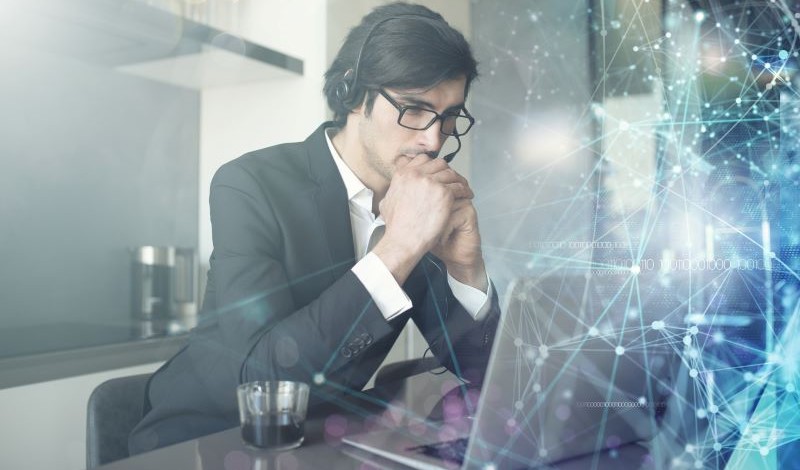
Scholars argue for the creation of a regulatory safe space to supplement AI regulations.
Artificial intelligence (AI) promises vast economic and social benefits, but also poses distinct dangers to the public. This friction presents regulators with a difficult challenge. Failing to regulate can exacerbate AI’s threats to privacy and public safety but regulating too strictly may prevent the public from benefiting from AI innovations.
In a recent article, Jon Truby of Qatar University and several coauthors warn that regulatory safe spaces—or sandboxes, as they are sometimes called—may not appropriately balance AI’s liability risks, the costs involved with regulatory compliance, and the desire to encourage AI innovation.
Truby and his coauthors propose a more unified and robust sandbox framework than some existing proposals, such as the recent European Union proposal. The sandbox proposed by Truby and his coauthors would allow AI developers to use the sandbox for discovery, application, or regulatory compliance. These uses would then shift the question of AI liability to a time after successful testing in the sandbox environment.
Truby and his coauthors acknowledge the importance of regulating AI, but they note that placing too heavy a burden on certain AI developers, such as those developing difficult-to-test black box AIs, through a strict liability regime—where the developers are liable for any harm that their AI causes, even when acting with due diligence—will essentially operate as an outright ban on development.
Truby and his coauthors point out that their sandbox approach would avoid stifling innovation in these unique high-risk scenarios.
The Truby team explains that their sandbox approach, which allows for a beta test supervised by regulatory authorities, offers several advantages to developers. One advantage, direct communication between developers and regulators, helps both parties achieve more ideal outcomes by speeding up testing trials and teaching regulators more about the product or service.
One example that Truby and his coauthors use to support their argument is the United Kingdom Information Commissioner’s Office’s (ICO) regulatory sandbox. The ICO has a specific focus on innovations related to data sharing and offers its sandbox to help support this focus. Many of its past participants have reported high satisfaction with the benefits of the ICO sandbox and noted the many benefits of their participation.
Truby and his coauthors propose their more robust sandbox—one that allows developers to validate and test their AI without liability—as a supplement to current EU strict liability proposals. Using sandboxes such as the ICO’s as examples, Truby and his coauthors argue that their proposal could find a better balance between liability, compliance costs, and innovation. Specifically, they provide four reasons for pairing their sandbox with the current EU proposal.
First, they say that their sandbox would avoid restricting experimentation in high-risk areas of AI. Unlike the EU model, which places the burden of avoiding harm directly on the developers without offering a suitable method of testing their AI, the Truby model would follow the ICO’s example by allowing developers to work out potential issues in their AI alongside regulators. Developers, then, will be more likely to innovate and develop higher-risk AI that may provide greater benefits.
Second, Truby and his coauthors argue that, even as high-risk AI is refined at a greater rate because of the sandbox, its more robust boundaries would maintain a safeguarded and controlled regulatory environment. This risk management is a primary reason for a sandbox regulatory regime, the Truby team maintains.
Truby and his coauthors consider a third benefit of sandboxes—that sandboxes allow for the creation of a regulatory environment that is flexible enough to accommodate changes to the market without losing regulatory certainty. Their sandbox solves this problem, Truby and his coauthors argue, by pairing the sandbox with a strict liability regime, the sandbox’s flexibility reduces the burden of the strict liability regime, and the strict liability regime offers regulatory certainty that stabilizes the market.
Truby and his coauthors conclude by reaffirming the benefits that their sandbox approach offers when paired with current proposals for a strict liability regime. They do concede that their sandbox approach is based on heavily studied examples in the FinTech sector and may need more research before it can be successfully applied more broadly in the AI field. Despite this, the Truby team emphasizes that the sandbox regulation regime can add value by helping to balance safety-ensuring regulations and innovation-chilling regulations.