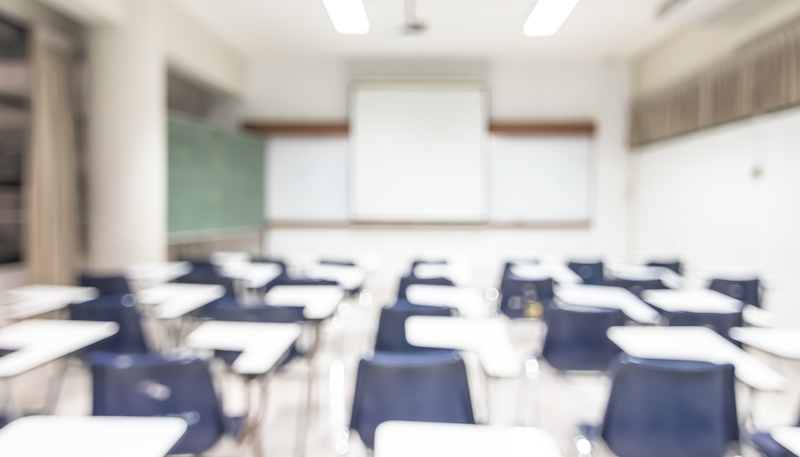
A groundbreaking algorithm designed to optimize school start times meets fierce community resistance.
Editor’s note: This essay is part two of a two-part feature on algorithmic governance.
After the Boston public school system decided in 2017 to redesign the city’s school bus schedule using algorithms, it held an open transportation challenge inviting computer scientists to develop the new system. The “Quantum Team” from the Massachusetts Institute of Technology (MIT) Operations Research Center won both phases of the challenge. Professor Dimitris Bertsimas, co-director of the Operations Research Center, along with PhD students Arthur Delarue and Sébastien Martin, would build the model. With respect to the bus routing, they solved what is known as the “traveling salesman problem” of mapping the most efficient way to cover a route without doubling back, while also accounting for road surfaces, traffic, and many other variables. Using public data and data from the school district, they designed an algorithm to reduce the number and footprint of bus routes, reconfigure bus stops, maximize the number of passengers on each bus, and reduce the use of empty buses.
In addition to delivering cost savings, the system would reduce bus travel, eliminating some 20,000 pounds of carbon emissions per day and cutting up to 1 million bus miles per year. The algorithm factored in the needs of wheelchair-friendly buses for disabled students, as well as those students who required home pick-ups. The new bell schedule optimized for later school start times for high school students. The model also optimized for equity in accordance with the school district’s guidelines to reduce the disparity between white and minority students with respect to start times.
David Scharfenberg of the Boston Globe called the algorithm “a marvel” that sorted through “1 novemtrigintillion options—that’s 1 followed by 120 zeroes.” It made these computations in approximately thirty minutes, as opposed to the grueling multi-week undertaking that had been typical.
This marvelous machine enacted significant change. To achieve the school district’s equity and health objectives, system officials reported that “the majority of schools and students must change their bell times, and some must shift earlier or later by two hours or more.”
In fact, the bell schedule algorithm shifted 84 percent of school start times to commence high school later and end elementary school earlier for more students. In conducting a disparate impact analysis, Boston Public Schools officials found that the MIT plan managed to distribute advantageous start times equally across major racial and ethnic groups, while substantially improving them for students in all of those groups. Under the status quo, white students were the only group with a plurality that enjoyed the desirable 8 to 9 a.m. start time, and that plurality was only 39 percent. Under the MIT plan, a majority of all students would start in the 8 to 9 a.m. slot, and that benefit was spread almost exactly equally across major racial and ethnic groups, with around 54 percent of students in each group enjoying it.
In the months following the transportation challenge, the MIT team continued to work closely with the city and participated in the school district’s ongoing community engagement process, making adjustments in response to feedback. In the end, the consultation process touched about 10,000 students, family members, and staff through 17 community meetings and other outreach.
This process sounds like a lot of outreach. It is perhaps for that reason that the MIT team was not prepared for the backlash that ensued when families saw how the changes would affect them. The consultations had not effectively described how the start time shifts in particular would change students’ schedules and they had not given families opportunities to play with the model.
Politics killed the algorithm. In December 2017, the Boston Public Schools voted in principle to adopt an algorithmically devised bus route and bell schedule. The new school schedule was released in December 2017, only nine months before it was due to be implemented in the fall of 2018.
The public pushback was strong and swift. Some parents who perhaps in principle accepted earlier start times for elementary school students were confronted with dramatic shifts from 9:30 a.m. to 7:15 a.m. Families with children of different ages suddenly found themselves facing a 90-minute launch time for their kids and juggling as many as three different bus schedules. Parents argued that the new schedule would deprive students of sleep, force families to spend more money on after-school programs, and “tear apart families.” Others complained that their high school kids, because of later start times, would now end school too late for work or extracurricular activities and that the work schedules of parents would be severely disrupted.
The disgruntled parents quickly generated an online petition, which garnered over 8,000 signatures, and then they protested at an “emotionally charged” school committee chamber, turning the plan into one of “the biggest crises of Mayor Marty Walsh’s tenure.” Parents at the school committee meeting carried signs reading, “Families over algorithms,” “Students are not widgets,” and “Stop the Lies.” Describing the situation as “an absolute disaster for Boston families,” one commenter argued that although algorithms can help “a shipping company move packages efficiently,” they are not as useful when it comes to figuring out how to move children.
Some 80 parents, students, politicians, and other interested parties testified against the new schedule. Protestors drowned out then-superintendent Tommy Chang’s attempts at reassurance. Five city councilors sent a letter to the school committee urging committee members to delay changes and formulate a better plan.
At the school committee meeting where protesters massed, MIT team members noticed that “most of the critics hailed from wealthier sections of the city.” From photos of the protests, it appears that most were white. But representatives of the minority communities—who were supposedly helped by the more equitable distribution of start times—were also opposed to the changes. The National Association for the Advancement of Colored People and the Lawyers’ Committee for Civil Rights and Economic Justice ended up opposing the plan on the ground that lower income households, having less flexibility to begin with, would find it difficult to adjust to the proposed schedule.
The algorithmic process itself became a scapegoat for community rage about both substantive change and its suddenness. More explanation about the tradeoffs, including visuals and tools enabling people to themselves play with the model, might have helped. This is the view of MIT Professor Joichi Ito, who noted,
While I’m not sure privileged families would give up their good start times to help poor families voluntarily, I think that if people had understood what the algorithm was optimizing for—sleep health of high school kids, getting elementary school kids home before dark, supporting kids with special needs, lowering costs, and increasing equity overall—they would agree that the new schedule was, on the whole, better than the previous one.
It is also possible that the proposed change, no matter how explained, was simply unacceptable to too many affected families. One of the benefits of algorithmic policy is that it is relatively easy to tweak. Had the MIT team been tasked with re-running the start time program, privileging stability to a greater degree, or doing so especially for families with children in different schools, they might have been able to produce a plan with more buy-in.
Boston quickly abandoned the project of changing school start times. The city’s school superintendent eventually resigned. The district did adopt the algorithmically derived bus routes, eliminating close to 50 superfluous bus routes and saving the district an estimated $3 million to $5 million per year. But when it walked away from the start time changes, it sacrificed a more equitable bell schedule and up to $15 million in savings. The proposed bell schedule changes were radical and the rollout did not provide a process for public input and change. The protesting public blamed the algorithm—which could have been tuned to optimize for other objectives—for failures of political process.
It is probably inevitable that the “losers” of what is a redistributive process will object to change no matter how it is implemented or explained. The benefit of a data-driven model is that it can be tuned to optimize different objectives. Tradeoffs can be represented graphically. Tools can be built to allow people to interrogate the model and scenario play. Had stakeholders been engaged not only before release of the model, but also iteratively with the model in beta form, they might have prioritized stability over change. In this case, more stability would have meant less equity.
Another piece missing from Boston’s algorithmic process was any added measure of due process for families unhappy with the results. Algorithmic generation of bus routes confers the authority of the machine on policy outcomes. “The machine says so” can be a powerful source of legitimacy. But it can also be a rebuke to the individual seeking flexibility or explanation. A model that can analyze 1 novemtrigintillion options in thirty minutes can also perhaps be instrumented with a way to represent how changing individual or neighborhood outputs can impact the system and the tradeoff curves. Such a “due process tool” was not part of the school district’s transportation challenge, so there is no reason the winning team would have built one.
Procurement of algorithmic processes, whether by contest or otherwise, should have explanatory and due process components. Citizens may be able to accept algorithmic policy losses if they have more purchase on the rationales behind them and recourse to change them.
Part one of this feature appears as “Defining Equity in Algorithmic Change.” To download both parts of this extended essay together, click here.
The author thanks Timon Cline for research assistance.