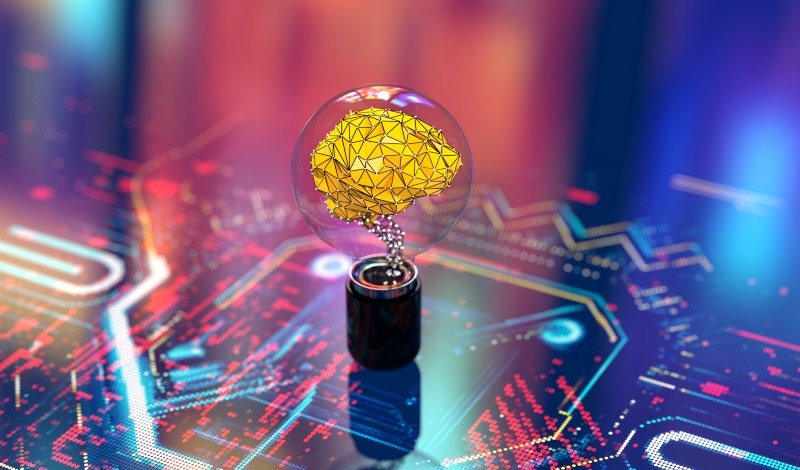
Experts recommend a two-pronged approach to help ensure artificial intelligence systems achieve equity.
The public conversation has increasingly focused on bias in artificial intelligence (AI) systems that can be traced to poorly representative datasets and the unconscious biases of programmers. In a recent paper, three experts propose a two-pronged approach—with both direct and indirect policy interventions—that aim to ensure that AI systems achieve equity.
These experts—Rangita de Silva de Alwis, a professor at the University of Pennsylvania Carey Law School, Amani Michelle Carter Coutinho, a lawyer at Kirkland & Ellis, and Govind Nagubandi, an independent data scientist—argue both for direct and indirect interventions to encourage changes in the design of machine learning as well as at the development stages of business models using AI technology.
As for direct intervention, de Silva de Alwis, Coutinho, and Nagubandi argue for the recognition of a fifth category of intellectual property rights alongside patents, copyright, trademark, and trade secrets. This new category would grant ownership interests in an algorithm to the extent it is developed lawfully, without implicit bias. The parameter for this evaluation would be the United Nations (UN) human rights treaties, especially the Guiding Principles on Business and Human Rights 2011—known as the Ruggie Principles.
Based on the norms of the UN human rights treaties, ownership of an algorithm would require that it possess six characteristics. These six characteristics are an inclusive policy statement, diverse input datasets, due diligence norms to mitigate negative outcomes, the possibility of user redress, and transparency through third-party audits.
As for indirect intervention, de Silva de Alwis, Coutinho, and Nagubandi propose a tax adjustment on the gains of venture capital firms and founding teams that have an optimal commitment statement to diversity and take robust steps to implement those commitments across a continuum.
They ground their recommendation for an indirect policy intervention to promote equity in AI in an analysis of the CrunchBase rank statistics. They point to a diversity gap in the data that reveal a link between the demographics of venture capital firms and startup founding teams and explain how, ultimately, this impacts the technology these firms develop.
“Venture capital firms’ demographics and startup founding teams do not reflect the broader U.S. population,” observe de Silva de Alwis, Coutinho, and Nagubandi.
After analyzing Crunchbase data from 2016 to 2020, they conclude that venture capital firms’ demographics present significant gender and race asymmetry.
According to data from 2016 to 2020, 80 percent of AI investors were male versus 20 percent female. As for ethnicity, Hispanic, and Black investors were underrepresented, with Black investors having the lowest representation at only 1 percent of the total of AI investors. The data showed clearly that white males were overrepresented among AI investors.
The demographic distribution of venture-funded startup teams was not much different during the same period of time. Although startup founder gender was not specified in Crunchbase rank statistics, white-led AI companies received 71 percent of venture capital funds, followed by Asians with 20 percent, Hispanics with 3 percent, and Black individuals with 1 percent.
De Silva de Alwis, Coutinho, and Nagubandi also analyze data related to investments in the early stages of AI companies from years 2016 to 2020. Overall, investments increased 20 percent in this period. In seed rounds, companies with white leadership positions accounted for 50 percent to 58 percent of investments, while firms with Asian leaders received only 7 percent to 13 percent of funds, with other ethnicities receiving 31 percent to 41 percent. In series A and B rounds, the unequal distribution of investments was even greater.
“The fact that venture capital firms and funding teams are out of the sync with the US population is relevant because diverse teams can increase the likelihood the AI they build will be equitable,” state de Silva de Alwis, Coutinho, and Nagubandi.
They propose the creation of a commitment index to determine if a company qualifies for tax reduction as a tool to promote indirect intervention toward equitable AI.
De Silva de Alwis, Coutinho, and Nagubandi clarify that no “one size fits all approach” exists for what to include in this commitment index. They suggest that different indicators should be valued in the index, whether promoting diversity on companies’ board and management teams, recommending executives and advisors to companies with diverse founders, creating funds to sponsor minority talents, and making donations to diversity and inclusion projects.
De Silva de Alwis, Coutinho, and Nagubandi’s two-pronged approach toward equitable AI—with its new form of intellectual property protection combined with tax adjustments for venture capital firms that are committed to diversity—aims at the entire AI ecosystem. Because their proposal is not limited simply to regulating AI, but encompasses managerial principles of environmental, social, and governance business models, they argue that it holds the promise of bringing equity to the digital infrastructure of the future.