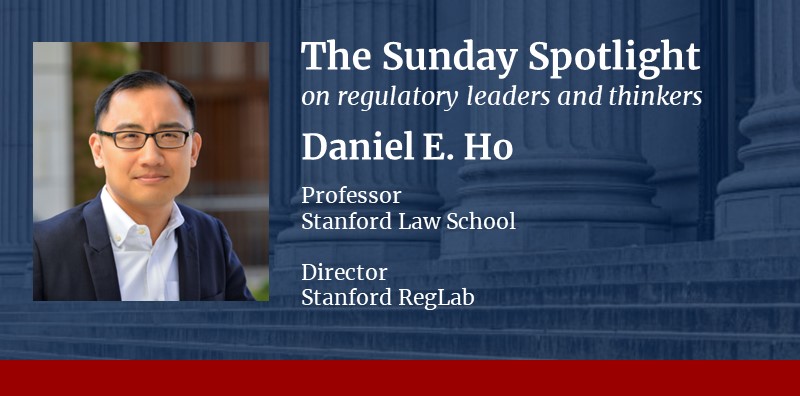
Daniel Ho discusses the importance of identifying emerging risks for effective artificial intelligence regulation.
In a recent discussion with The Regulatory Review, Daniel E. Ho, the William Benjamin Scott and Luna M. Scott Professor of Law at Stanford Law School, offers his thoughts on the future of artificial intelligence (AI) regulation in a time of rapidly changing and asymmetric information.
ChatGPT was nowhere near the first artificial intelligence model created. The public release of ChatGPT late last year, however, helped propel AI from a technical field of study of coders and computer scientists to an everyday topic of conversation. With companies rushing to offer their own tools to integrate AI into the lives of their users, important regulatory considerations about AI have emerged. Professor Ho helps shed light on some of the considerations facing creators, regulators, and users of artificial intelligence. Ho shares his concerns about identifying AI risks, his support of risk reporting and auditing mechanisms, and his recommendations for future legislative proposals.
In addition to teaching at Stanford Law, Ho serves on the National AI Advisory Committee, where he co-chairs the Working Group on Regulation and Executive Action that issued a guide to AI regulation. He serves as senior advisor on responsible AI to the U.S. Department of Labor and as a public member of the Administrative Conference of the United States. Ho was previously associate director of the Institute for Human-Centered AI at Stanford University.
Ho also directs the Stanford RegLab, which partners with government agencies on demonstration projects that use data science and machine learning to modernize governance. The partnership between RegLab and the Internal Revenue Service (IRS), for instance, developed AI approaches to audit tax returns more effectively and fairly. With U.S. Department of Treasury collaborators, RegLab developed a framework for equity assessments when demographic attributes were not directly observed. This framework identified racial disparities in legacy audit systems and led the IRS to overhaul its earned income tax credit auditing process.
Ho is also a professor of political science, professor of computer science (by courtesy), and a senior fellow at the Stanford Institute for Economic Policy Research.
The Regulatory Review is pleased to share the following interview with Professor Ho. All of his statements in this exchange are made in his individual capacity.
TRR: AI has been a major topic of conversation as of late, with corporations, law firms, educational institutions, and others preparing for the implementation of AI technologies in their operations. What do you see as the most pressing regulatory issue created by AI today?
Ho: The most pressing regulatory issue is developing a mechanism to understand emergent risks associated with AI. Currently, much of the dialogue is driven by speculative and anecdotal accounts of how high-capacity AI systems might pose risks to cybersecurity and national security. It will be critical for the government to build regulatory capacity to develop an informed understanding of these emergent risks, without relying on accounts by a small number of parties who may have very distinct interests.
TRR: Some members of Congress have sought to address the challenges of algorithmic accountability, including through the introduction of the Algorithmic Accountability Act of 2022. Can legislation solve the accountability challenges created by AI? How would you recommend Congress approach this issue?
Ho: As I noted above, the fundamental challenge is the information asymmetry between industry and government about emergent risks. When we talk about the risks of biological weapons or even existential risk from AI, policy should not be made based on anecdotes and fairy tales. To reduce that information asymmetry and produce timely information, I have increasingly favored mechanisms for adverse event reporting and auditing.
In other areas where the government needs to know about emergent risks—such as those from cybersecurity or dangerous pathogens—we have established mechanisms for mandated reporting and investigation of such incidents. Some claims of the risks associated with the use of large language models for biological weapons may turn out to be no more worrisome than the use of web searches. Other risks may turn out to be substantial. And the problem right now is that the government cannot easily distinguish hype from reality.
TRR: Is there anything that concerns you about existing legislative proposals?
Ho: When you look at the legislative proposals that have actually moved forward, I worry that Congress may be replicating the historical accident of the Privacy Act of 1974. In the wake of Watergate, concerns about surveillance led Congress to regulate what was easily within reach: federal agencies. That left much of the private sector alone, and the private sector, in turn, created the kinds of systems of records that may pose a much more substantial threat to privacy.
Today, proposals that have been easier to move forward are ones that put constraints on the public sector, less the private sector. Many of these proposals are salutary, as government systems make consequential decisions. But some may inadvertently tie up agencies with red tape and undercut the transformative potential of technology and AI for government. In my mind, public sector technology and AI regulation are inextricably intertwined, as they are both fundamentally about agency expertise and capacity. In an earlier evaluation of the federal government’s efforts to implement a key transparency initiative, for example, AI use case inventories, as mandated under Executive Order 13,960, bureaucratic capacity was alarmingly low. If agencies are so tied up in red tape that they cannot hire technologists, they will not be able to effectively regulate AI.
TRR: Looking forward, in what areas would you like to see further research that could inform future efforts to regulate AI?
Ho: First, I think we need to develop a better understanding of emergent risk. We need research that clearly identifies the marginal risk of AI, and specifically foundation models, relative to existing baselines.
Second, we will need to build improved collaborations across technical and legal domains to identify policies that are technically and institutionally feasible. For instance, too many legislative proposals anchor around explainability, but the science of explainability is still in its infancy. Conversely, many technical scholars will point toward government audits, which may not be feasible, or industry audits, which pose sharp conflicts of interest. We will need many more collaborations between technologists, social scientists, and lawyers.
Third, we should also be clear that the impetus for AI regulation, upon reflection, may actually militate toward non-AI regulation. The anxiety around biological weapons risk, for instance, may actually require strengthening oversight of brick-and-mortar laboratories. Similarly, it is not obvious why the environmental footprint of training foundation models should lead to a tax on training AI models, as opposed to a general carbon tax.
TRR: Thinking specifically about AI tools used by lawyers, should there be measures in place to ensure that AI is used ethically and responsibly in the legal profession? If so, what measures do you recommend?
Ho: As AI moves into new domains, safeguards will be critical. For the legal profession, I think it means, most importantly, that technology should assist legal decision making, not replace it. The ABA Resolution, which calls for human oversight and organizational accountability is a good step forward to that effect.
The Sunday Spotlight is a recurring feature of The Regulatory Review that periodically shares conversations with leaders and thinkers in the field of regulation and, in doing so, shines a light on important regulatory topics and ideas.