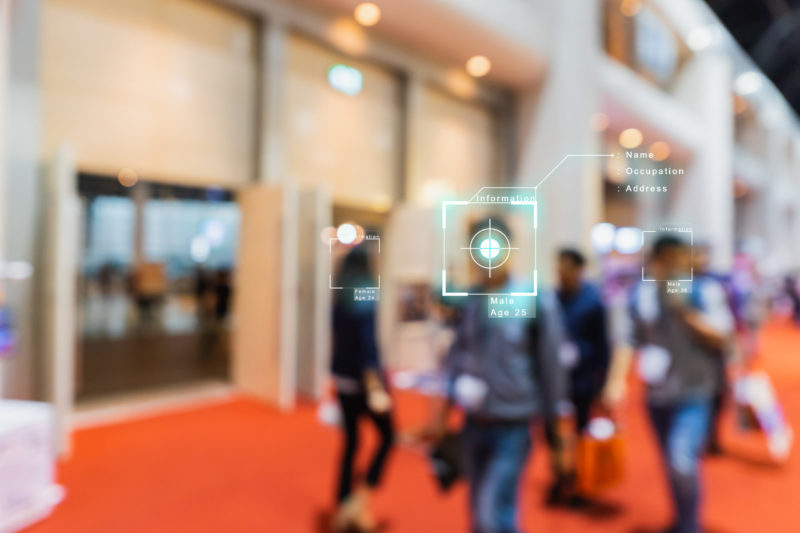
Experts advocate robust regulation of facial recognition technology to reduce discriminatory outcomes.
After Detroit police arrested Robert Williams for another person’s crime, officers reportedly showed him the surveillance video image of another Black man that they had used to identify Williams. The image prompted him to ask the officers if they thought “all Black men look alike.” Police falsely arrested Williams after facial recognition technology matched him to the image of a suspect—an image that Williams maintains did not look like him.
Some experts see the potential of artificial intelligence to bypass human error and biases. But algorithms used in artificial intelligence are only as good as the data used to create them—data that often reflect racial, gender, and other human biases.
In a National Institute of Standards and Technology report, researchers studied 189 facial recognition algorithms—“a majority of the industry.” They found that most facial recognition algorithms exhibit bias. According to the researchers, facial recognition technologies falsely identified Black and Asian faces 10 to 100 times more often than they did white faces. The technologies also falsely identified women more than they did men—making Black women particularly vulnerable to algorithmic bias. Algorithms using U.S. law enforcement images falsely identified Native Americans more often than people from other demographics.
These algorithmic biases have major real-life implications. Several levels of law enforcement and U.S. Customs and Border Protection use facial recognition technology to support policing and airport screenings, respectively. This technology sometimes determines who receives housing or employment offers. One analyst at the American Civil Liberties Union reportedly warned that false matches “can lead to missed flights, lengthy interrogations, watch list placements, tense police encounters, false arrests, or worse.” Even if developers can make the algorithms equitable, some advocates fear that law enforcement will employ the technology in a discriminatory manner, disproportionately harming marginalized populations.
A few U.S. cities have already banned law enforcement and other government entities from using facial recognition technology. But only three states have passed privacy laws pertaining to facial recognition technology. Currently, no federal law governs the use of facial recognition technology. In 2019, members of the U.S. Congress introduced the Algorithmic Accountability Act. If passed, it would direct the Federal Trade Commission (FTC) to regulate the industry and require companies to assess their technology continually for fairness, bias, and privacy issues. As of now, the FTC only regulates facial recognition companies under general consumer protection laws and has issued recommendations for industry self-regulation.
Given its potential for harm, some experts are calling for a moratorium on facial recognition technology until strict regulations are passed. Others advocate an outright ban of the technology.
This week’s Saturday Seminar addresses fairness and privacy concerns associated with facial recognition technology.
- “There is historical precedent for technology being used to survey the movements of the Black population,” writes Mutale Nkonde, founder of AI for the People. In an article in the Harvard Kennedy School Journal of African American Policy, she draws a through line from past injustices to discriminatory technology today. She explains that facial recognition technology relies on the data developers feed it—developers who are disproportionately white. Nkonde urges lawmakers to adopt a “design justice framework” for regulating facial recognition technology. Such a framework would center “impacted groups in the design process” and reduce the error rate that leads to anti-Black outcomes.
- The use of facial recognition technology is growing more sophisticated, but it is far from perfect. In a Brookings Institution article, Daniel E. Ho of Stanford Law School and his coauthors urge policymakers to address issues of privacy and racial bias related to facial recognition. Ho and his coauthors recommend that regulators develop a framework to ensure adequate testing and responsible use of facial recognition technology. To ensure more accurate results, they call for more robust validation tests that take place in real-world settings instead of the current validation tests, which take place in controlled settings.
- Facial recognition technology poses serious threats to some fundamental human rights, Irena Nesterova of the University of Latvia, Faculty of Law claims in an SHS Web of Conferences article. Nesterova argues that facial recognition technology can undermine the right to privacy, which would impact citizens’ sense of autonomy in society and harm democracy. Pointing to the European Union’s General Data Protection Regulation as a model, Nesterova proposes several ways in which facial recognition could be regulated to mitigate the harmful effects that the increasingly prevalent technology might have on democracy. These methods include setting strict limits on when and how public and private entities can use the technology and requiring companies to perform accuracy and bias testing on their technology.
- Elizabeth A. Rowe of the University of Florida Levin College of Law proposes in a Stanford Technology Law Review article three steps that the U.S. Congress should consider while debating whether to regulate facial recognition technology. First, Rowe urges lawmakers to consider discrete issues within facial recognition technology separately. For instance, members of Congress should address concerns about biases in algorithms differently than they address privacy concerns about mass surveillance. Second, Rowe contends that regulations should provide specific rules concerning the “storage, use, collection, and sharing” of facial recognition technology data. Finally, Rowe suggests that a trade secrecy framework could prevent the government or private companies from misappropriating individuals’ information gathered through facial recognition technology.
- In an article in the Boston University Journal of Science and Technology Law, Lindsey Barrett of Georgetown University Law Center advocates banning facial recognition technology. Barrett claims that the use of facial recognition technology violates individuals’ rights to “privacy, free expression, and due process.” Facial recognition technology has a particularly high potential to cause harm, Barrett suggests, when it targets children because facial recognition technology is less accurate at identifying children. Barrett argues that current laws inadequately protect children and the general population. She concludes that to protect children and other vulnerable populations, facial recognition technology must be banned altogether.
- In a Loyola Law Review article, Evan Selinger of Rochester Institute of Technology and Woodrow Hartzog of Northeastern University School of Law assert that many proposed frameworks for regulating facial recognition technology rely on a consent requirement. But they argue that individuals’ consent to surveillance by this technology is rarely meaningful given the lack of alternatives to participating in today’s technological society. For example, without even reading the terms and conditions, internet users can grant technology companies use of their images, Selinger and Hartzog explain. Although lawmakers could regulate the technology and require consent, any use of the technology will inevitably reduce society’s “collective autonomy,” they argue. Selinger and Hartzog conclude that the only way to prevent the harms of facial recognition technology is to ban it.
The Saturday Seminar is a weekly feature that aims to put into written form the kind of content that would be conveyed in a live seminar involving regulatory experts. Each week, The Regulatory Review publishes a brief overview of a selected regulatory topic and then distills recent research and scholarly writing on that topic.