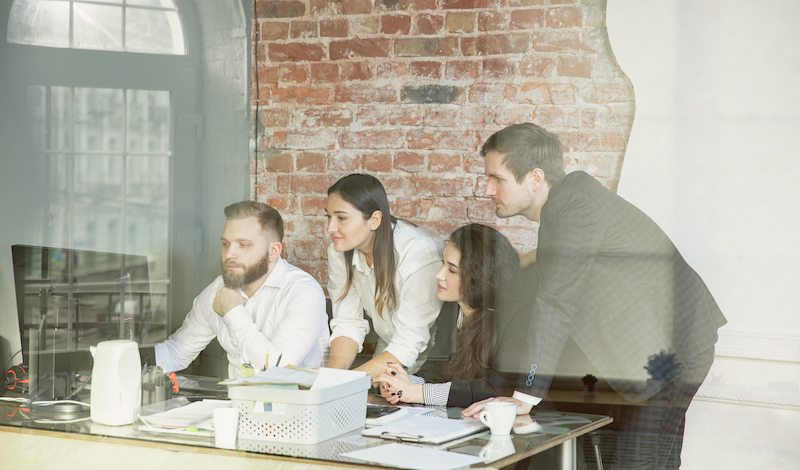
In deciding to use artificial intelligence, the key question for administrators is a comparative one.
Algorithms, at their most basic level, consist of a series of steps used to reach a result. Cooking recipes are algorithms, mathematical equations are algorithms, and computer programs are algorithms.
Today, advanced machine-learning algorithms can process large volumes of data and generate highly accurate predictions. They increasingly drive internet search engines, retail marketing campaigns, weather forecasts, and precision medicine. Government agencies have even begun to adopt machine-learning algorithms for adjudicatory and enforcement actions to enhance public administration.
These uses have also garnered considerable criticism. Critics of machine-learning algorithms have suggested that they are highly complex, inscrutable, prone to bias, and unaccountable. But any realistic assessment of these digital algorithms must acknowledge that government already relies on algorithms of arguably greater complexity and potential for abuse: those that undergird human decision-making. The algorithms underlying human decision-making are already highly complex, inscrutable, prone to bias, and too often unaccountable.
What are these human algorithms? The human brain itself operates through complex neural networks that have inspired developments in machine-learning algorithms. And when humans make collective decisions, especially in government, they operate via algorithms too—many reflected in legislative, judicial, and administrative procedures.
But these human algorithms can often fail. On an individual level, human decision-making falls prey to a multitude of limitations: memory failures, fatigue, availability bias, confirmation bias, and implicit racial and gender biases, among others. For example, working human memory can process only a handful of different variables at any given time.
On an organizational level, humans are prone to groupthink and social loafing, along with other dysfunctionalities. Recent governmental failures—from inadequate responses to COVID-19 to a rushed exit of U.S. forces from Afghanistan—join a long list of other group decision-making failures throughout history—such as the Bay of Pigs crisis and the Challenger Shuttle explosion. One researcher has even estimated that approximately half of all organizational decisions end up in failure.
For these reasons, human decision-making may well be even more prone to suboptimal and inconsistent decisions than their machine-learning counterparts—at least for a nontrivial set of important tasks.
Government agencies ought to aspire for speed, accuracy, and consistency in implementing all their policies and programs. And machine-learning algorithms and automated systems promise to deliver the greater capacity needed for making decisions that are more accurate, consistent, and prompt. As a result, digital algorithms could clear backlogs and reduce delays that arise when governmental decisions depend on human decision-makers. The Internal Revenue Service, for example, has estimated in the first quarter of 2022 that it still faces a backlog in processing as many as 35 million tax returns from 2021—a problem that it is seeking to solve by hiring up to 10,000 more employees to sift through paperwork.
Compared against the evident limitations in human-driven governmental performance, not to mention low levels of public confidence in current governmental systems, machine-learning algorithms appear to be particularly attractive substitutes. They can process large amounts of data to yield surprisingly accurate decisions. Given the high volume of data available to governments, in principle administrators could use these algorithms in a wide range of settings—thereby helping to overcome existing constraints on personnel and budget resources.
In the future, government agencies must choose between maintaining a status quo driven by human algorithms and moving toward a potentially more promising future that relies on digital algorithms.
To ensure that governments can make smarter decisions about when to rely on digital algorithms to automate administrative tasks, public officials must first consider whether a particular use of digital algorithms would likely satisfy basic preconditions needed for these algorithms to succeed. The goals for algorithmic tools need to be clear, such that the social objectives of the contemplated task, and the algorithm’s performance in completing it, can be specified precisely. Administrators must also have relevant and up-to-date data available to support rigorous algorithmic analysis.
But beyond just seeing if these preconditions are met, it will also be important for government decision-makers to validate machine-learning algorithms and other digital systems to ensure that they indeed make improvements over the status quo. They also need to give serious consideration to risks that might be associated with digital algorithms. Administrators must also ensure adequate planning for accountability, proper procurement of private contractor services, and appropriate opportunities for public participation in the design, development, and ongoing oversight of digital algorithmic tools.
Ultimately, when evaluating machine learning in governmental settings, public administrators must act carefully and thoughtfully. But they need not feel that they must guarantee that digital systems will be perfect. Any anticipated shortcomings of artificial intelligence in the public sector must be placed in proper perspective—with digital algorithms compared side-by-side with existing processes that are based on less-than-perfect human algorithms.
This essay draws on the authors’ article, Algorithm vs. Algorithm, in the Duke Law Journal. The opinions set forth in this essay are solely those of the authors and do not represent the views of any other person or institution.