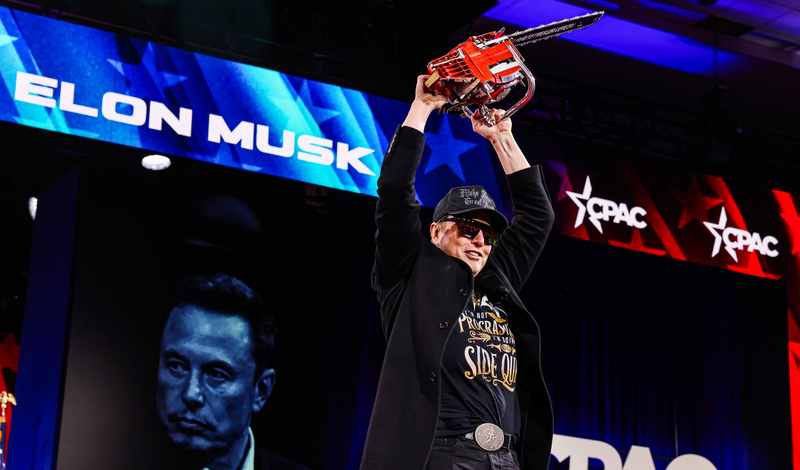
The Trump Administration’s mad rush toward AI automation of the administrative state will not succeed.
The federal administrative state faces its greatest upheaval since the Roosevelt Administration. The figure most associated with these sweeping changes, Elon Musk, claims that a huge proportion of the federal workforce can be painlessly automated through the aggressive deployment of artificial intelligence (AI) systems.
Although the current pace of change is unprecedented, the idea of using AI to boost the efficiency of the federal workforce has been around for at least a decade. A lack of technical expertise bedeviled early administrative agency experiments with AI. Today, as agencies shed a generation’s worth of human subject-matter expertise in favor of automated systems, the opposite problem looms.
Expanding the use of AI in regulatory enforcement carries natural tradeoffs. Given constant demands for agencies to regulate more entities without corresponding growth in agency resources, it is easy to see the appeal of increasing integration of algorithmic tools into administrative functions. Growing sophistication among targets of regulation may also necessitate relying on the processing power that only advanced algorithms can provide.
But AI may undermine the values—such as public trust, due process, expertise, and, not least, human character—underlying the unique role of administrative agencies in America’s constitutional system. Examples abound of automated decision-making systems in the public sector performing poorly or otherwise returning biased or erroneous results.
Beyond individual problematic use cases, the far more concerning impact is structural: the potential of AI systems to erode public trust in agencies and belief in their legitimacy.
Previous administrations—recognizing the fragility of public trust in the government and the critical nature of many automatable government functions—emphasized the need for innovation and experimentation while maintaining reasonable safeguards against catastrophic loss. For example, in March 2024, the Office of Management and Budget (OMB) issued an AI policy memo including recommendations and requirements such as tracking and publicly reporting agency AI use and identifying “rights-impacting” or “safety-impacting” AI use cases.
Moreover, over the last decade, governments worldwide—including those in the European Union, Singapore, and Canada—have developed robust standards to assess the suitability and performance of algorithmic decision-making systems. Domestically, the National Institute of Standards and Technology’s 2024 Artificial Intelligence Risk Management Framework provides a model assessment process for agencies to map potential risks, develop tracking mechanisms, and respond appropriately.
But there is no indication that the Trump Administration has incorporated existing domestic or international standards in its dash to remake the federal workforce. On his first day in office, President Donald J. Trump repealed a Biden-era executive order that required agencies to implement standardized AI safety evaluations, including for high-risk applications. On April 3, OMB issued a memo that replaced its 2024 policy memo. The new memo maintains elements of the earlier framework, such as requiring agencies to appoint “Chief AI Officers” and introduce management processes for “high-impact” uses, but it employs much more aggressive language focused on speed and eliminating “bureaucratic bottlenecks” to AI implementation.
This tonal shift suggests the Trump Administration may be more inclined to waive risk management requirements when inconvenient. More concretely, the new memo defers the deadline for the implementation of minimum safeguards to next year.
Concerns about the good faith of the Trump Administration illustrate a pervasive problem with the dominant, risk-based model of AI regulation. Although risk assessment approaches offer the advantages of familiarity, building on traditional models of assessments targeted at environmental or privacy harms also leads to a number of recognized weaknesses—including difficulties in addressing unpredictable, not easily quantified, or individualized harms. Moreover, effective risk assessment depends on agencies’ good faith implementation and use of oversight systems.
These weaknesses lead to calls for “epistemic humility,” a recognition of the limits of what regulators can know or predict when deploying AI systems, especially in sensitive or high-impact use cases. Thus far, the Trump Administration shows no signs of exercising any humility or caution when it comes to its planned overhaul of federal agencies.
Likewise, in connection with its use of AI tools to date, the Trump Administration has taken no steps to introduce robust public engagement processes—a key hallmark of a strong oversight system. At a minimum, effective public oversight requires robust transparency, such as the publication of AI risk or impact assessment results, and meaningful opportunities for public feedback before irreversible changes.
The speed and scale of AI implementation across the federal government make an appropriate system for assessing performance failures by AI tools vital if the government is to forestall incipient problems, including potential cascading failures if these tools do not perform as advertised. Monitoring and evaluation systems are of paramount importance given this Administration’s apparent neglect of the safeguards intended to guide initial policymaking.
This approach presents its own obstacles. Common tendencies to over-estimate AI capabilities and to avoid perceived sunk costs make deploying effective assessment mechanisms challenging even under ideal conditions. In an agency that has lost significant personnel and expertise due to mass layoffs, effective oversight will present still greater difficulties.
Agencies that increasingly automate key functions should also incorporate retirement strategies for AI systems into their assessment and planning. A court decision holding unlawful an agency enforcement action based on an unreliable or otherwise problematic AI-enabled component could taint years of enforcement relying on the same AI-based operational paradigm. Likewise, if agencies ignore problems until public outrage or press leaks force their hands, the loss of public trust and legitimacy could be catastrophic.
The administrative state reflects the American people and American values. Questions of fairness, bias, and due process demand a human element in official decision-making. Although the Trump Administration appears to be charting its own path to greater automation, existing standards developed to guide responsible innovation in the public sector are a vital framework to assess these changes—and, hopefully, will help the next generation of federal employees salvage their agencies character, values, and core mission from the aftermath of this Administration’s mad rush toward automation.