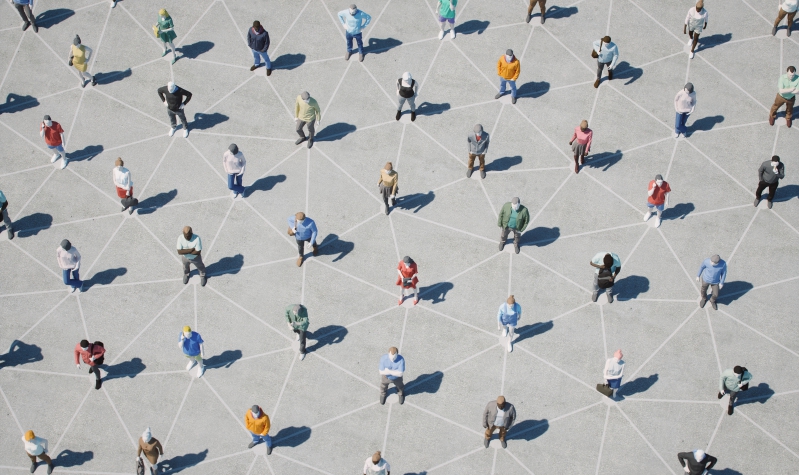
Data sharing is not always required when firms use information to their advantage.
The European Union is positioning data sharing as a central piece of its online economic strategy. For decades, European courts have imposed mandatory data sharing as antitrust remedies. The Magill decision in 1995, IMS in 2004, and Microsoft in 2007, among others, established precedent for data sharing remedies.
The data shared in these cases often did not include user data but instead data on dominant companies’ processes, structures, and internal documents. These remedies have often proved effective, but the European approach to data sharing is changing. As the EU finalizes a new regulation, this time data sharing could cover users’ data, including their interactions with online products and services.
In recent weeks, the European Parliament and EU member states reached an agreement on the European Data Governance Act. It followed the proposal made by the European Commission to mandate different types of data to be shared, including personal data. And in the coming days, the European Commission will introduce the Data Act with rules to provide better control for data sharing to citizens and businesses.
Looking at these initiatives from a competition angle, they seek to answer “concerns about data accumulation.” The underlining logic stems from the emerging idea that data have become “the new oil.” If the analogy were correct, various companies would easily use the same data and data sharing would indeed be effective. But the analogy is misleading.
First, contrary to oil, data are non-fungible; each dataset is unique.
Second, data are an “experience good,” meaning that companies generate value by combining data with other data and internal processes. It has little value in itself, unlike oil, which has a known market value before acquiring it.
Finally, oil has relatively constant returns to scale: An increase in oil results in a proportional increase in output. Data have rapidly diminishing returns, and ultimately no or negative returns when the costs of managing the data exceed the benefits.
So, more data are not always better for companies. But new data can provide a benefit if they differ from existing datasets. Accordingly, processes to deal with data prove at least equally important as the quantity of data. Google’s failure to engage users to stay on Google+ for over five seconds is a good example of the limits of big data and “bigness” analyses.
To be sure, data sharing can positively affect competition as companies may need more data to improve their products and benefit from “learning effects”—the more data about user behavior, the better a product or service becomes. For instance, access to the data generated by users’ interaction with online translation services, such as the frequency at which users run a new search or are editing the results after the first result displays, can prove valuable to competitors. But this example shows one of the greatest limits of data sharing: A dataset is only useful if the company holding it can use it.
In practice, however, companies rarely need the same data. Social media serves as a good example. Facebook mainly relies on “likes” to build user profiles. TikTok measures how long users spend on each video, how many accounts users follow, and various video information, such as captions, sounds, and hashtags. Instagram focuses on interactions with pictures and reels. YouTube emphasizes user profiles that watch similar videos and users’ Google Account activity. And Snapchat zeroes in on engagement with stories and filters.
Although these descriptions are over-simplified, as these companies measure users’ engagement in many ways, these distinctions demonstrate how difficult it would be for one of these social media companies to use other companies’ data.
Because each company provides users with a unique experience, data are often too specific to each company’s business for other companies to use. So, data sharing alone will not foster online competition.
I propose an exploration of new processes to use data. In recent months, computer science literature has documented promising methods to develop artificial intelligence (AI) systems that require less data and could benefit small players. One example is “less than one-shot” algorithms, which offer a new way to train a machine learning model.
For example, a machine can be trained to distinguish between two classes—say, cats and dogs—and later add tigers to the list of classes it can detect without providing tiger images. Researchers have already used this method with hierarchical soft-label classification algorithms.
Data distillation—the process that reduces large datasets to small ones—has sparked new data processing techniques allowing computers to extract several characteristics from a single data point. Also, new methods to improve accuracy exist that train language models to compare in real time what the machine is writing with existing databases, such as Wikipedia and other websites. In recent experiments, these models matched neural networks 25 times their size.
If these techniques develop and democratize, small and medium companies could benefit from increased learning effects and better use the data they have to compete with companies holding larger datasets. Translation service users might agree that DeepL provides better results than Google translation—sorry, Google—despite having fewer users and less data. In such cases, new ways of processing data could improve competition better than imposing data sharing.
Public institutions have an important role in fostering the emergence of these new data techniques by investing in research and development programs, awarding grants and rewards, fostering patent buyouts, and adopting other methods of public investment.
In Europe, the European Research Council and other individual grants serve as potential outlets for support, but no coordinated strategy in this space exists. The Data Governance Strategy does not mention or support the need for innovative processes working with smaller datasets.
Although mandating data sharing does not stop innovation in data processing, regulators should set priorities where the potential is the greatest. Here, fostering advances in computer science—and, specifically, data processing techniques—should be antitrust policymakers’ and regulators’ top priority. At the very least, regulators should acknowledge and support them.
This regulatory stance would require shaping legal and economic incentives appropriately. As researchers volunteer their weekends to build an open source language model, public institutions should support them financially and expand financing to other research projects exploring new processes instead of focusing on data quantities.