensmeier and Skiera state that these attributes are unprotected, meaning they are not protected by law and are not typically regarded as sensitive in fairness assessments.
The buy box is a feature with significant implications—industry experts estimate that the buy box seller receives close to 90 percent of product sales. Jürgensmeier and Skiera examined whether particular products were more visible in the search results on days when Amazon, rather than a third party, was featured in the buy box.
Jürgensmeier and Skiera found that, on average, Amazon branded products were more visible on days when Amazon products held the buy box. Over three best-selling products, Amazon branded products held the buy box 70.4 percent of the time. After controlling for the unprotected attributes, however, Jürgensmeier and Skiera concluded that Amazon did not unfairly favor its products.
Jürgensmeier and Skiera suggest that the disparity in visibility of Amazon’s products between the two examples may be a way for Amazon to skirt antitrust issues. They argue that, if Amazon were favoring its own products in the search results, this bias would be easier for regulators to detect, whereas favoritism in the buy box feature is less obvious.
Jürgensmeier and Skiera conclude that Amazon’s recommendation practices do not yield unfair results for third parties, based on their analysis, a result that contradicts suspicions voiced by the FTC. Jürgensmeier and Skiera offer their statistical approach as one way for regulators to test online platforms for compliance, given the limitations of existing analytical tools.
Should the court deciding the case between the FTC and Amazon choose to rely on a test similar to Jürgensmeier and Skiera’s, Alexa may have an answer to the question of which side will prevail after all.