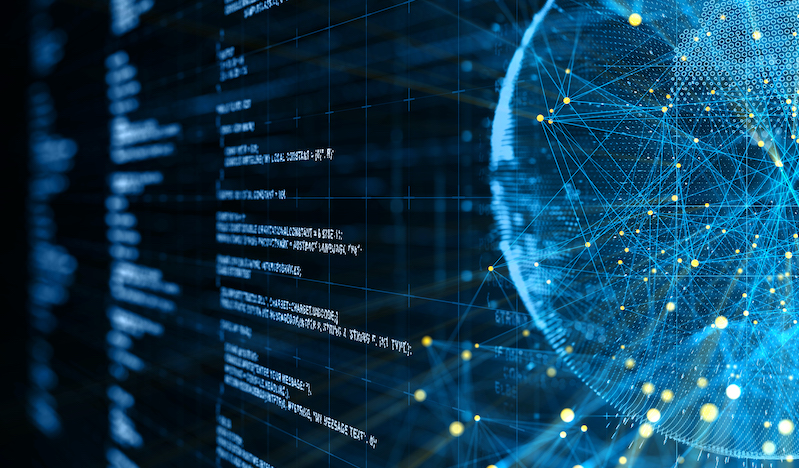
Developing ongoing programs to monitor AI and sustain good outcomes is essential to ensure AI accountability.
Private companies, governments, and organizations around the world seek to build AI systems that are safe when employed, comply with applicable law, minimize harm and risk to affected stakeholders, and are—in a word—responsible in their behavior.
But building responsible AI systems remains an elusive goal: AI systems have exhibited unexpected failure and fragility, reflected structural bias in unintended ways, and reinforced existing power asymmetries.
Policy interventions around the world have sought to provide frameworks for evaluating risks and harms caused by AI systems, so that problems can be identified and mitigated when systems are designed or procured.
These frameworks, however, generally treat both harms and mitigation instrumentally, assuming that problems with AI can be fixed with technical interventions such as improvements to data. This approach is fundamentally flawed: sociotechnical systems require sociotechnical control.
The most extensively studied assemblages of humans and technical artifacts with high levels of trust are sociotechnical systems. These systems are complex agglomerations of people, organizations, policies, and technical components where behavior is defined by the hierarchy and structure of interactions, new properties that emerge from interacting components, and communication of control information among the disparate parts.
Examples of high trust sociotechnical systems include the structural engineering and construction of large structures such as bridges, skyscrapers, and other infrastructure; safety in aviation, medicine, and industrial processes; and clinical outcomes in medicine. In each case, what assures that the technology serves the system’s goal is not the technical capabilities, a discrete set of control requirements, or a magic tool that system controllers can buy, but an entire management and oversight program.
A program is more than a process or a piece of technology. But the design and capabilities of technological components can support or enable programs. Consider how the presence of smoke detectors in a building can alert people to a fire, which could cause structural failure. But this alert is only useful in conjunction with plans for building evacuation and the presence of adequate fire response and suppression. Programs include resourcing for staffing, training, oversight, management, and ongoing maintenance.
Similarly, sensors available to airplane mechanics can determine when a plane’s engine has degraded performance or requires scheduled or conditions-based maintenance. But these tools are only useful when the sensor outputs are read by the maintenance team and appropriate responsive actions can be taken.
Assurance comes not from the technology, but from an assemblage of technology and humans, organizations, cultures, and policies. In other words, the control is itself a sociotechnical system.
It is not the safety features that make these systems safe, but the robust safety policies, cultures, and programs that drive operationalization of the activities necessary to avoid hazards and harms.
Frameworks such as the U.S. National Institute of Standards and Technology’s new draft AI Risk Management Framework, the World Economic Forum’s AI procurement guidelines, and the European Union’s AI regulatory framework proposal aim to bridge the gaps between policy goals and the details of technology development. These frameworks seek to improve management decision-making, reinforced by implementable requirements. Yet, these frameworks exemplify the problems of instrumental approaches to responsible AI development and procurement. By focusing attention during the development and procurement processes on specific risks and testable outcomes, the frameworks decontextualize AI systems and their control.
A framework might, for example, demand testing for bias in system outputs or call for modifying the system to “de-bias” these outputs, but these activities deemphasize the question of why the bias is present in the first place or how its presence in outputs affects actual outcomes.
Concretely, an AI resume sorting system might rank the resumes of women lower than those of men not because the women are less qualified but because fewer women are promoted after hiring and the system is designed to rank on likelihood of retention and promotion based on historical data. The solution to this underlying issue is not to boost the ranking of women’s resumes at hiring or even to hire more women, but to understand why more women are not being promoted and to create the conditions for women to be successful in that organization. That would be true even without the AI system.
Procurement and computing system development are alike in that both often start with a set of explicit requirements about what the procured artifact or final delivered system must do to be considered adequate. But properties such as safety, legal compliance, or trustworthiness are not well circumscribed by an obvious set of requirements. Instead, these are what engineers call “non-functional” properties. Rather than being explicit “functions” of a system, they are assessments of the system’s performance in general, not subject to a specific test.
Consider the question of whether a procured software program has an exploitable vulnerability. Demonstrating how to exploit the software is proof positive that a vulnerability exists. There is, however, no technical way to demonstrate that vulnerabilities do not exist. The non-existence of vulnerabilities must instead be argued based on the software’s design and implementation. Most software development organizations have a management program in place to operationalize the security of code produced and to manage the identification and remediation of vulnerabilities discovered over a product’s lifecycle
Framing the problem of responsible AI in terms of requirements and individuated controls leads to siloed thinking: Seeking to identify problems leads to adding new requirements that introduce specific compensating controls.
Complexity proliferates while key questions go uninvestigated, such as whether slightly altered goals or methods could avoid risks altogether. That is not to say that requirements cannot capture important system features, but that alone they disaggregate risks and create the illusion of control.
Responsible AI frameworks focus attention on developing technical “fixes” for technical problems when they treat solutions as driven by individual controls rather than a systemic match between AI system operation and AI system sociotechnical control.
Procuring responsible AI is not a question of sourcing from responsible vendors or adding requirements for testing and evaluation onto the functional requirements. Rather, responsible AI is a problem of developing an adequate management program.
Such programs might be aided by technical requirements for traceability, ongoing data management to monitor potential operational performance drift, and training for human users of the AI system. Training could even focus on managing cultures around the system so that humans who interact with it understand when to rely on AI and when to override it. But management programs have their own requirements for staffing, training, and ongoing operations. Requirements attach not only to procured artifacts, but also to the organizations in which those artifacts will be used.
Humans are also often needed to adapt technologies into existing organizational processes by “repairing” the technologies and the processes until they match or making adjustments when they drift apart.
For example, systems for ranking web pages, products, social media posts, and other content—and systems that match advertisements to viewers—are exquisitely instrumented to capture the ways that changes to content, end user behavior, or the relationship between them might affect the value of the ranking or ad assignment. Companies that supervise such systems have entire teams devoted to monitoring these analytics and validating them, possibly by collecting and annotating new data against which existing system behavior can be validated.
Responsible AI is not merely responsibly sourced AI or AI contracted to follow an organization’s internal controls. It requires an ongoing effort to understand an AI system’s behaviors and sustain good outcomes. AI defines and operationalizes organizational policies; it is not a re-deployable tool like a telephone system or human resources database.
Programs must be built, not bought. Costs for AI must include activities beyond development, acquisition, and initial integration. They should account for ongoing monitoring and sustainment, maintenance, and upgrading throughout the AI lifecycle.
Recognizing that responsible AI is an ongoing process more than a product does not mean that organizations cannot responsibly buy AI systems. Rather, it means that when they do, they must take care when adopting these new tools that the tools capture the organization’s intended outcomes and avoid undesirable ones.
This essay is part of a nine-part series entitled Artificial Intelligence and Procurement.