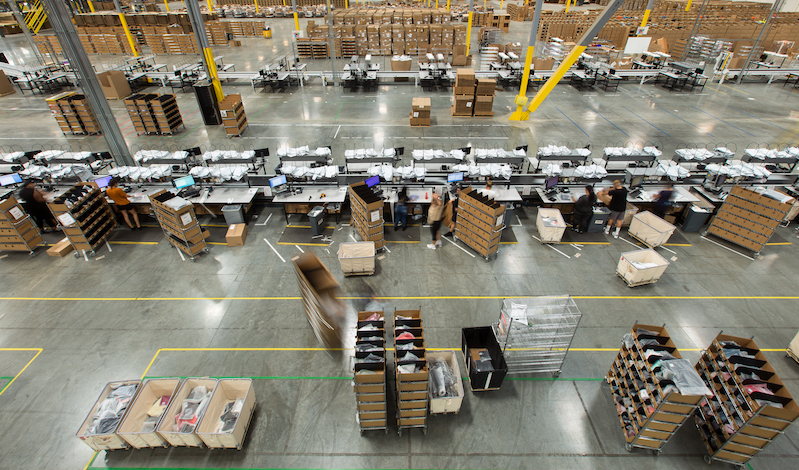
Advances in digital technology can help in managing the goals of federal acquisition.
The U.S. government procurement system aspires to obtain the best goods and services, from the best private sector firms, at the best prices. To attain these goals and ensure that taxpayer dollars are appropriately safeguarded, the Federal Acquisition Regulation (FAR) makes clear that the government procurement process demands the highest commitment to ethical conduct. To meet this commitment, the FAR incorporates a variety of tools designed to prevent, detect, and mitigate corruption risks in the U.S. government procurement system.
One way in which the FAR attempts to reduce risk in government procurement is by establishing processes to ensure that contracts are awarded to “responsible” contractors only. To determine whether a contractor is responsible, the FAR provides a list of standards contractors must meet, including adequate financial resources, a satisfactory past performance record, a demonstrated record of integrity and business ethics, and necessary experience. The contractor must also be “otherwise qualified and eligible to receive an award under applicable laws and regulations.”
To verify whether a prospective contractor meets these standards, contractors are required to provide numerous representations and certifications about their ability to perform the contract and comply with the many laws and requirements imposed on companies that do business with the U.S. government.
Acquisition professionals charged with making these “responsibility determinations” must review information from a variety of databases that collect not only the representations and certifications but also other relevant information, including past performance history, civil and criminal settlements, exclusions (such as suspensions or debarments), and contract terminations. Reviewing and documenting this information is a notoriously lengthy and cumbersome process.
In recent years, several U.S. government agencies have pioneered the use of artificial intelligence (AI) and other emerging technologies to improve the efficiency and accuracy of these procurement determinations.
For example, the U.S. Army has developed and deployed a Determination of Responsibility Assistant bot (DORA), which has revolutionized the responsibility determination process. DORA can pull relevant information about a vendor from government databases and websites, such as the System for Award Management and the Federal Awardee Performance and Integrity Information System and then create a report for the relevant contracting specialist in under five minutes. When completed manually, this process usually takes up to an hour.
At the U.S. Department of Homeland Security (DHS), acquisition officials turned to AI to help improve the agency’s review of contractor past performance records. Like other federal agencies, acquisition professionals at DHS struggled with a multitude of issues relating to its review of information found in the Contractor Performance Assessment Reporting System (CPARS), including poor data quality and acquisition teams not using the system. As the agency has explained:
CPARS has a wealth of data, with over 1 million records for over 60,000 vendors, but it is hard to rapidly access assessment reports that are relevant to a given source selection. One company might have contracts to support the Department of Defense for weapon systems, DHS for cybersecurity, and Veterans Affairs for information systems, with multiple years of performance. In this scenario, the report results are voluminous. There is no efficient way for a human to easily review them all. It is difficult to manually review each record and synthesize the information to get an overall picture of the contractor’s past performance.
Given these challenges, acquisition professionals often turned to workarounds, such as distributing past performance questionnaires to contractors, which relinquished “the responsibility of identifying relevant past performance information to vendors.”
In response, DHS developed the Artificial Intelligence for Past Performance Project, through which it has experimented with emerging technology, such as machine learning and AI, to improve the past performance review process by enabling acquisition professionals to “quickly identify the CPARS assessment records that are most relevant to a current past performance source selection evaluation based on a given solicitation.”
There are other examples of similar innovations occurring across the federal government. And although these developments should be rightfully applauded, reacting to them with a sense of guarded optimism is in order. These exciting innovations show promise in improving the contractor vetting process, yet some key concerns remain.
The technology, for example, is only as good as the underlying data it uses. If data collection is poor, inaccurate, or incomplete, then subsequent analyses will also be poor, inaccurate, or incomplete. For instance, both the Army and DHS rely on information provided in government databases to assist in their evaluation of contractors. Yet, anyone who has spent 10 minutes on these sites is aware of their deficiencies. Not only does the accuracy of the information they house depend on civil servants actually inputting the data, but it also depends on them inputting it correctly.
There are also legitimate concerns about the government relying on this information to make critical decisions without improvements to the quality of information collected, maintained, and disclosed in these systems. As agencies continue to innovate, they must dedicate attention and resources to ensuring data is accurate. Moreover, they should consider additional layers of review to safeguard against improper determinations based upon inaccurate or incomplete data.
Government procurement and good governance experts are rightfully enthusiastic about the use of AI and other emerging technologies to drive greater efficiency and help mitigate the risks of fraud, corruption, and incompetence in the U.S. procurement system. As federal agencies continue to think strategically about how to improve processes and reduce risk in their procurements, technology-driven solutions will play a critical role in this undertaking.
This essay is part of a nine-part series entitled Artificial Intelligence and Procurement.