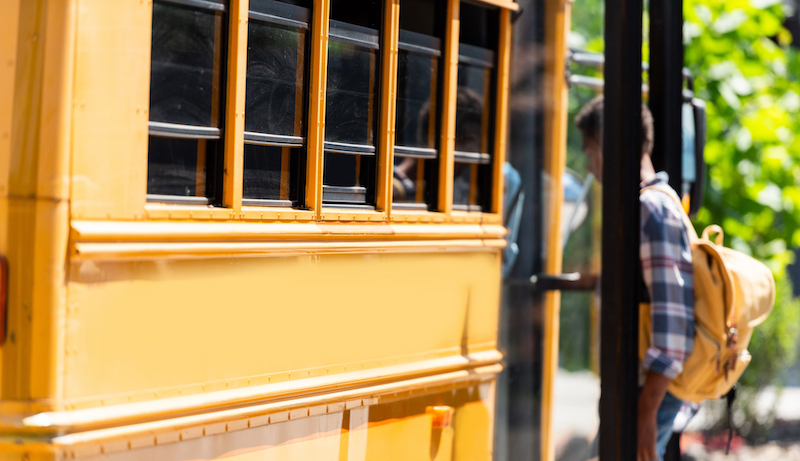
When Boston school leaders sought to use algorithms to redesign the bus schedule, equity remained a challenge.
Iconic yellow public school buses pass through streets all across the United States every day, performing a complex choreography of crisscrossing routes and school handoffs. It turns out that school districts have only very recently acquired the computational heft to optimally design bus traffic.
Algorithms and big data have now made it possible to redo public school busing in ways that cut costs, improve the environment, and better serve students, teachers, and parents. Taking advantage of these tools, the Boston public school system proposed an overhaul of bus routes and school schedules for the 2018 to 2019 school year with the possibility of saving up to $15 million per year.
What happened next should teach public officials everywhere—especially in regulatory agencies—something about how algorithmically derived policy can go sideways even when it promises greater efficiency and equity.
Algorithms are useful in solving any complex regulatory problem with a difficult computational component, such as a carbon tax, congestion pricing, pollution allowances, dynamic zoning codes. But there is a growing literature on the things we might worry about in the model that makes up the algorithm, including unfairness, opacity, and a lack of due process. These issues have been easiest to see where human life and liberty are on the line, as in policing and sentencing by algorithm. But more technocratic forms of regulation, like congestion pricing, can also raise pressing public interest questions. An algorithm tuned to maximize efficient traffic flows may result in inequitable or disruptively unpredictable costs for drivers. Even if equity is factored in, members of the public may resist because they do not understand the systems or prefer other tradeoffs.
Although Boston Public Schools adopted an algorithmic solution to bus routing, it rejected the more ambitious algorithmically generated changes to school start times after the public revolted against change that was too much, too fast. The overhaul was introduced with insufficient explanation or opportunity for citizen interaction with the model. With its demise, the school system forfeited up to $15 million in annual savings, better health for high school students starting school well before 8 a.m., and a more equitable distribution of the most desirable schedules.
Of all the concerns raised about algorithmic systems, scholars have focused most closely on the these systems’ potential for bias. In the case of the paired algorithms prepared for the Boston schools, the developers recognized the dangers of building a new system that perpetuated the inequalities of the old: Minority and poorer families on average got the less desirable school schedules. The developers took pains to design the new model to redress systemic bias. And yet the public, including representatives of the historically disadvantaged groups the algorithm was intended to benefit, roundly denounced the changes to school start times. The school district’s experience became a story about algorithmic scapegoating, in which opposition to comprehensive and disruptive change focused on the computational agent of that change. Press reports said the algorithm had “flopped.”
As significant as the risks are of biased algorithms, there is also a risk that public backlash and opaque implementations will burn trust where these technologies could do good. The failure in Boston to roll out improved bus routes paired with school start times shows that good science is not enough to overcome bad politics. In Boston, the school system made significant efforts to engage the public in what they wanted out of bus transportation and school starts in the abstract. But there was almost no engagement with the model itself, insufficient transparency about the algorithm’s tradeoffs, and no opportunity to adjust it.
The Boston public school system spends more than 10 percent of its budget on busing kids to and from school. In 2016, the schools spent $120 million to bus nearly 30,000 students to about 230 schools on 650 buses. The annual cost per student, which is just below $2,000, is the second highest in the United States and more than five times the average of the largest public school districts. Reducing the costs of public school busing by optimizing routes and fleets can allow districts to roll those funds off the street and into education.
Furthermore, when new bus routes are paired with new school schedules, the twin changes can improve student health and safety by creating later starts for teenagers and earlier dismissals for grade schoolers. Early school starts have been linked to serious teenage health issues, such as decreased cognitive ability and increased obesity and depression while late school starts for elementary school children mean that younger children have to travel home in the dark.
At the same time, changing bus routes and schedules can be hugely disruptive. More than half of all American K–12 students use public school buses for transportation to and from school every day, and, naturally, their families build work and childcare schedules around the bus times. Making any significant change district-wide requires balancing “competing objectives” and values, such as student health, special education programs, extracurricular activities, and student, parent, and staff schedules.
This balancing raises difficult computational challenges. Most school districts use handmade, ad hoc solutions to manage their bus fleets and have only been able to tackle this inherently systemic task in piecemeal fashion. Boston’s approach in the past included staggering the start and end times of different schools so that buses could make multiple trips throughout the day. The result was that there was a high variance of school start times, with the burden of the earliest start times falling on high school students, often before 8 a.m. Not surprisingly, the wealthier and whiter schools in the district have benefitted from later start times, with poorer and minority households disproportionately burdened by earlier ones.
More than two generations of Boston kids have passed through the school system since the city last restructured start times in 1990. In late 2016, the Boston public school system began the process of making significant improvements to its bus routes and school start times throughout the district by initiating a public engagement process aimed at discerning community values. Through this process, the district identified two overarching goals: “create an algorithm that will optimally route all of our buses, producing efficient routes and improving on-time performance,” and “create a series of bell times that are equitably and efficiently balanced, reducing transportation costs and accommodating community feedback.”
The school district wanted a combination of equity and efficiency. But what does equity mean in this context? Should the less popular earlier start times be equally distributed among demographic groups? Or should disruption from the status quo be distributed equally? Or should start times be distributed in a way that takes into account differential needs for earlier or later start times?
The Boston public school district recognized in an equity impact statement that change might be especially hard for lower income families, implicitly acknowledging the tradeoff between short-term disruption and longer-term benefits. Quantifying these tradeoffs may be hard or even impossible, so it is not surprising they were not visually represented or communicated effectively by school officials. Nor is it surprising that these officials did not get to the bottom of contesting conceptions of equity, which are a central problem to be worked out in the implementation of algorithmic systems.
In addition to these top-level goals, there were subsidiary objectives to increase the number of high school students starting school after 8 a.m., decrease the number of elementary school students dismissed after 4 p.m., accommodate special needs students, and reduce the cost of busing generally. In its public presentation to the Boston Schools Committee, school administrators reported that these subsidiary goals had won out against other possible contenders, including “minimizing overall change in the system.”
Obviously, there would be a tradeoff between stability and change. Given that the baseline distribution of school start times was inequitable, a move towards maximal equity might entail maximal disruption. Sacrificing some equity for stability would be one tradeoff that the public—including people who stood to gain from change in the longer term—might endorse. Ultimately, a phase-in of the plan, a more compelling explanation of why any model privileging equity and efficiency might entail significant disruption, and a better explanation of the equity story might all have helped to smooth acceptance of what was to come.
The Boston public school district decided that, rather than go through traditional procurement, it would solicit developers through a contest. In the spring of 2017, the school district announced a hackathon-style competition called the Boston Public Schools Transportation Challenge, consisting of two phases with $15,000 in prize money for both a bus routing program and bell schedule program optimizing the articulated goals. Notably, the challenge was entirely technical. Competitors were tasked with building an algorithmic system but nothing in the challenge concerned presentation, communication, or tools that would allow the public to interrogate the model. That absence of emphasis on the socio-political implementation of the system would prove decisive in the controversy to follow.
This essay is the first part of a two-part feature by Professor Goodman on algorithmic governance. Part two appears as “Smart Algorithmic Change Requires Collaborative Political Process.” To download both parts of this extended essay together, click here.
The author thanks Timon Cline for research assistance.