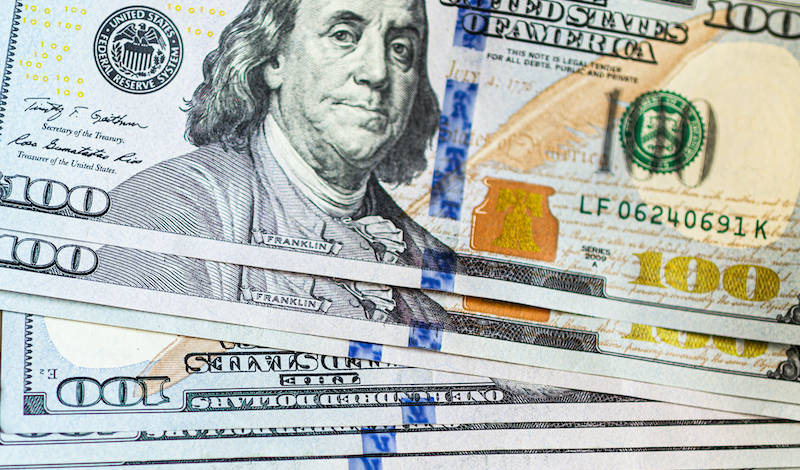
Streamlining administration of cash aid requires substantial legal and regulatory reform.
During a lull in the COVID-19 pandemic in 2021, hockey fans in South Dakota witnessed the halftime spectacle of 10 teachers scrambling to grab as many dollar bills from a pile of $5,000 to buy extra equipment for their classrooms.
Observers criticized the organizers for manifesting a real-life Squid Game—a television drama in which debt-ridden contestants risk their lives for money—and for exploiting essential public workers who were already risking their health to educate the nation’s children. Although the event sponsors foresaw enough physical contact to provide the competitors with helmets, they publicly apologized for their failure to predict the psychologically “degrading” impact of what they had intended to be “a positive and fun experience.” But they could be forgiven for the oversight. Unlike the event sponsors, the United States is not known for rewarding people with cold, hard cash, and certainly not without strings attached.
The United States’ reputation for providing public benefits in the most stingy and stigmatizing fashion stems from longstanding political choices aimed at eradicating dependency, even if it means abandoning the most desperate in society. Senior fellow at the American Enterprise Institute, Angela Rachidi, explains that cash aid should be “targeted to those with low incomes, time limited, and not erode work incentives in the long term.” And as journalist Bryce Covert also observes, even when society decides to give out cash—including during a pandemic emergency—policymakers make it impossible for many to receive it.
Public benefits agencies impose onerous and repetitive eligibility verification procedures and rely on outdated, clunky, and inaccessible computer technology. Moreover, policymakers require in-person visits to overcrowded, understaffed government offices to receive cash assistance. Basic subsistence becomes a game of economic regulation that those in need of cash aid cannot win.
Such thickets of red tape only compound the harms from pervasive government surveillance, police violence, and divestment in disproportionately low-income Black, Indigenous and people of color (BIPOC) neighborhoods and households. The resulting lack of trust that BIPOC communities hold for big government may reflect either an intuition or a cold calculation that the disproportionate costs of government intervention outweigh the benefits. No surprise, then, that Black conservatives criticize excessive regulation that purportedly holds Black people back from economic advancement. On this view, big government is not just a killjoy but a counterproductive damper on Black economic prosperity.
Now that COVID-19 and its harsh impacts are becoming endemic, policymakers are forced to rethink the processes and results of regulating private economic activities that fund public goods, such as infrastructure and healthcare. If politicians ever agree to extend pandemic public benefits beyond emergency use, there must be a better way to administer them.
Policymakers should perhaps not return to the old cost-benefit analysis many critics love to hate. But some commentators argue that tinkering with the existing cost-benefit framework can yield better results for less resources. A Biden Administration Memorandum on “Modernizing Regulatory Review” mandates federal agencies to consider racial and other forms of equity to offset miscalculations from discounting certain costs, such as a regulation’s cost to future generations and other costs that are hard to quantify. Instead, a kinder, gentler form of modified cost-benefit analysis—or as some scholars prefer, “benefit-cost analysis”—could provide more accurate, just, and economical methods to achieve regulatory ends.
One such method is to employ automated decision-making systems to reduce bureaucracy and human error. But that only does so much. Simplifying public benefits administration by streamlining the application process, for example, cannot occur without also increasing access to technology—such as broadband—and increasing food supply or cash assistance to match the needs of those found eligible.
Perhaps human-trained, machine-learning artificial intelligence can do more than just run code but also solve human problems with the benefit of the “legal intuition” of trained professionals. By detecting trends and identifying patterns in large tranches of data, “data scientists and experienced lawyers” can combine the best resources to get better results faster and cheaper. For example, humans have cracked the tax code by using machine learning to quantify business risk and identify advantageous tax planning and approaches.
But can automated “lawgorithms,” a term coined by Michele Gilman, do better than humans without introducing new errors or compounding existing ones? And can the lawgorithms discern what is missing in a data set, and why that data may not be included?
Society cannot rely on analytics or artificial intelligence to do all the thinking or prioritizing. Although current conversations are focused less on personal fault or failure and more on behavioral incentives and cognitive bias research, policymakers still cannot agree on what the research means. Greater attention to race strikes some observers as unconstitutional bean-counting or, worse still, akin to making everyone a prisoner of their race. Likewise, economic policy wonks may incorrectly blame inflation on hard-won expansion of labor protections instead of a more general “non-nuanced anxiety” sweeping the country as communities struggle to find their footing in the global pandemic.
Research centers spend money and time rationalizing the decisions of experts, but that money and time could provide public benefits simply because it is the right thing to do, regardless of the benefit-cost calculus. Even benefit-cost analysis assumes the existence of a basic safety net.
Indeed, policymakers may be more prone to making mistakes when regulating public benefits because economic justice concerns are invisible in legal and social institutions. For instance, legal scholar Julie A. Nice explains that “meaningful concern about the plight of poor people specifically, and about economic justice generally, is virtually unintelligible within American law.”
We need legal and social reforms to add humanity back into regulation. As humans who supply the principles on which machine learning builds, humans must also diversify the sources of information, analysis, and public voices that machine learning depends on. Only then can society tackle economic injustice instead of fighting over scraps.
This essay is part of a six-part series entitled Promoting Economic Justice.